Predicting hospitalizations related to ambulatory care sensitive conditions with machine learning for population health planning: derivation and validation cohort study
medRxiv(2021)
摘要
Objective To predict older adults’ risk of avoidable hospitalization related to ambulatory care sensitive conditions (ACSC) using machine learning applied to administrative health data of Ontario, Canada.
Design, Setting, and Participants A retrospective cohort study was conducted on a large cohort of all residents covered under a single-payer system in Ontario, Canada over the period of 10 years, between 2008 and 2017. The study included 1.85 million Ontario residents between 65 and 74 years old at any time throughout the study period.
Data sources Administrative health data from Ontario, Canada obtained from the ICES Data Repository.
Main outcome measures Risk of hospitalizations due to ACSCs one year after the observation period.
Results The study used a total of 1,854,116 patients, split into train, validation, and test sets. The ACSC incidence rates among the data points were 1.1% for all sets. The final XGBoost model achieved an AUC of 80.5% on the held-out test set, and the predictions were well-calibrated. When ranking the predictions made by the model, those at the top 5% of risk as predicted by the model captured 37.4% of those presented with an ACSC-related hospitalization. A variety of features such as the previous number of ambulatory care visits, presence of ACSC-related hospitalizations during the observation window, age, rural residence, and prescription of certain medications were contributors to the prediction. Our model was also able to capture the geospatial heterogeneity of ACSC risk in the province of Ontario, and especially the elevated risk in rural and marginalized regions.
Conclusions This study aimed to predict the 1-year risk of hospitalization from a series of ambulatory-care sensitive conditions in seniors aged 65 to 74 years old with a single, large-scale machine learning model. The model shows the potential to inform population health planning and interventions to reduce the burden of ACSC-related hospitalizations.
### Competing Interest Statement
All authors have completed the ICMJE uniform disclosure form at www.icmje.org/coi_disclosure.pdf and declare: SY, JG, MR, MV, and TP are full-time employees of Layer 6 AI, co-founded by MV and TP, owned by Toronto-Dominion Bank. VH, KK, and TW are employed at the Dalla Lana School of Public Health. The employers of the authors had no role in the design or funding of this research.
### Funding Statement
This study was supported by the New Frontiers in Research Fund (NFRFE-2018-00662). LR is supported by a Canada Research Chair in Population Health Analytics (950-230702). VH is supported by the Ontario Graduate Scholarship and Canadian Institutes of Health Research Banting and Best Canada Graduate Scholarship-Master's awards. The analyses, conclusions, opinions, and statements reported and expressed herein are solely those of the authors and do not reflect those of the funding or data sources; no endorsement is intended or should be inferred.
### Author Declarations
I confirm all relevant ethical guidelines have been followed, and any necessary IRB and/or ethics committee approvals have been obtained.
Yes
The details of the IRB/oversight body that provided approval or exemption for the research described are given below:
ICES has obtained ethical approval (and repeats this review tri-annually) for its privacy and security policies, procedures, and practices. Each research project that is conducted at ICES is also subject to internal ethical review by the ICES Privacy and Compliance Office. ICES is a prescribed entity under section 45 of Ontario's Personal Health Information Protection Act (PHIPA). Section 45 is the provision that enables analysis and compilation of statistical information related to the management, evaluation, and monitoring of, allocation of resources to, and planning for the health system. Section 45 authorizes health information custodians to disclose personal health information to a prescribed entity, like ICES, without consent for such purposes. Projects conducted wholly under section 45, by definition, do not require review by a Research Ethics Board. As a prescribed entity, ICES must submit to trio-annual review and approval of its privacy and security policies, procedures and practices by Ontario's Information and Privacy Commissioner. These include policies, practices and procedures that require internal review and approval of every project by ICES' Privacy and Compliance Office.
All necessary patient/participant consent has been obtained and the appropriate institutional forms have been archived.
Yes
I understand that all clinical trials and any other prospective interventional studies must be registered with an ICMJE-approved registry, such as ClinicalTrials.gov. I confirm that any such study reported in the manuscript has been registered and the trial registration ID is provided (note: if posting a prospective study registered retrospectively, please provide a statement in the trial ID field explaining why the study was not registered in advance).
Yes
I have followed all appropriate research reporting guidelines and uploaded the relevant EQUATOR Network research reporting checklist(s) and other pertinent material as supplementary files, if applicable.
Yes
The dataset for this study is held securely in coded form at ICES. While data sharing agreements prohibit ICES from making the dataset publicly available, access may be granted to those who meet pre- specified criteria for confidential access, available at www.ices.on.ca/DAS. The full dataset creation plan is available from the authors upon request.
更多查看译文
关键词
population health planning,ambulatory care,hospitalizations,cohort study,machine learning
AI 理解论文
溯源树
样例
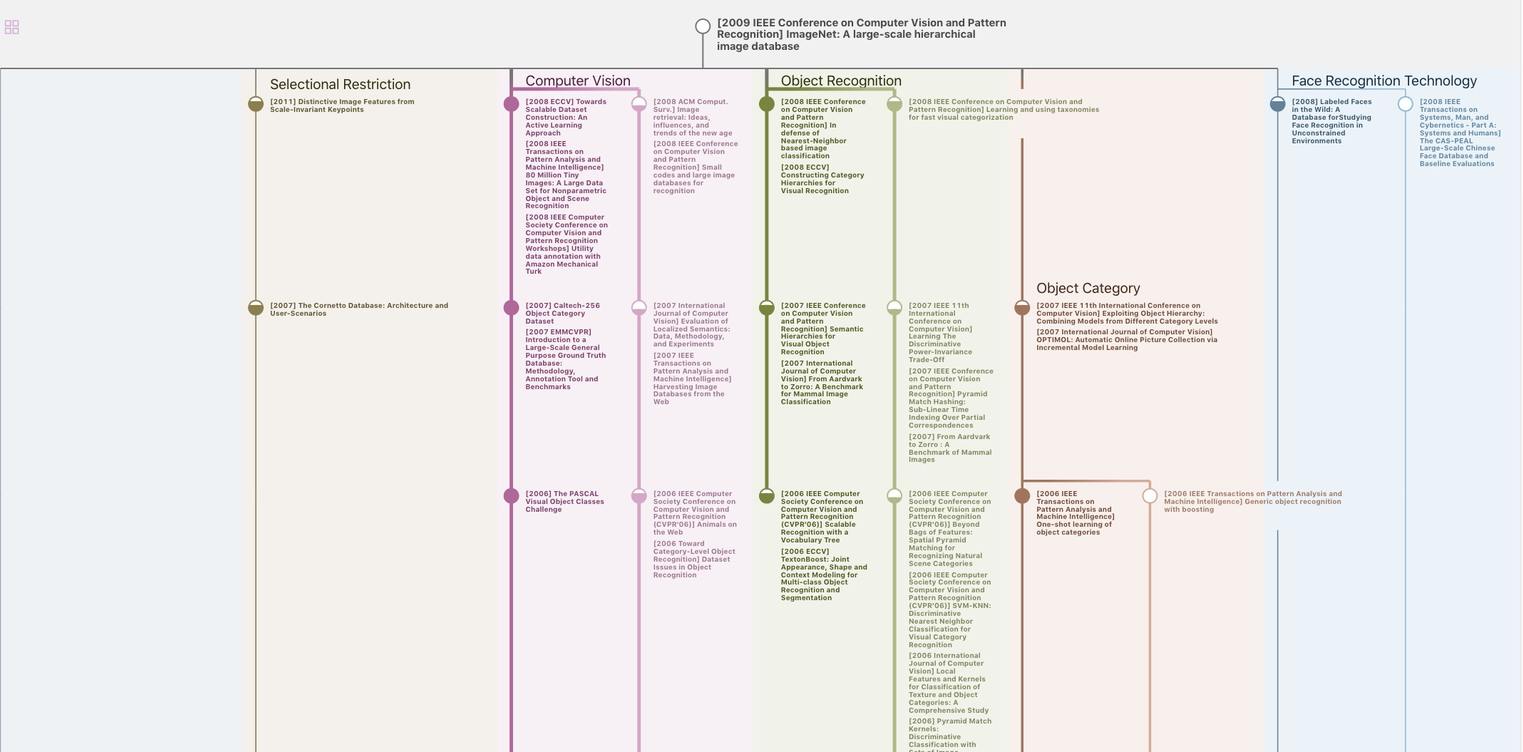
生成溯源树,研究论文发展脉络
Chat Paper
正在生成论文摘要