Variational Quantum Soft Actor-Critic
arxiv(2021)
摘要
Quantum computing has a superior advantage in tackling specific problems, such as integer factorization and Simon's problem. For more general tasks in machine learning, by applying variational quantum circuits, more and more quantum algorithms have been proposed recently, especially in supervised learning and unsupervised learning. However, little work has been done in reinforcement learning, arguably more important and challenging. Previous work in quantum reinforcement learning mainly focuses on discrete control tasks where the action space is discrete. In this work, we develop a quantum reinforcement learning algorithm based on soft actor-critic -- one of the state-of-the-art methods for continuous control. Specifically, we use a hybrid quantum-classical policy network consisting of a variational quantum circuit and a classical artificial neural network. Tested in a standard reinforcement learning benchmark, we show that this quantum version of soft actor-critic is comparable with the original soft actor-critic, using much less adjustable parameters. Furthermore, we analyze the effect of different hyper-parameters and policy network architectures, pointing out the importance of architecture design for quantum reinforcement learning.
更多查看译文
关键词
quantum,actor-critic
AI 理解论文
溯源树
样例
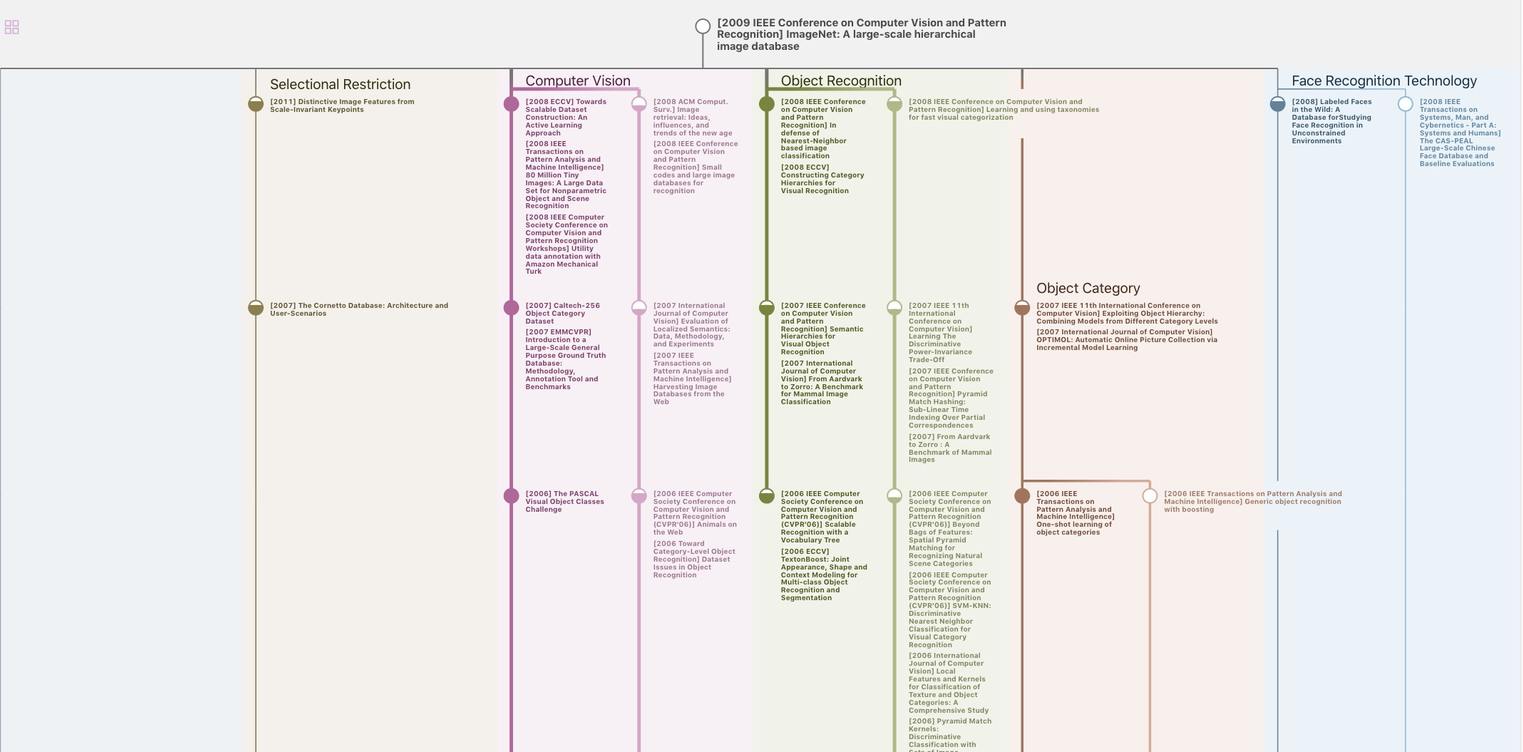
生成溯源树,研究论文发展脉络
Chat Paper
正在生成论文摘要