Safe Medicine Recommendation Via Star Interactive Enhanced-Based Transformer Model.
Computers in biology and medicine(2022)
摘要
With the rapid development of electronic medical records (EMRs), most existing medicine recommendation systems based on EMRs explore knowledge from the diagnosis history to help doctors prescribe medication correctly. However, due to the limitations of the EMRs' content, recommendation systems cannot explicitly reflect relevant medical data, such as drug interactions. In recent years, medicine recommendation approaches based on medical knowledge graphs and graph neural networks have been proposed, and the methods based on the Transformer model have been widely used in medicine recommendation systems. Transformer-based medicine recommendation approaches are readily applicable to inductive problems. Unfortunately, traditional Transformer-based medicine recommendation approaches require complex computing power and suffer information loss among the multi-heads in Transformer model, which causes poor performance. At the same time, these approaches have rarely considered the side effects of drug interaction in traditional medical recommendation approaches. To overcome the drawbacks of the current medicine recommendation approaches, we propose a Star Interactive Enhanced-based Transformer (SIET) model. It first constructs a high-quality heterogeneous graph by bridging EMR (MIMIC-III) and a medical knowledge graph (ICD-9 ontology and DrugBank). Then, based on the constructed heterogeneous graph, it extracts a disease homogeneous graph, a medicine homogeneous graph, and a negative factors homogeneous graph to get auxiliary information of disease or drug (named enhanced neighbors). These are fed into the SIET model in conjunction with the relevant information in the EMRs to obtain representations of diseases and drugs. It finally generates the recommended drug list by calculating the cosine similarity between disease combination representations and drug combination representations. Extensive experiments on the MIMIC-III, DrugBank, and ICD-9 ontology datasets demonstrate the outstanding performance of our proposed model. Meanwhile, we show that our SIET model outperforms strong baselines on an inductive medicine recommendation task.
更多查看译文
关键词
Medicine recommendation,Star interactive enhanced-based transformer,Graph embedding
AI 理解论文
溯源树
样例
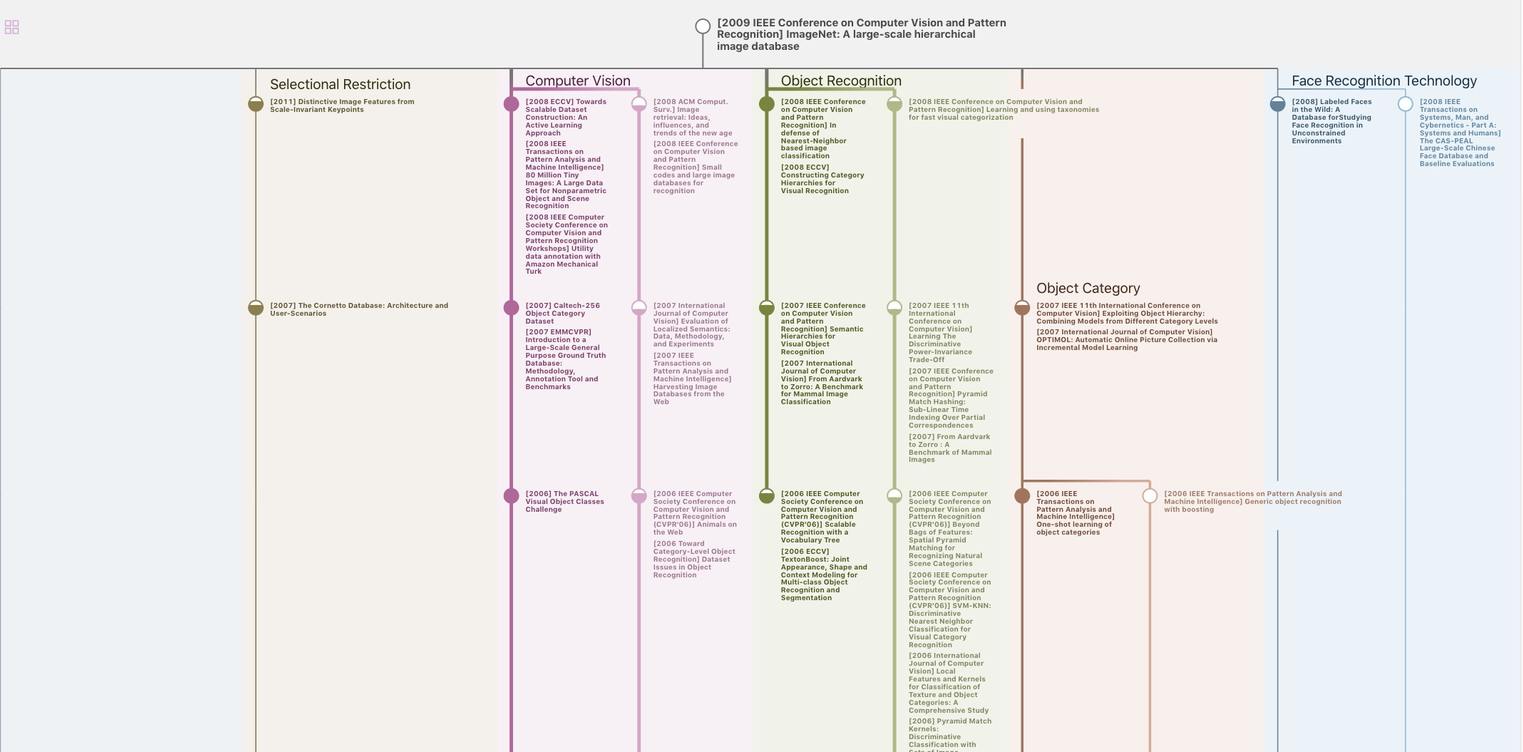
生成溯源树,研究论文发展脉络
Chat Paper
正在生成论文摘要