VelocityNet: Motion-Driven Feature Aggregation for 3D Object Detection in Point Cloud Sequences
2021 IEEE INTERNATIONAL CONFERENCE ON ROBOTICS AND AUTOMATION (ICRA 2021)(2021)
摘要
The most successful methods for LiDAR-based 3D object detection use sequences of point clouds in order to exploit the increased data density through temporal aggregation. However, common aggregation methods are rarely able to capture fast-moving objects appropriately. These objects are displaced by large distances between frames and naive approaches are not able to successfully leverage the full amount of information spread across time. Yet, especially in autonomous driving scenarios, fast-moving objects are most crucial to detect as they actively take part in highly dynamic traffic situations. This work presents a novel network architecture called VelocityNet which is explicitly designed to temporally align features according to object motion. Our approach extends traditional 3D convolutions by a motion-driven deformation of the convolution kernels across the temporal dimension. The required motion information can be obtained from various sources, ranging from external computation or complementary sensors to an integrated network branch which is trained jointly with the object detection task. The explicit feature alignment allows the training process to focus on the object detection problem and results in a significant increase in detection performance compared to the popular PointPillars baseline, not only for dynamic but also for static objects. We evaluate our approach on the nuScenes dataset and analyze the main reasons for the observed performance gains.
更多查看译文
关键词
VelocityNet,motion-driven feature aggregation,point cloud sequences,LiDAR-based 3D object detection,increased data density,temporal aggregation,common aggregation methods,autonomous driving scenarios,highly dynamic traffic situations,novel network architecture,traditional 3D convolutions,motion-driven deformation,convolution kernels,temporal dimension,required motion information,integrated network branch,object detection task,explicit feature alignment,object detection problem,detection performance,static objects
AI 理解论文
溯源树
样例
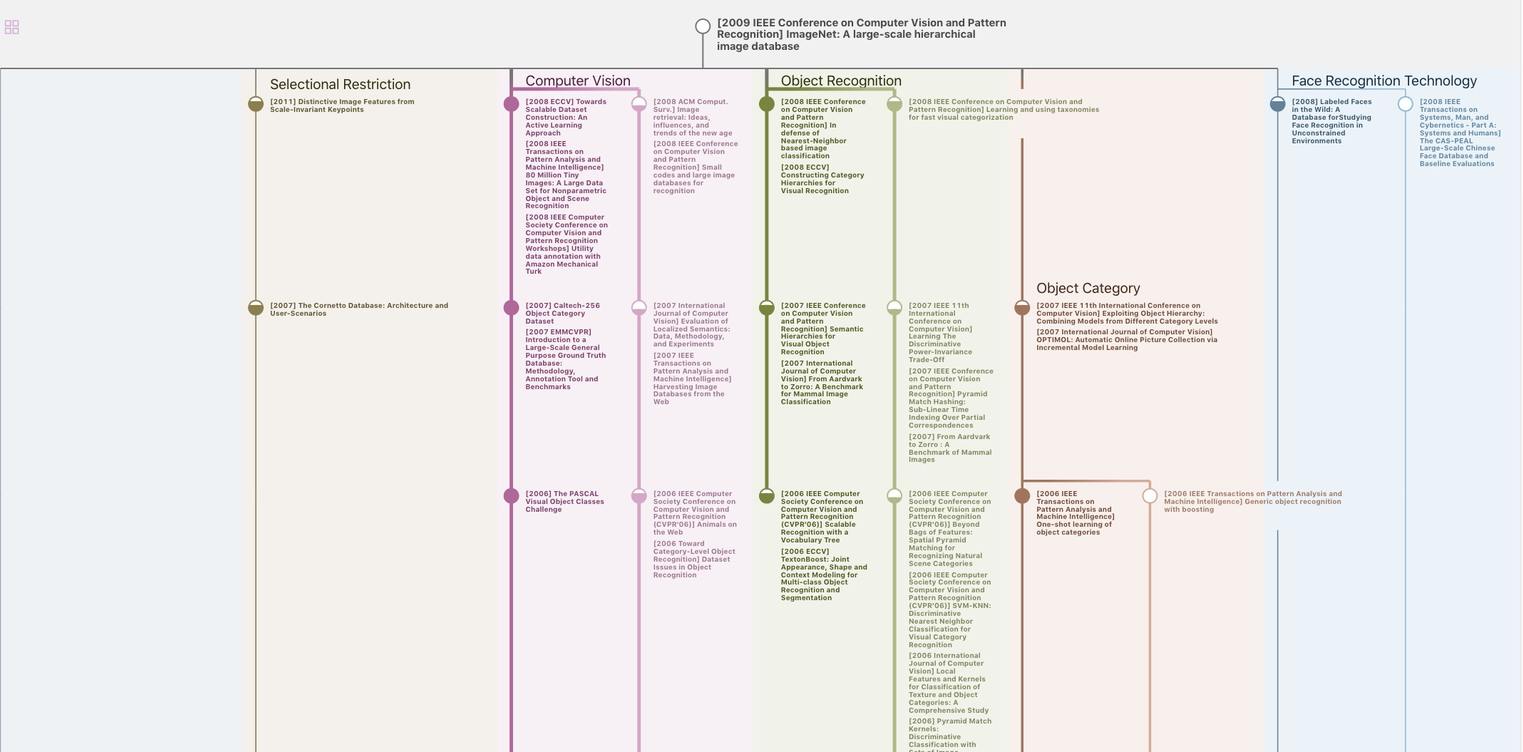
生成溯源树,研究论文发展脉络
Chat Paper
正在生成论文摘要