Semantic-Oriented Unlabeled Priming for Large-Scale Language Models
ArXiv(2022)
摘要
Due to the high costs associated with finetuning large language models, various recent works propose to adapt them to specific tasks without any parameter updates through in-context learning. Unfortunately, for in-context learning there is currently no way to leverage unlabeled data, which is often much easier to obtain in large quantities than labeled examples. In this work, we therefore investigate ways to make use of unlabeled examples to improve the zero-shot performance of pretrained language models without any finetuning: We introduce Semantic-Oriented Unlabeled Priming (SOUP), a method that classifies examples by retrieving semantically similar unlabeled examples, assigning labels to them in a zero-shot fashion, and then using them for in-context learning. We also propose bag-of-contexts priming, a new priming strategy that is more suitable for our setting and enables the usage of more examples than fit into the context window.
更多查看译文
关键词
unlabeled priming,language models,semantic-oriented,large-scale
AI 理解论文
溯源树
样例
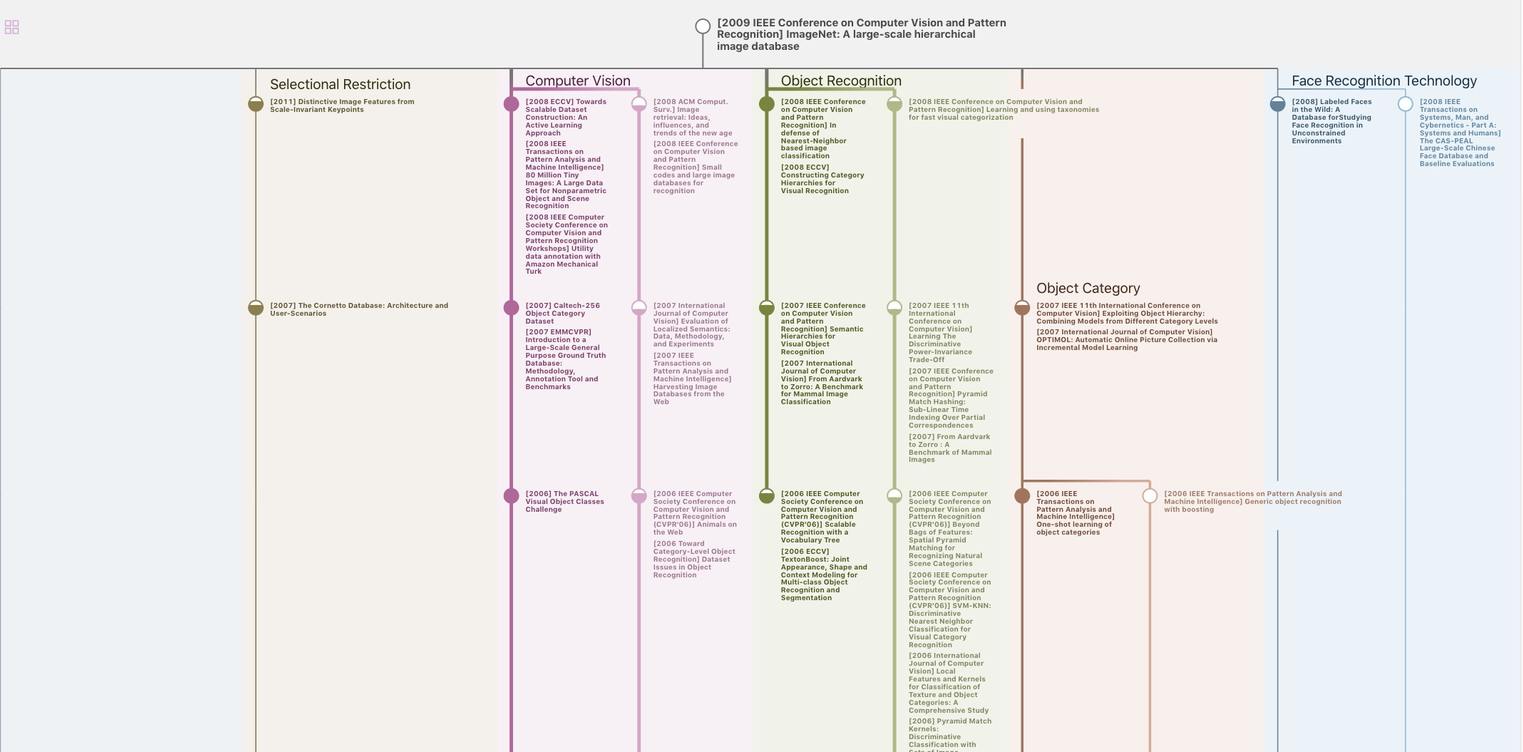
生成溯源树,研究论文发展脉络
Chat Paper
正在生成论文摘要