Equivariant Graph Attention Networks for Molecular Property Prediction
CoRR(2022)
摘要
Learning and reasoning about 3D molecular structures with varying size is an emerging and important challenge in machine learning and especially in drug discovery. Equivariant Graph Neural Networks (GNNs) can simultaneously leverage the geometric and relational detail of the problem domain and are known to learn expressive representations through the propagation of information between nodes leveraging higher-order representations to faithfully express the geometry of the data, such as directionality in their intermediate layers. In this work, we propose an equivariant GNN that operates with Cartesian coordinates to incorporate directionality and we implement a novel attention mechanism, acting as a content and spatial dependent filter when propagating information between nodes. We demonstrate the efficacy of our architecture on predicting quantum mechanical properties of small molecules and its benefit on problems that concern macromolecular structures such as protein complexes.
更多查看译文
关键词
networks,graph,prediction,attention
AI 理解论文
溯源树
样例
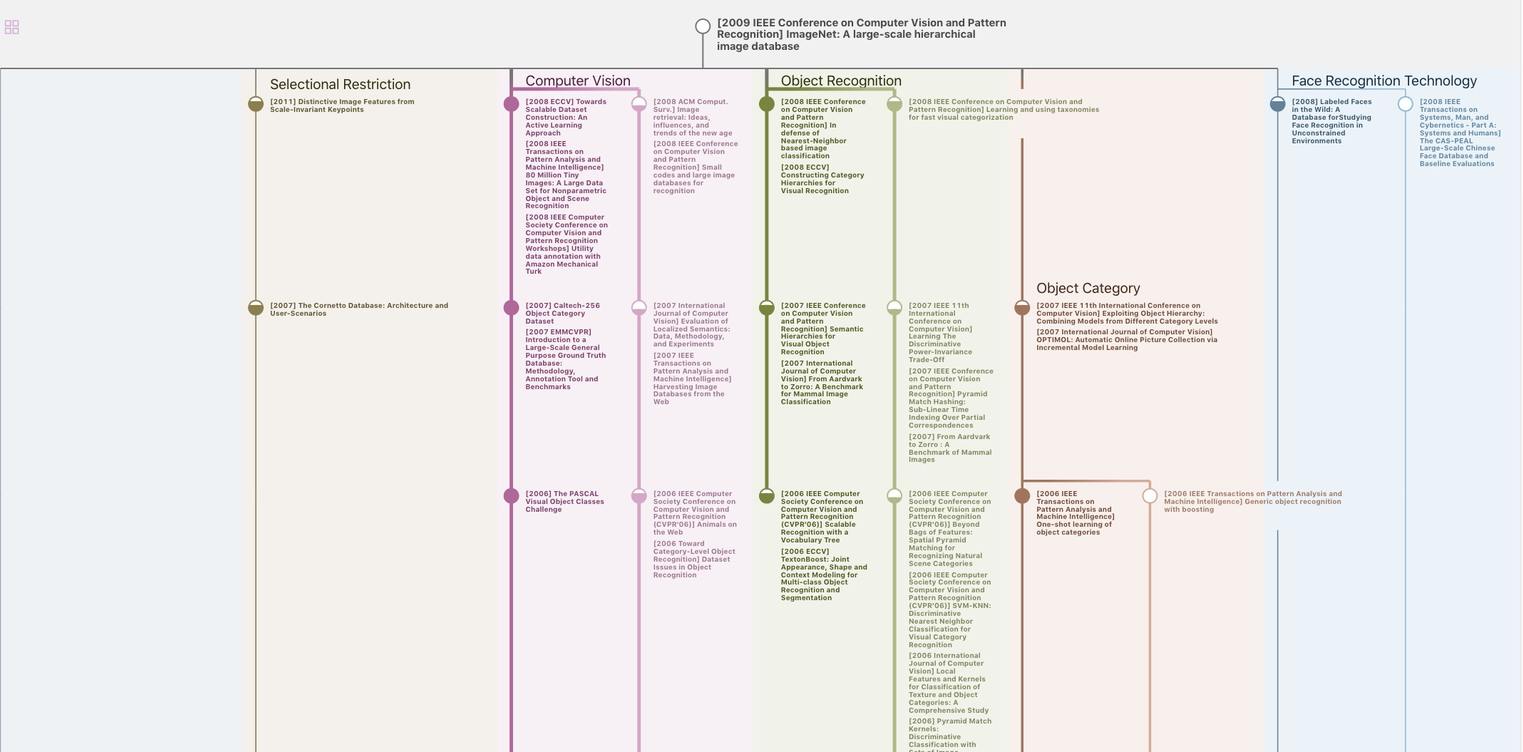
生成溯源树,研究论文发展脉络
Chat Paper
正在生成论文摘要