Challenges and Opportunities in Approximate Bayesian Deep Learning for Intelligent IoT Systems
2021 IEEE Third International Conference on Cognitive Machine Intelligence (CogMI)(2021)
摘要
Approximate Bayesian deep learning methods hold significant promise for addressing several issues that occur when deploying deep learning components in intelligent systems, including mitigating the occurrence of over-confident errors and providing enhanced robustness to out of distribution examples. However, the computational requirements of existing approxi-mate Bayesian inference methods can make them ill-suited for deployment in intelligent IoT systems that include lower-powered edge devices. In this paper, we present a range of approximate Bayesian inference methods for supervised deep learning and highlight the challenges and opportunities when applying these methods on current edge hardware. We highlight several potential solutions to decreasing model storage requirements and improving computational scalability, including model pruning and distillation methods.
更多查看译文
关键词
Bayesian deep learning,Internet of Things,Deep Learning,Model Compression
AI 理解论文
溯源树
样例
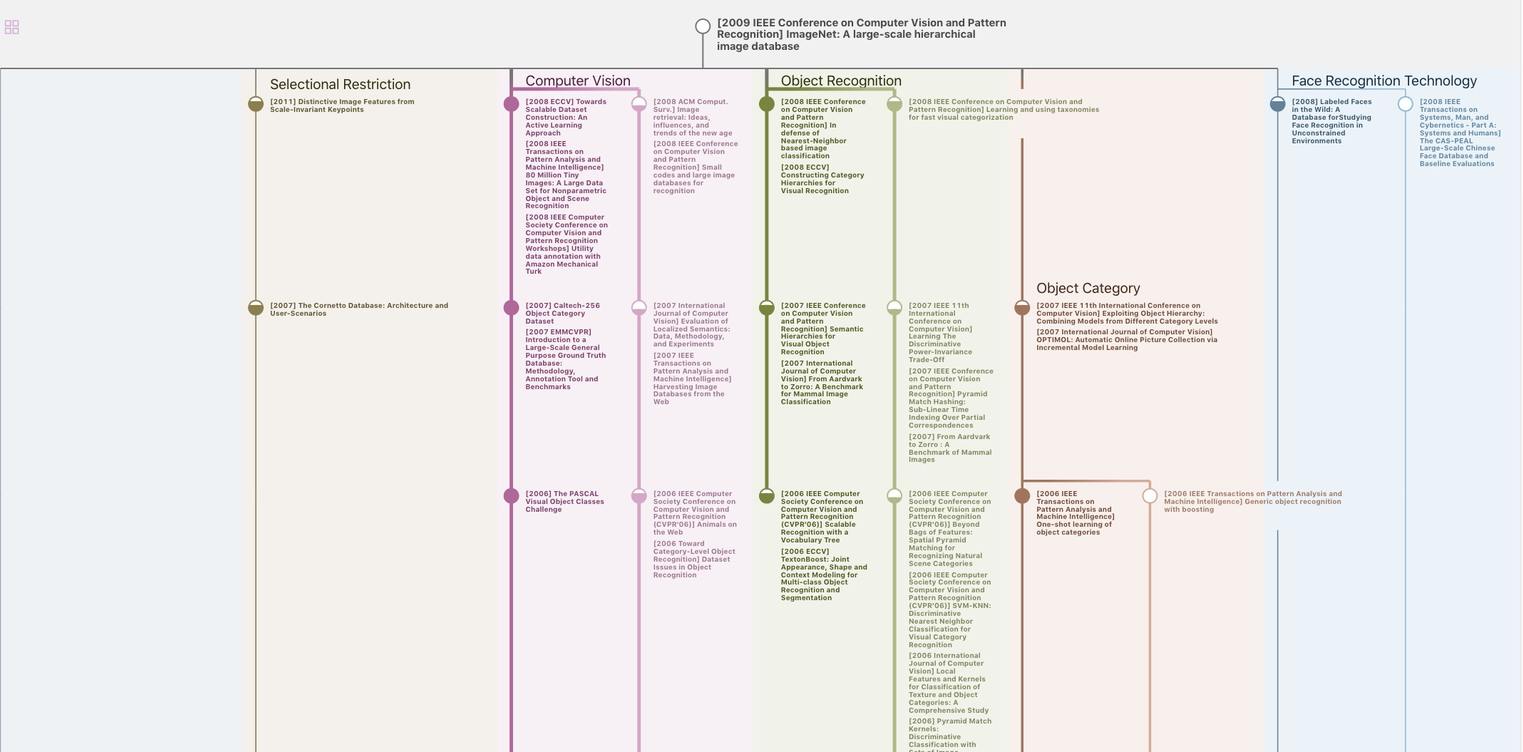
生成溯源树,研究论文发展脉络
Chat Paper
正在生成论文摘要