MolRep: A Deep Representation Learning Library for Molecular Property Prediction
biorxiv(2021)
摘要
Summary Recently, novel representation learning algorithms have shown potential for predicting molecular properties. However, unified frameworks have not yet emerged for fairly measuring algorithmic progress, and experimental procedures of different representation models often lack rigorousness and are hardly reproducible. Herein, we have developed MolRep by unifying 16 state-of-the-art models across 4 popular molecular representations for application and comparison. Furthermore, we ran more than 12.5 million experiments to optimize hyperparameters for each method on 12 common benchmark data sets. As a result, CMPNN achieves the best results ranked the 1st in 5 out of 12 tasks with an average rank of 1.75. Relatively, ECC has good performance in classification tasks and MAT good for regression (both ranked 1st for 3 tasks) with an average rank of 2.71 and 2.6, respectively.
Availability The source code is available at:
Supplementary information Supplementary data are available online.
### Competing Interest Statement
The authors have declared no competing interest.
更多查看译文
关键词
molecular property prediction,deep representation learning library
AI 理解论文
溯源树
样例
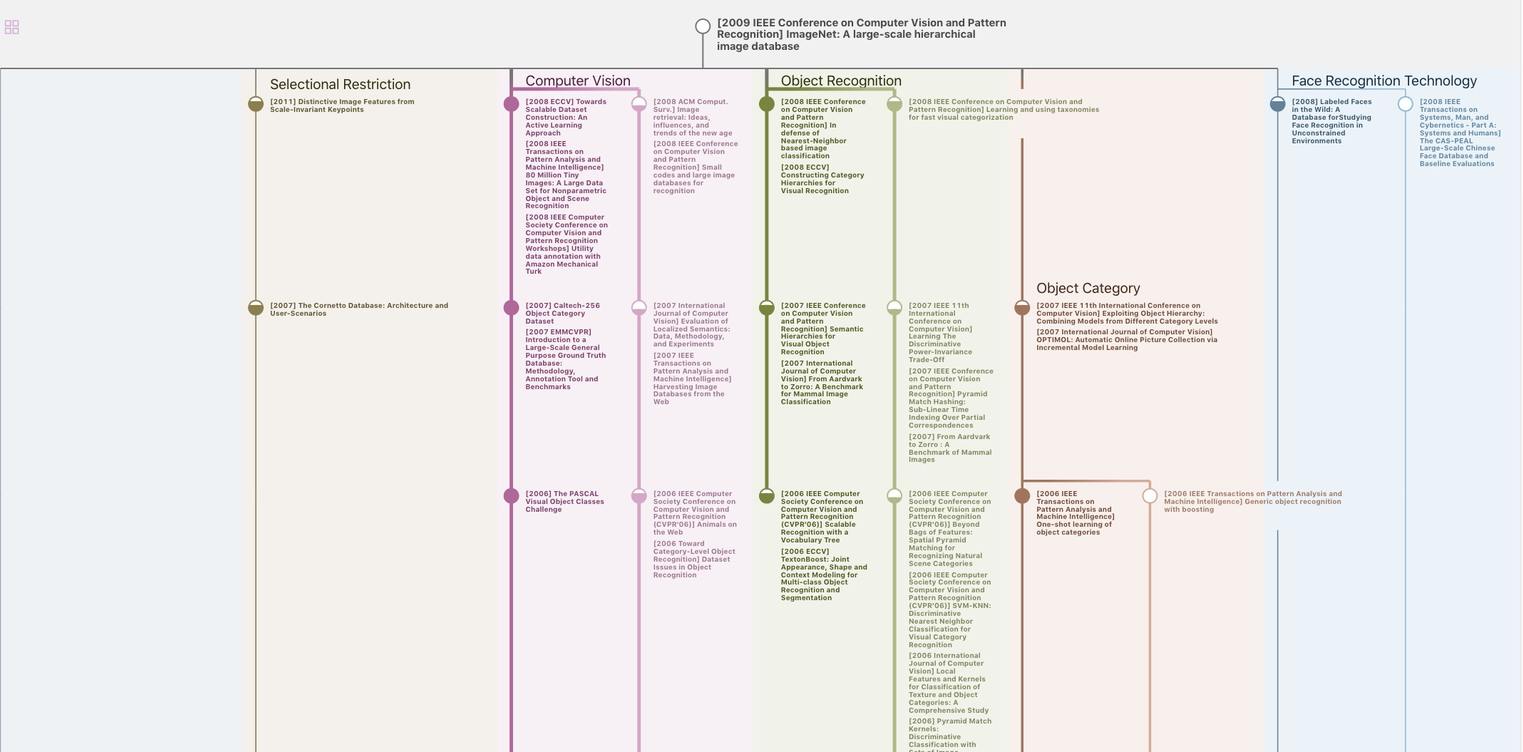
生成溯源树,研究论文发展脉络
Chat Paper
正在生成论文摘要