Tensor Programs V: Tuning Large Neural Networks Via Zero-Shot Hyperparameter Transfer
ArXiv(2022)
摘要
Hyperparameter (HP) tuning in deep learning is an expensive process, prohibitively so for neural networks (NNs) with billions of parameters. We show that, in the recently discovered Maximal Update Parametrization (mu P), many optimal HPs remain stable even as model size changes. This leads to a new HP tuning paradigm we call mu Transfer: parametrize the target model in mu P, tune the HP indirectly on a smaller model, and zero-shot transfer them to the full-sized model, i.e., without directly tuning the latter at all. We verify mu Transfer on Transformer and ResNet. For example, 1) by transferring pretraining HPs from a model of 13M parameters, we outperform published numbers of BERT-large (350M parameters), with a total tuning cost equivalent to pretraining BERT-large once; 2) by transferring from 40M parameters, we outperform published numbers of the 6.7B GPT-3 model, with tuning cost only 7% of total pretraining cost. A Pytorch implementation of our technique can be found at github.com/microsoft/mup.(2)
更多查看译文
关键词
Deep Learning,Backpropagation Learning,Statistical Modeling,Feedforward Neural Networks,Inverse Problems
AI 理解论文
溯源树
样例
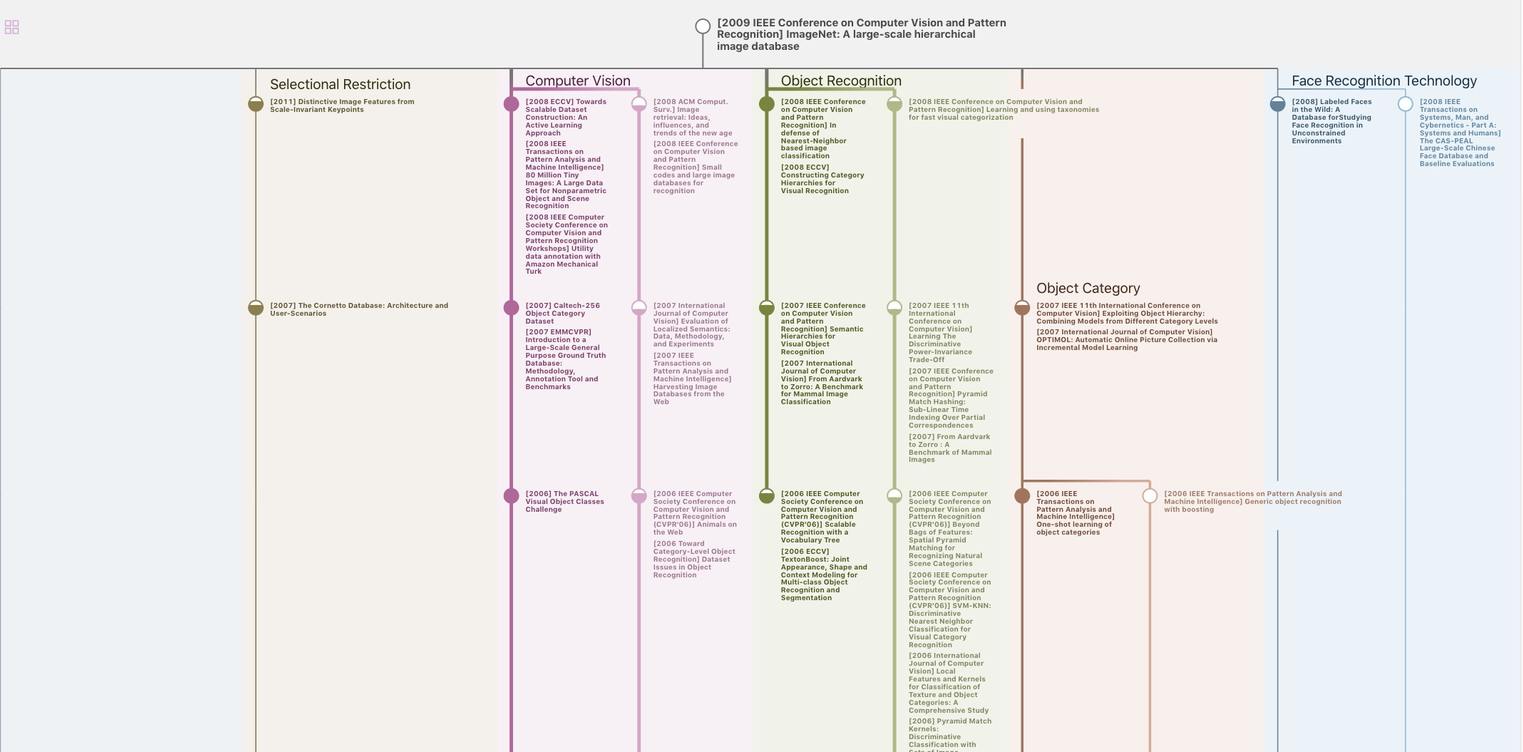
生成溯源树,研究论文发展脉络
Chat Paper
正在生成论文摘要