Retrieve-and-Fill for Scenario-based Task-Oriented Semantic Parsing
17TH CONFERENCE OF THE EUROPEAN CHAPTER OF THE ASSOCIATION FOR COMPUTATIONAL LINGUISTICS, EACL 2023(2023)
摘要
Task-oriented semantic parsing models have achieved strong results in recent years, but unfortunately do not strike an appealing balance between model size, runtime latency, and crossdomain generalizability. We tackle this problem by introducing scenario-based semantic parsing: a variant of the original task which separates disambiguating an utterance ' s '' scenario '' (an intent-slot template with variable leaf spans) and generating its frame, complete with ontology and utterance tokens. This formulation closely ties to the data collection process where the scenarios are first designed followed by crowd sourced utterance annotation. Concretely, we create a Retrieve-and-Fill (RAF) architecture comprised of (1) a retrieval module which ranks the best scenario given an utterance and (2) a filling module which imputes spans into the scenario to create the frame. Our model is modular, differentiable, interpretable, and allows us to garner extra supervision from scenarios. RAF achieves strong results in highresource, low-resource, and multilingual settings, outperforming recent approaches despite, using base pre-trained encoders and efficient decoding.
更多查看译文
AI 理解论文
溯源树
样例
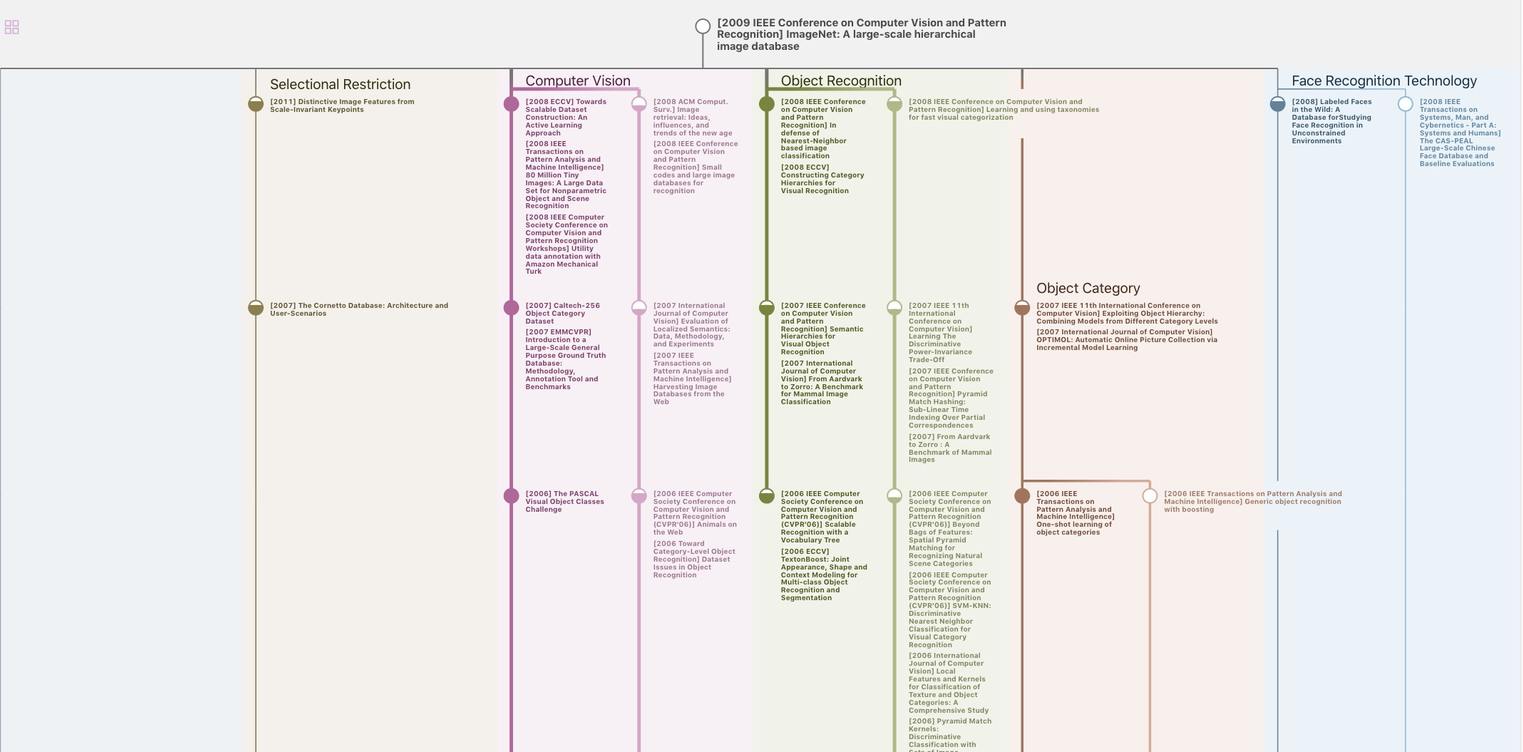
生成溯源树,研究论文发展脉络
Chat Paper
正在生成论文摘要