Hierarchical Graph Convolutional Networks for Jointly Resolving Cross-document Coreference of Entity and Event Mentions
Proceedings of the Fifteenth Workshop on Graph-Based Methods for Natural Language Processing (TextGraphs-15)(2021)
摘要
This paper studies the problem of cross-document event coreference resolution (CDECR) that seeks to determine if event mentions across multiple documents refer to the same real-world events. Prior work has demonstrated the benefits of the predicate-argument information and document context for resolving the coreference of event mentions. However, such information has not been captured effectively in prior work for CDECR. To address these limitations, we propose a novel deep learning model for CDECR that introduces hierarchical graph convolutional neural networks (GCN) to jointly resolve entity and event mentions. As such, sentence-level GCNs enable the encoding of important context words for event mentions and their arguments while the document-level GCN leverages the interaction structures of event mentions and arguments to compute document representations to perform CDECR. Extensive experiments are conducted to demonstrate the effectiveness of the proposed model.
更多查看译文
关键词
hierarchical graph convolutional networks,event mentions,entity,cross-document
AI 理解论文
溯源树
样例
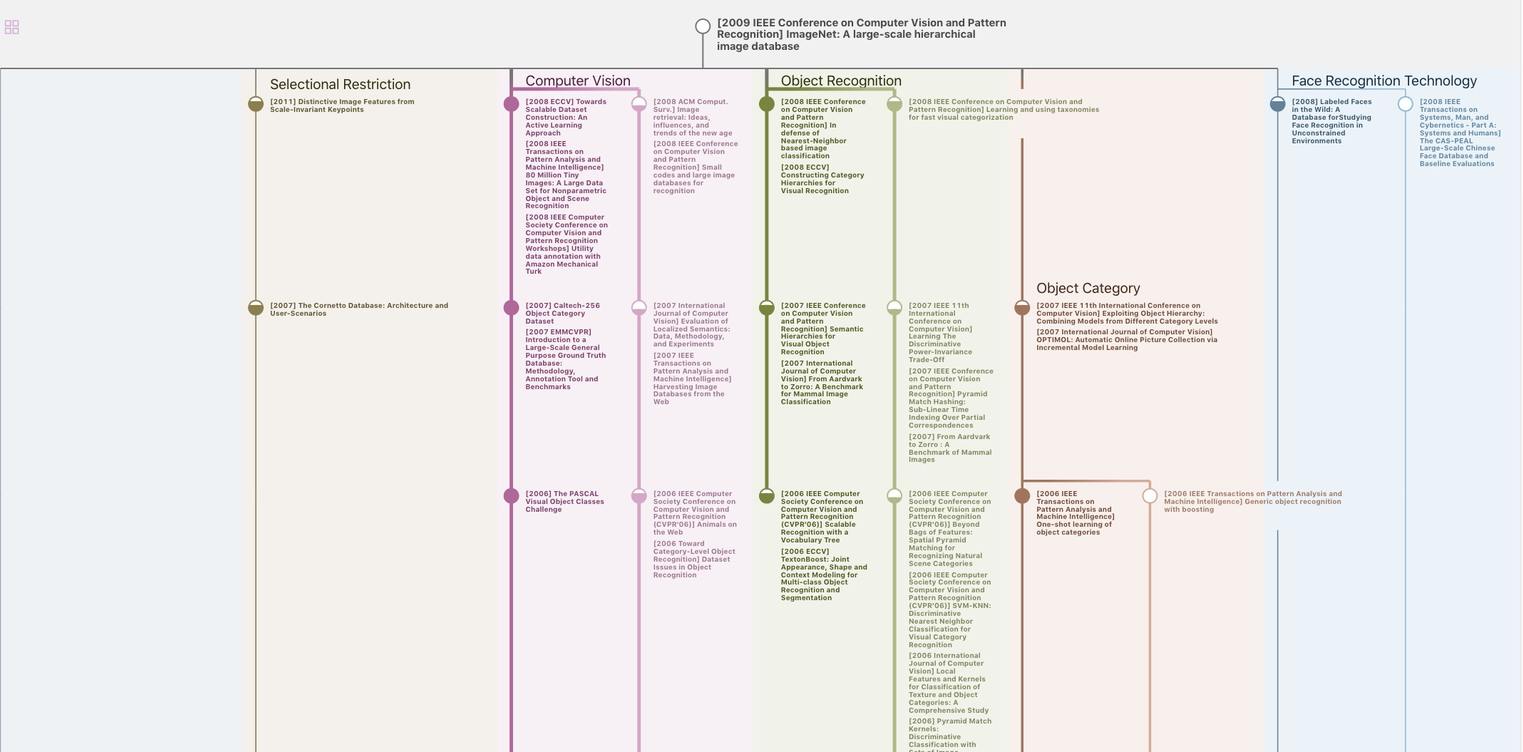
生成溯源树,研究论文发展脉络
Chat Paper
正在生成论文摘要