Learning Models for Actionable Recourse.
Annual Conference on Neural Information Processing Systems(2021)
摘要
As machine learning models are increasingly deployed in high-stakes domains such as legal and financial decision-making, there has been growing interest in post-hoc methods for generating counterfactual explanations. Such explanations provide individuals adversely impacted by predicted outcomes (e.g., an applicant denied a loan) with recourse---i.e., a description of how they can change their features to obtain a positive outcome. We propose a novel algorithm that leverages adversarial training and PAC confidence sets to learn models that theoretically guarantee recourse to affected individuals with high probability without sacrificing accuracy. We demonstrate the efficacy of our approach via extensive experiments on real data.
更多查看译文
关键词
Counterfactual thinking,Outcome (game theory),Loan,Adversarial system,Machine learning,Computer science,Artificial intelligence,High probability,Learning models
AI 理解论文
溯源树
样例
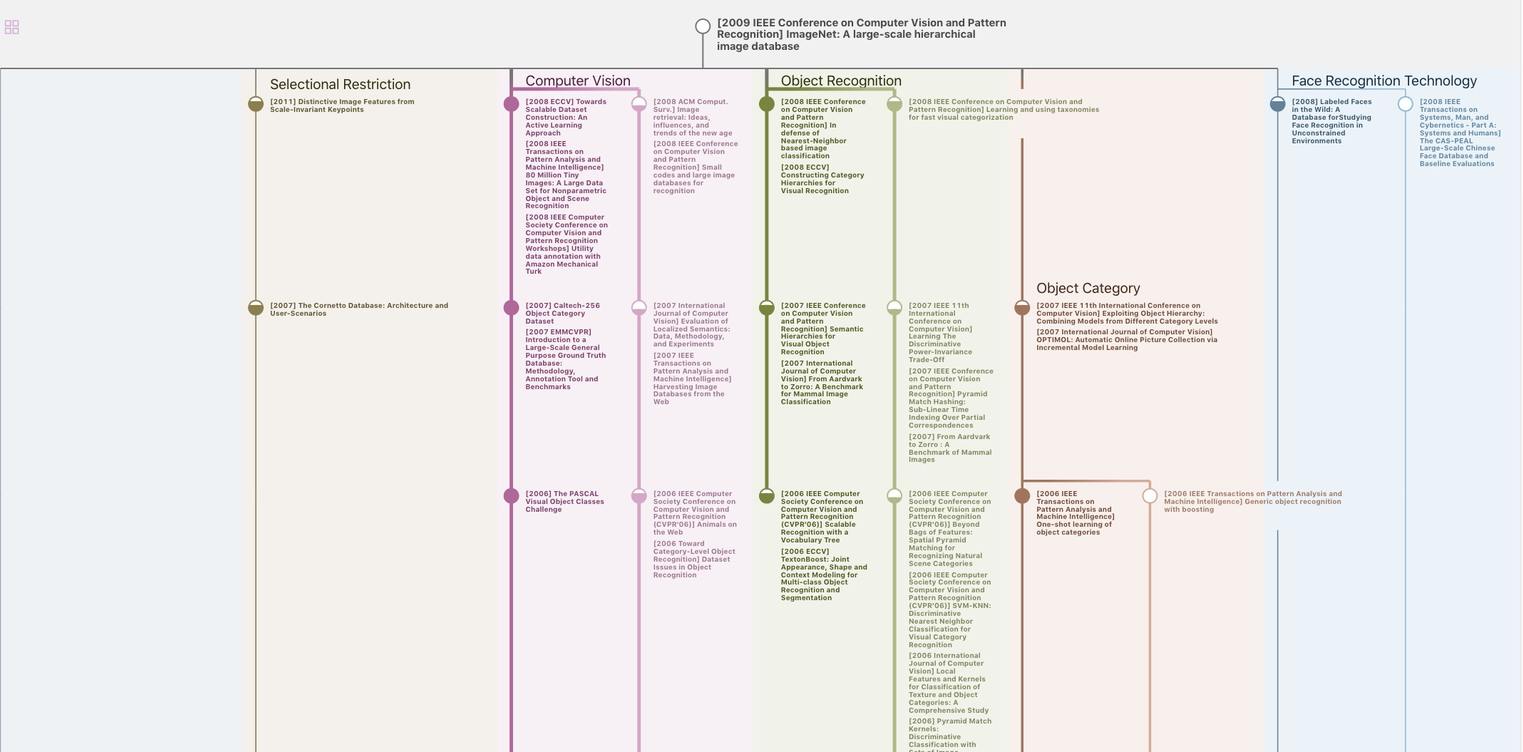
生成溯源树,研究论文发展脉络
Chat Paper
正在生成论文摘要