SLIM - Self-Supervised LiDAR Scene Flow and Motion Segmentation.
ICCV(2021)
摘要
Recently, several frameworks for self-supervised learning of 3D scene flow on point clouds have emerged. Scene flow inherently separates every scene into multiple moving agents and a large class of points following a single rigid sensor motion. However, existing methods do not leverage this property of the data in their self-supervised training routines which could improve and stabilize flow predictions. Based on the discrepancy between a robust rigid ego-motion estimate and a raw flow prediction, we generate a self-supervised motion segmentation signal. The predicted motion segmentation, in turn, is used by our algorithm to attend to stationary points for aggregation of motion information in static parts of the scene. We learn our model end-to-end by backpropagating gradients through Kabsch's algorithm and demonstrate that this leads to accurate ego-motion which in turn improves the scene flow estimate. Using our method, we show state-of-the-art results across multiple scene flow metrics for different real-world datasets, showcasing the robustness and generalizability of this approach. We further analyze the performance gain when performing joint motion segmentation and scene flow in an ablation study. We also present a novel network architecture for 3D LiDAR scene flow which is capable of handling an order of magnitude more points during training than previously possible.
更多查看译文
关键词
Motion and tracking,Scene analysis and understanding,Segmentation,grouping and shape,Stereo,3D from multiview and other sensors,Transfer/Low-shot/Semi/Unsupervised Learning,Vision for robotics and autonomous vehicles
AI 理解论文
溯源树
样例
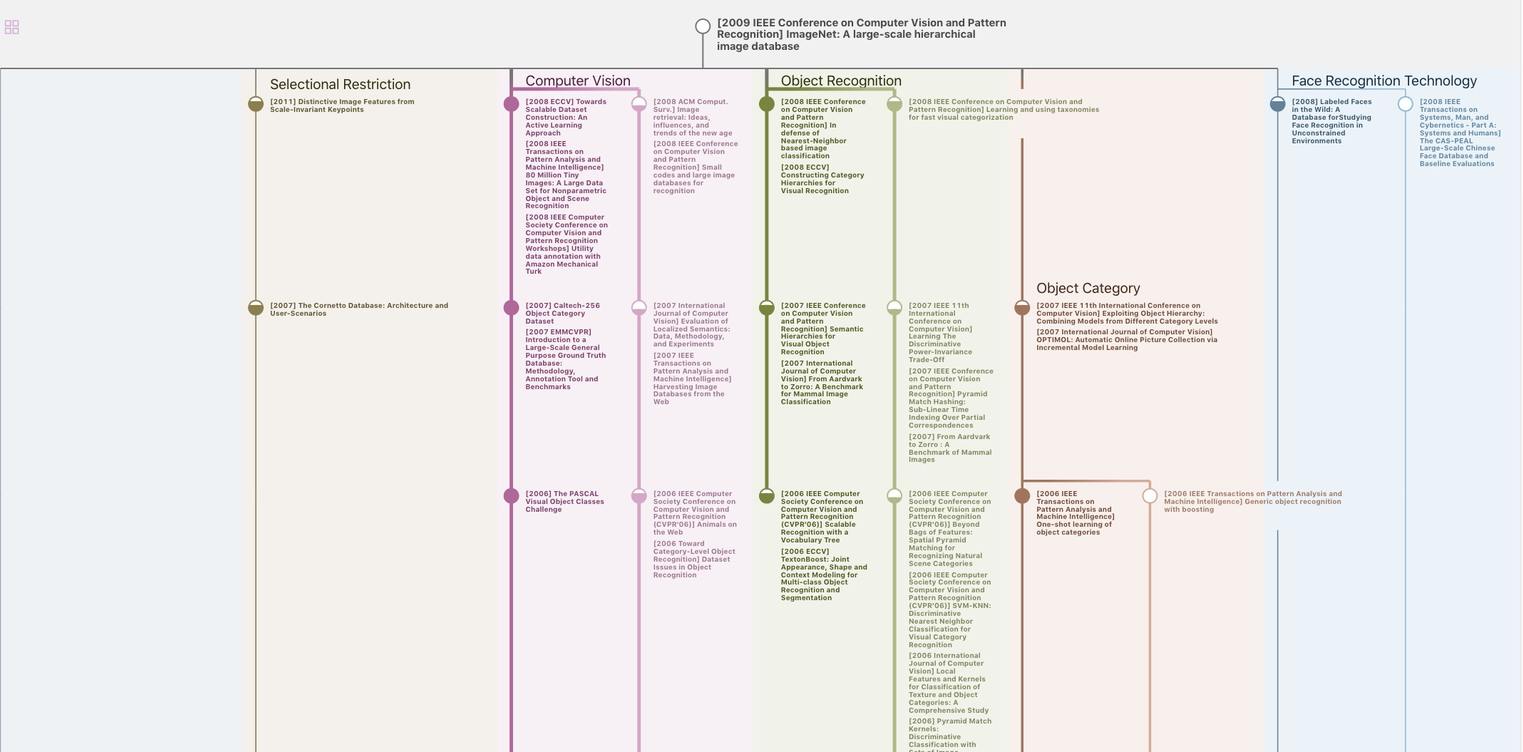
生成溯源树,研究论文发展脉络
Chat Paper
正在生成论文摘要