DUBStepR: correlation-based feature selection for clustering single-cell RNA sequencing data
biorxiv(2021)
摘要
Feature selection (marker gene selection) is widely believed to improve clustering accuracy, and is thus a key component of single cell clustering pipelines. However, we found that the performance of existing feature selection methods was inconsistent across benchmark datasets, and occasionally even worse than without feature selection. Moreover, existing methods ignored information contained in gene-gene correlations. We therefore developed DUBStepR (Determining the Underlying Basis using Stepwise Regression), a feature selection algorithm that leverages gene-gene correlations with a novel measure of inhomogeneity in feature space, termed the Density Index (DI). Despite selecting a relatively small number of genes, DUBStepR substantially outperformed existing single-cell feature selection methods across diverse clustering benchmarks. In a published scRNA-seq dataset from sorted monocytes, DUBStepR sensitively detected a rare and previously invisible population of contaminating basophils. DUBStepR is scalable to over a million cells, and can be straightforwardly applied to other data types such as single-cell ATAC-seq. We propose DUBStepR as a general-purpose feature selection solution for accurately clustering single-cell data.
### Competing Interest Statement
The authors have declared no competing interest.
更多查看译文
关键词
feature selection,rna,correlation-based,single-cell
AI 理解论文
溯源树
样例
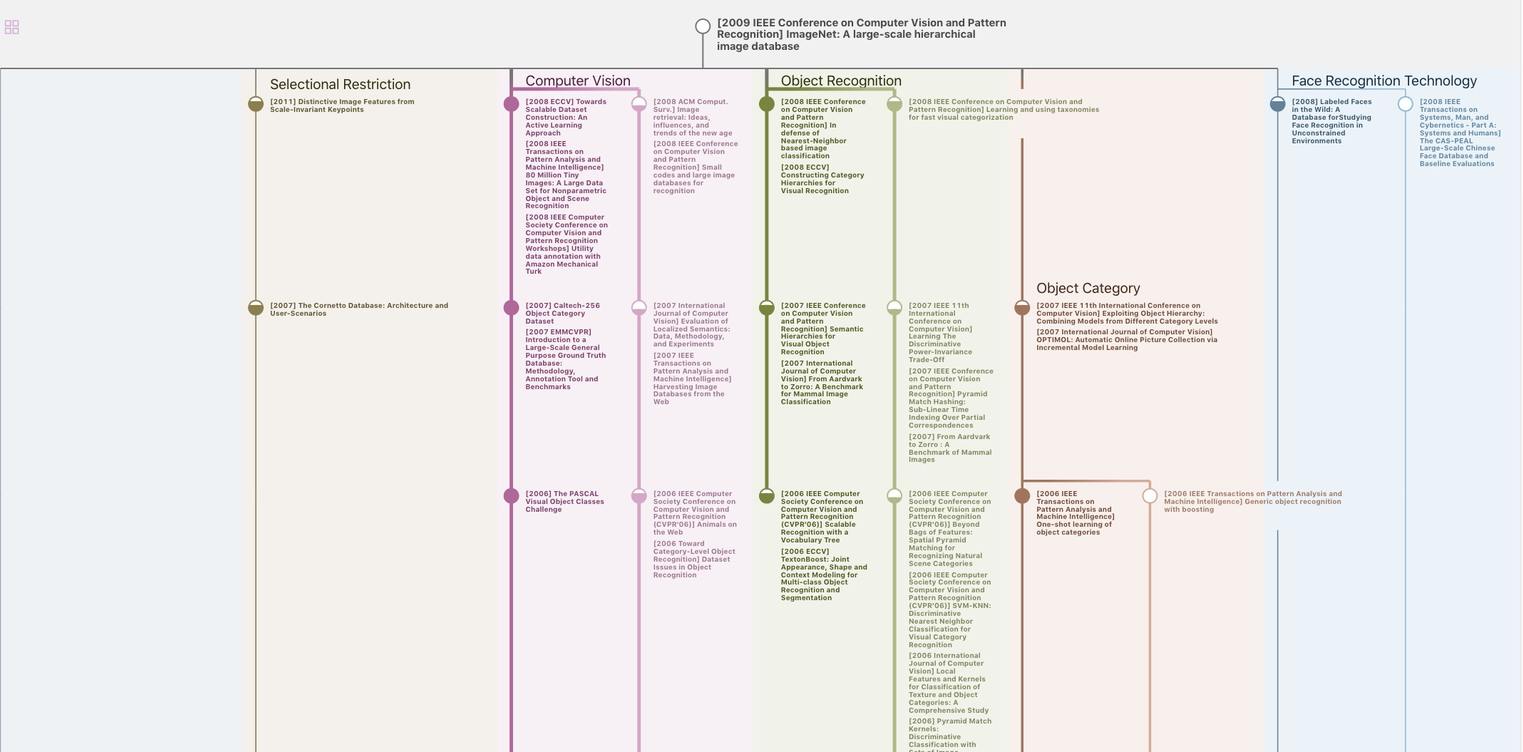
生成溯源树,研究论文发展脉络
Chat Paper
正在生成论文摘要