A Lightweight Full Homomorphic Encryption Scheme on Fully-connected Layer for CNN Hardware Accelerator achieving Security Inference
2021 28TH IEEE INTERNATIONAL CONFERENCE ON ELECTRONICS, CIRCUITS, AND SYSTEMS (IEEE ICECS 2021)(2021)
摘要
The inference results of neural network accelerators often involve personal privacy or business secrets in intelligent systems. It is important for the safety of convolutional neural network (CNN) accelerator to prevent the key data and inference result from being leaked. The latest CNN models have started to combine with fully homomorphic encryption (FHE), ensuring the data security. However, the computational complexity, data storage overhead, inference time are significantly increased compared with the traditional neural network models. This paper proposed a lightweight FHE scheme on fully-connected layer for CNN hardware accelerator to achieve security inference, which not only protects the privacy of inference results, but also avoids excessive hardware overhead and great performance degradation. Compared with state-of-the-art works, this work reduces computational complexity by approximately 90% and decreases ciphertext size by 87%similar to 95%.
更多查看译文
关键词
convolutional neural network, hardware accelerator, fully homomorphic encryption, lightweight, security inference
AI 理解论文
溯源树
样例
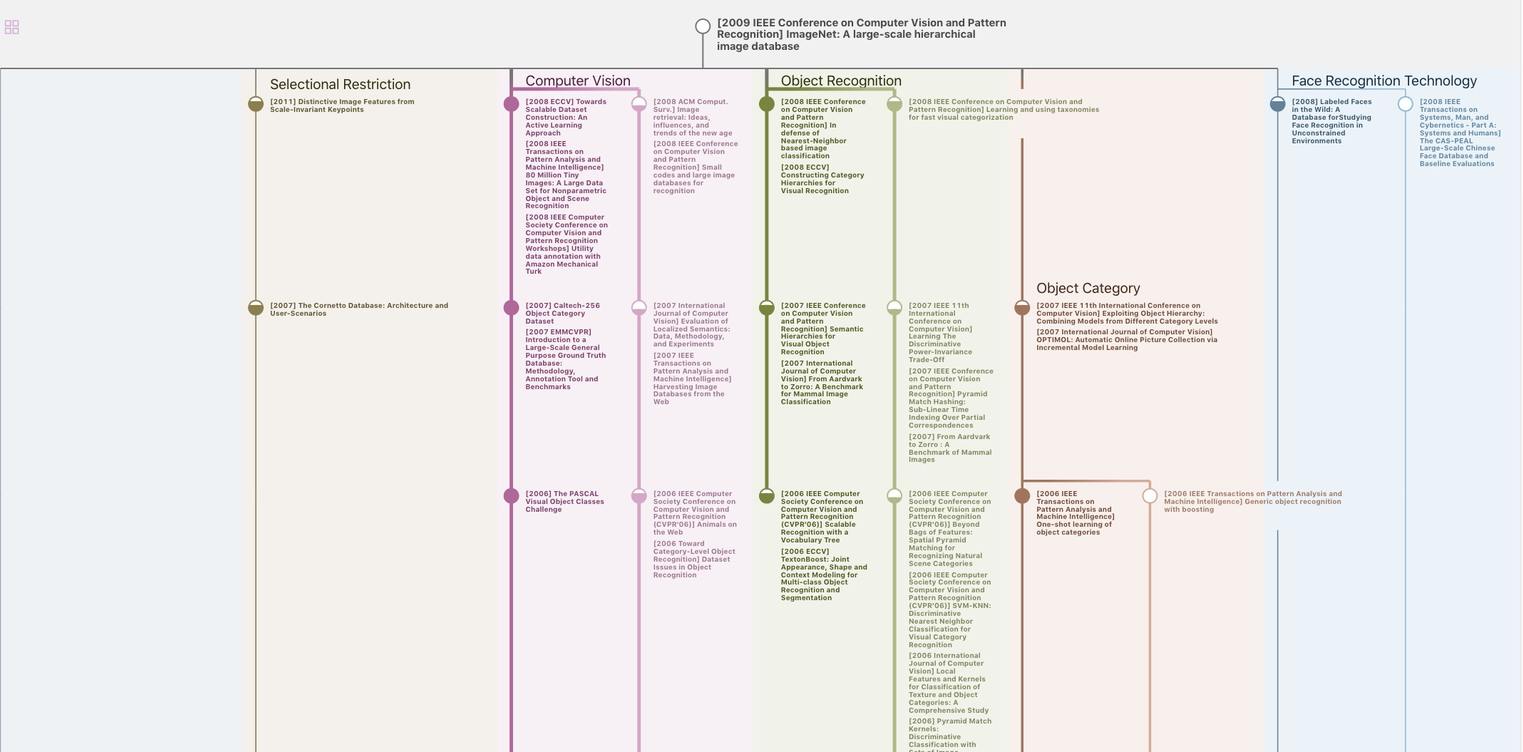
生成溯源树,研究论文发展脉络
Chat Paper
正在生成论文摘要