Fast and High Quality Image Denoising via Malleable Convolution.
European Conference on Computer Vision(2022)
摘要
Most image denoising networks apply a single set of static convolutional kernels across the entire input image. This is sub-optimal for natural images, as they often consist of heterogeneous visual patterns. Dynamic convolution tries to address this issue by using per-pixel convolution kernels, but this greatly increases computational cost. In this work, we present Malleable Convolution (MalleConv), which performs spatial-varying processing with minimal computational overhead. MalleConv uses a smaller set of spatially-varying convolution kernels, a compromise between static and per-pixel convolution kernels. These spatially-varying kernels are produced by an efficient predictor network running on a downsampled input, making them much more efficient to compute than per-pixel kernels produced by a full-resolution image, and also enlarging the network’s receptive field compared with static kernels. These kernels are then jointly upsampled and applied to a full-resolution feature map through an efficient on-the-fly slicing operator with minimum memory overhead. To demonstrate the effectiveness of MalleConv, we use it to build an efficient denoising network we call MalleNet. MalleNet achieves high-quality results without very deep architectures, making it 8.9\(\times \) faster than the best performing denoising algorithms while achieving similar visual quality. We also show that a single MalleConv layer added to a standard convolution-based backbone can significantly reduce the computational cost or boost image quality at a similar cost. More information are on our project page: https://yifanjiang.net/MalleConv.html.
更多查看译文
关键词
Image denoising,Dynamic kernel,Efficiency
AI 理解论文
溯源树
样例
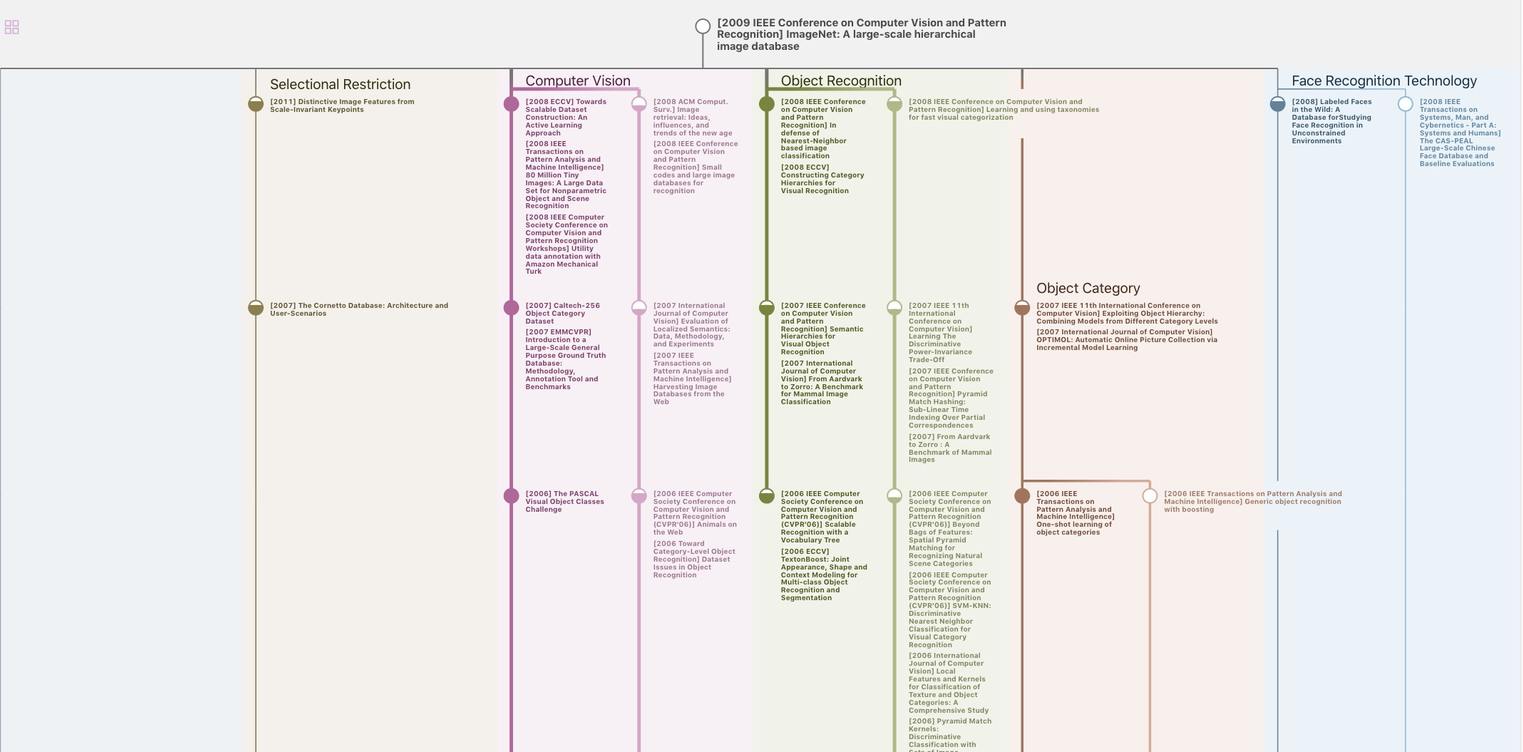
生成溯源树,研究论文发展脉络
Chat Paper
正在生成论文摘要