Detecting Distributional Differences in Labeled Sequence Data with Application to Tropical Cyclone Satellite Imagery
arxiv(2022)
摘要
Our goal is to quantify whether, and if so how, spatio-temporal patterns in tropical cyclone (TC) satellite imagery signal an upcoming rapid intensity change event. To address this question, we propose a new nonparametric test of association between a time series of images and a series of binary event labels. We ask whether there is a difference in distribution between (dependent but identically distributed) 24-h sequences of images preceding an event versus a non-event. By rewriting the statistical test as a regression problem, we leverage neural networks to infer modes of structural evolution of TC convection that are representative of the lead-up to rapid intensity change events. Dependencies between nearby sequences are handled by a bootstrap procedure that estimates the marginal distribution of the label series. We prove that type I error control is guaranteed as long as the distribution of the label series is well-estimated, which is made easier by the extensive historical data for binary TC event labels. We show empirical evidence that our proposed method identifies archetypes of infrared imagery associated with elevated rapid intensification risk, typically marked by deep or deepening core convection over time. Such results provide a foundation for improved forecasts of rapid intensification.
更多查看译文
关键词
Two-sample testing, high-dimensional time series, association studies, remote sens-ing, functional data, weather forecasting
AI 理解论文
溯源树
样例
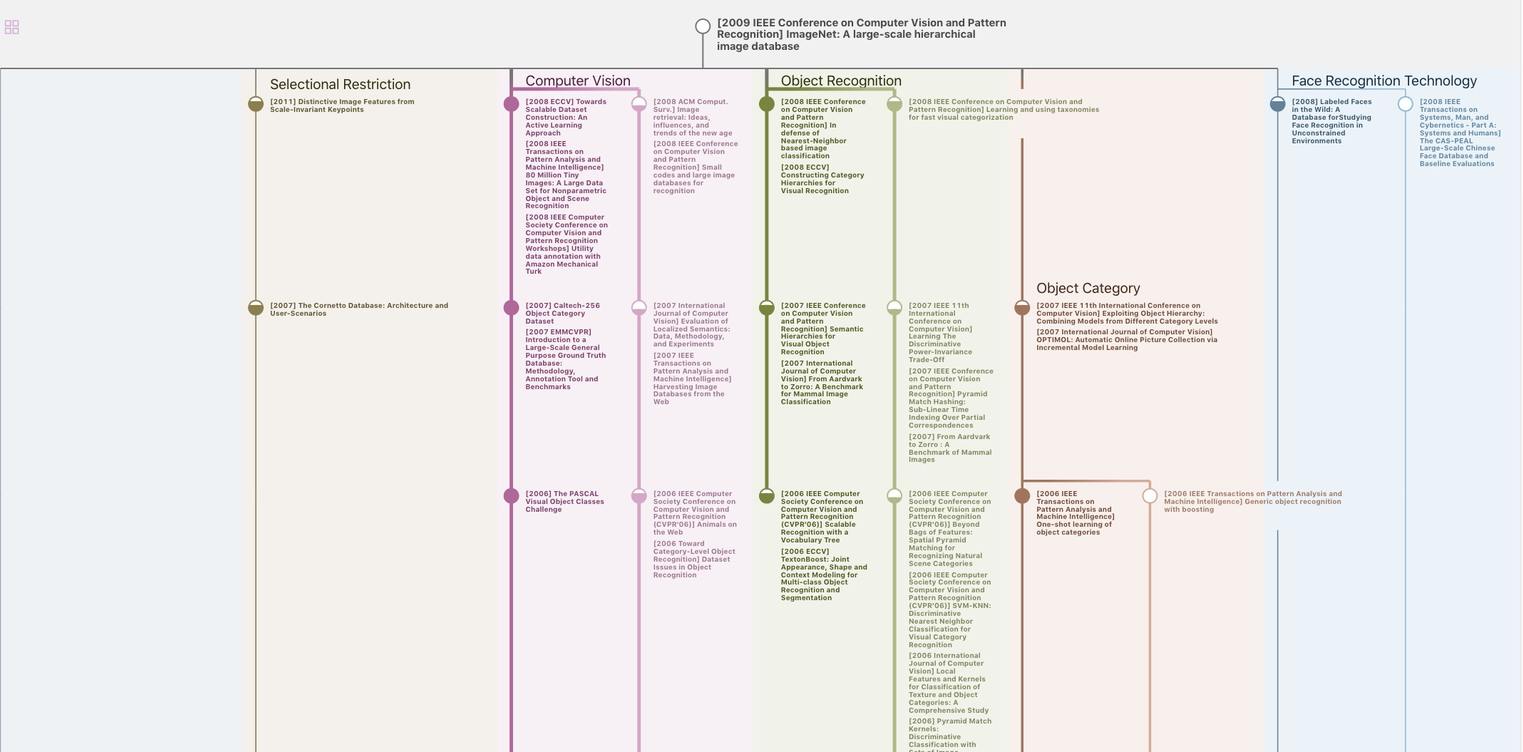
生成溯源树,研究论文发展脉络
Chat Paper
正在生成论文摘要