Solving Occlusion in Terrain Mapping with Neural Networks
ArXiv(2021)
摘要
Accurate and complete terrain maps enhance the awareness of autonomous robots and enable safe and optimal path planning. Rocks and topography often create occlusions and lead to missing elevation information in the Digital Elevation Map (DEM). Currently, mostly traditional inpainting techniques based on diffusion or patch-matching are used by autonomous mobile robots to fill-in incomplete DEMs. These methods cannot leverage the high-level terrain characteristics and the geometric constraints of line of sight we humans use intuitively to predict occluded areas. We propose to use neural networks to reconstruct the occluded areas in DEMs. We introduce a self-supervised learning approach capable of training on real-world data without a need for ground-truth information. We accomplish this by adding artificial occlusion to the incomplete elevation maps constructed on a real robot by performing ray casting. We first evaluate a supervised learning approach on synthetic data for which we have the full ground-truth available and subsequently move to several realworld datasets. These real-world datasets were recorded during autonomous exploration of both structured and unstructured terrain with a legged robot, and additionally in a planetary scenario on Lunar analogue terrain. We state a significant improvement compared to the Telea and Navier-Stokes baseline methods both on synthetic terrain and for the real-world datasets. Our neural network is able to run in real-time on both CPU and GPU with suitable sampling rates for autonomous ground robots.
更多查看译文
关键词
terrain mapping,occlusion,neural networks
AI 理解论文
溯源树
样例
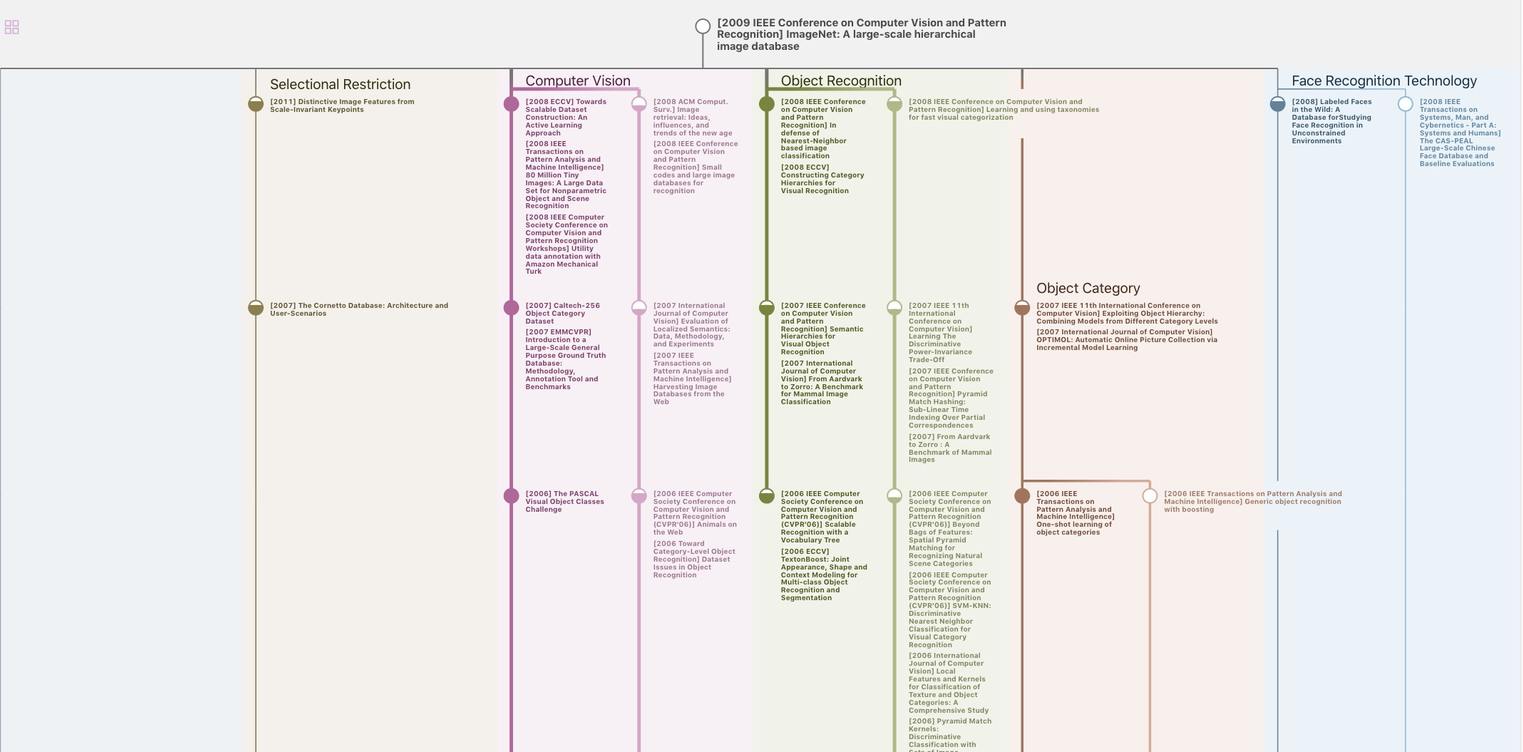
生成溯源树,研究论文发展脉络
Chat Paper
正在生成论文摘要