Semi-supervised Segmentation of Brain MRI Images
semanticscholar(2020)
摘要
While progress in neural network architectures has led to significant recent progress in the task of semantic segmentation, the challenge of obtaining large amounts of labeled segmentation masks has limited broad use in real-world applications such as medical image analysis. This has led to an emerging body of work focused on semi-supervised segmentation where a larger quantity of unlabeled data can be used with a smaller quantity of labeled data to train segmentation models. Recent work on semi-supervised classification has demonstrated that when simple techniques such as consistency regularization are used effectively, the gains in performance can be dramatic. In this work, we explore effective use of consistency regularization for semi-supervised segmentation, and show that when we use a consistency loss for segmentation in conjunction with a discriminator selecting informative labeled images, the resulting model significantly outperforms the prior body of work on semi-supervised semantic segmentation across multiple standard benchmarks. The code for our implementation is available at https://github.com/samottaghi/brainsegmentation.
更多查看译文
AI 理解论文
溯源树
样例
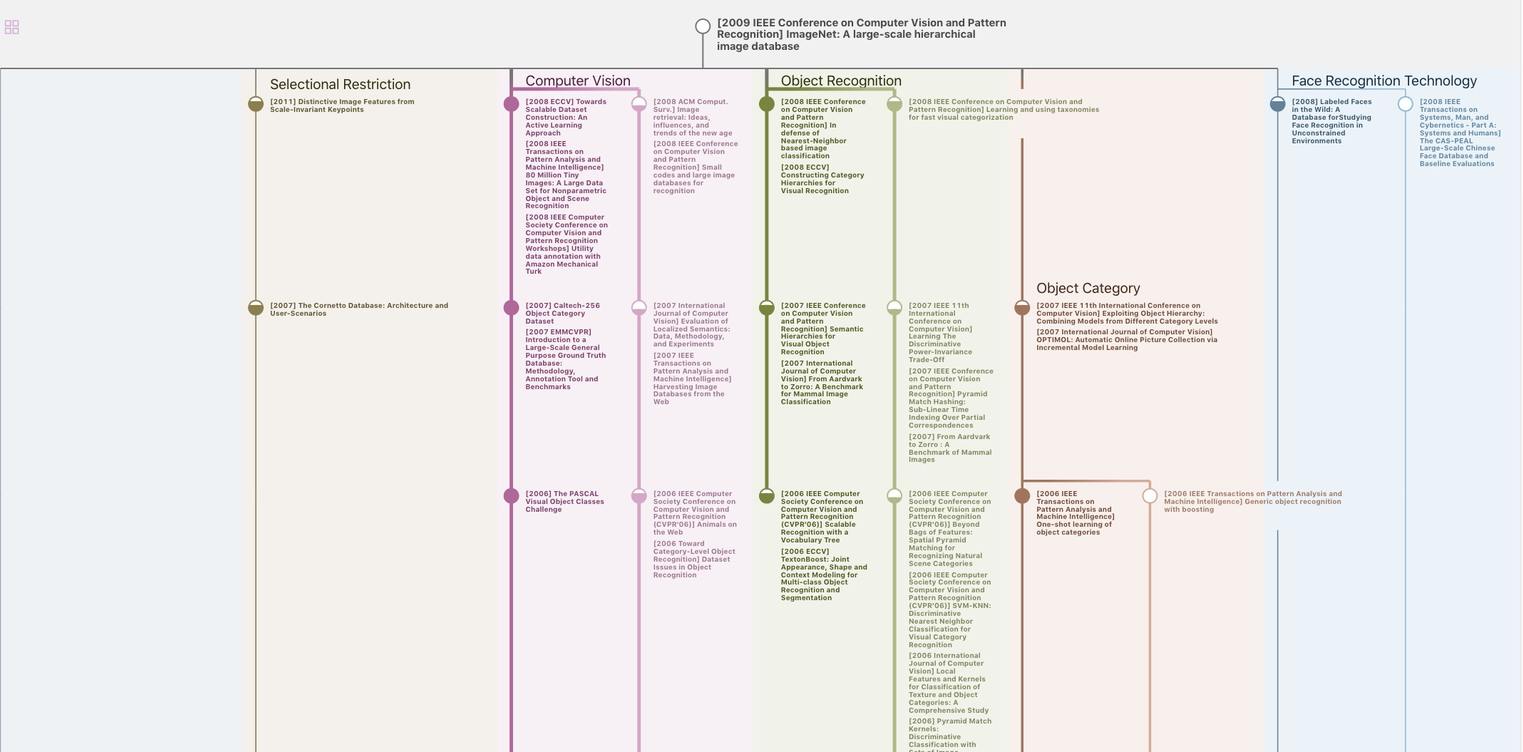
生成溯源树,研究论文发展脉络
Chat Paper
正在生成论文摘要