On The Ingredients of an Effective Zero-shot Semantic Parser
PROCEEDINGS OF THE 60TH ANNUAL MEETING OF THE ASSOCIATION FOR COMPUTATIONAL LINGUISTICS (ACL 2022), VOL 1: (LONG PAPERS)(2022)
摘要
Semantic parsers map natural language utterances into meaning representations (e.g. programs). Such models are typically bottlenecked by the paucity of training data due to the laborious annotation efforts. Recent studies have performed zero-shot learning by synthesizing training examples of canonical utterances and programs from a grammar, and further paraphrasing these utterances to improve linguistic diversity. However, such synthetic examples cannot fully capture patterns in real data. In this paper we analyze zero-shot parsers through the lenses of the language and logical gaps (Herzig and Berant, 2019), which quantify the discrepancy of language and programmatic patterns between the synthetic canonical examples and real-world user-issued ones. We propose bridging these gaps using improved grammars, stronger paraphrasers, and efficient learning methods using canonical examples that most likely reflect real user intents. Our model achieves strong results on the SCHOLAR and GEO benchmarks with zero labeled data.(1)
更多查看译文
关键词
zero-shot
AI 理解论文
溯源树
样例
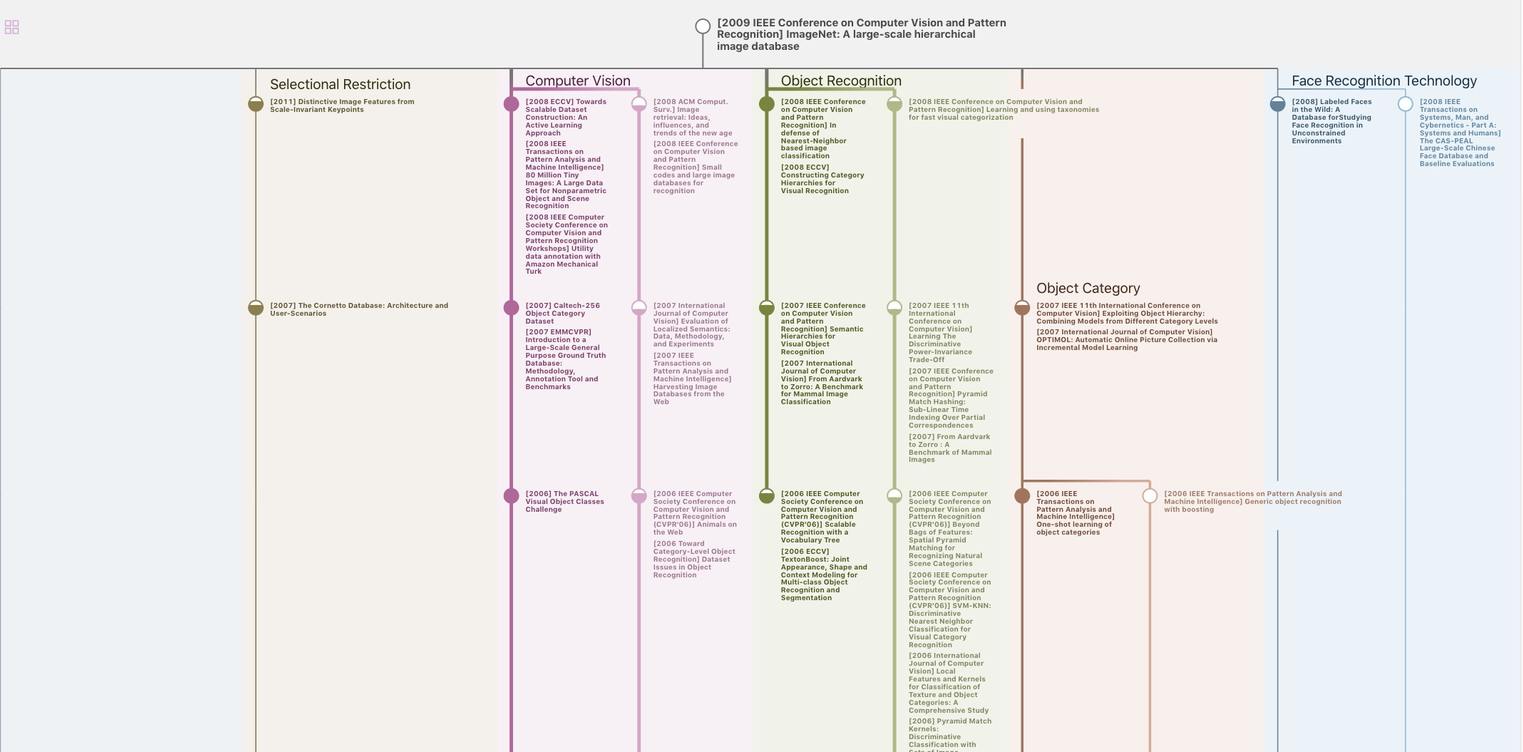
生成溯源树,研究论文发展脉络
Chat Paper
正在生成论文摘要