On the Necessity of Auditable Algorithmic Definitions for Machine Unlearning
PROCEEDINGS OF THE 31ST USENIX SECURITY SYMPOSIUM(2022)
摘要
Machine unlearning, i.e. having a model forget about some of its training data, has become increasingly more important as privacy legislation promotes variants of the right-to-be-forgotten. In the context of deep learning, approaches for machine unlearning are broadly categorized into two classes: exact unlearning methods, where an entity has formally removed the data point's impact on the model by retraining the model from scratch, and approximate unlearning, where an entity approximates the model parameters one would obtain by exact unlearning to save on compute costs. In this paper, we first show that the definition that underlies approximate unlearning, which seeks to prove the approximately unlearned model is close to an exactly retrained model, is incorrect because one can obtain the same model using different datasets. Thus one could unlearn without modifying the model at all. We then turn to exact unlearning approaches and ask how to verify their claims of unlearning. Our results show that even for a given training trajectory one cannot formally prove the absence of certain data points used during training We thus conclude that unlearning is only well-defined at the algorithmic level, where an entity's only possible auditable claim to unlearning is that they used a particular algorithm designed to allow for external scrutiny during an audit.
更多查看译文
关键词
auditable algorithmic definitions,machine unlearning
AI 理解论文
溯源树
样例
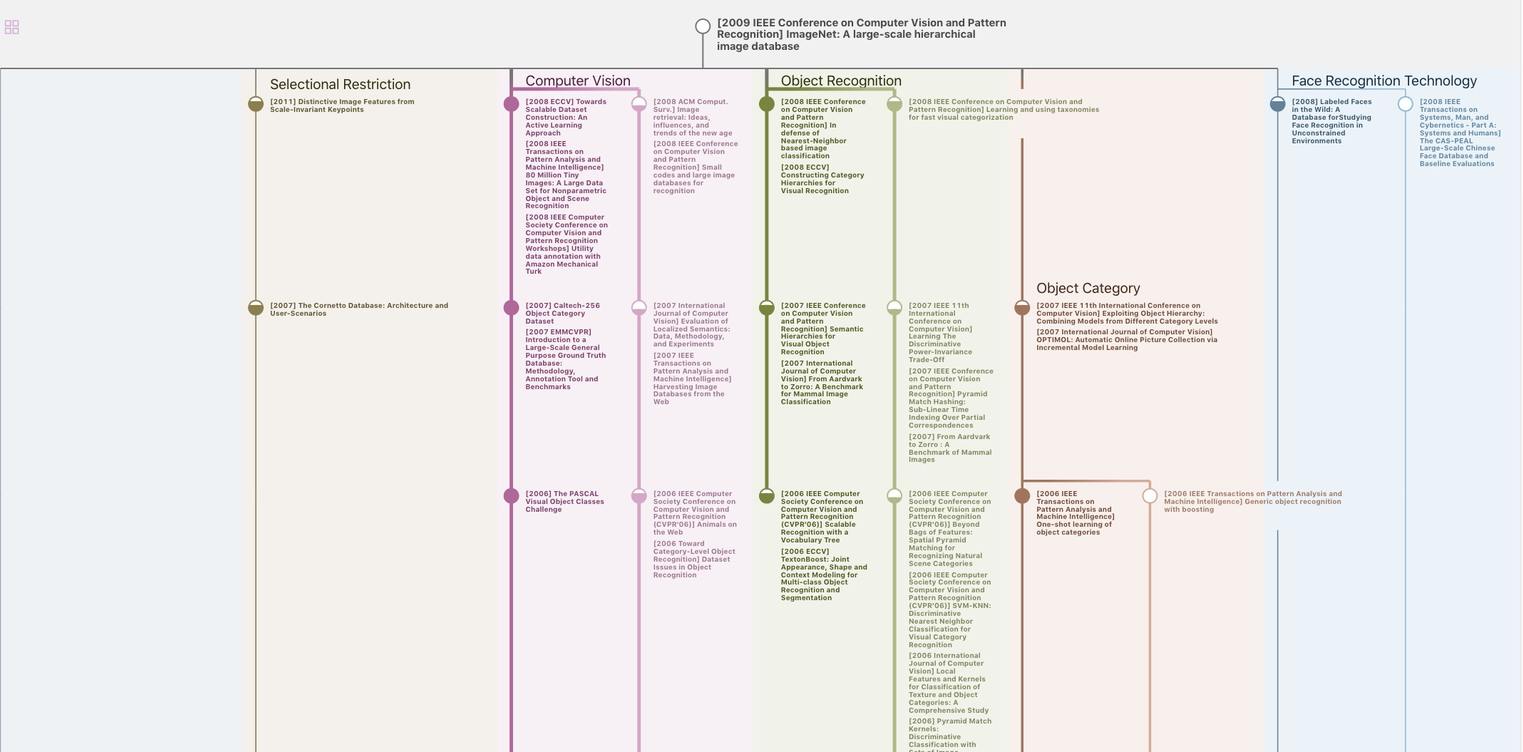
生成溯源树,研究论文发展脉络
Chat Paper
正在生成论文摘要