Summarizing User-Item Matrix By Group Utility Maximization
2021 21ST IEEE INTERNATIONAL CONFERENCE ON DATA MINING (ICDM 2021)(2021)
摘要
A user-item matrix conveniently represents the utility measure associated with (user, item) pairs, such as citation counts, users' rating/vote on items or locations, and clicks on items. A high utility value indicates a strong association of the pair. In this work, we consider the problem of summarizing strong associations for a large user-item matrix using a small summary size. The traditional techniques fail to distinguish user groups associated with different items, such as top-l item selection, or fail to focus on high utility, such as similarity based subspace clustering and biclustering. We define a new problem, called Group Utility Maximization, to summarize the entire user population through k groups and l items for each group; the goal is to maximize the sum of utility of selected items over all groups collectively. We propose the k-max algorithm for it, which iteratively refines existing k groups. We evaluate the proposed algorithm on two real-life datasets. The results provide an easyto-understand overview of the whole dataset efficiently.
更多查看译文
关键词
Data summarization,Greedy algorithm,k-max algorithm,group utility maximization
AI 理解论文
溯源树
样例
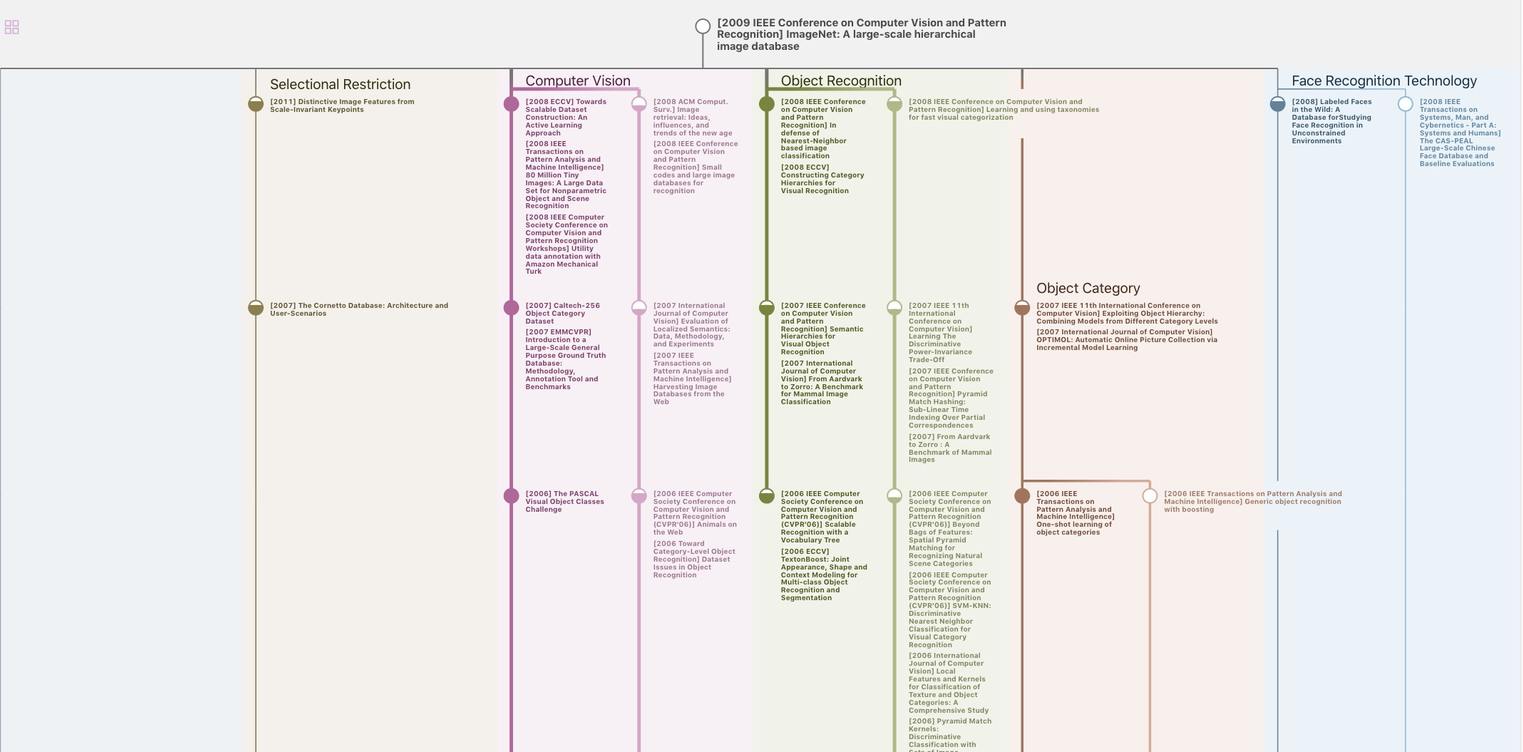
生成溯源树,研究论文发展脉络
Chat Paper
正在生成论文摘要