Wearable motion sensor-based chewing side detection
Smart Health(2021)
摘要
Abstract Chewing side preference means a tendency to use one side to chew food more frequently than the other. Medical studies show that chewing side preference can result in lateral facial asymmetry, teeth abrasion, temporomandibular disorders, malocclusion, and stomach illness. To continuously detect chewing side preference and quantify its severity in daily life, several wearable sensor-based methods have been proposed in recent years. However, these methods are either intrusive or not fine-grained enough. In this paper, we propose a wearable motion sensor-based chewing side detection method. We observe that chewing activity generates mastication muscle bulge and skull vibration, which can be sensed by motion sensors worn on the mastication muscles. In addition, the muscle bulge and skull vibration of the chewing side are different from those of the non-chewing side. These observations motivate us to deploy motion sensors on the left and right temporalis muscles to detect chewing sides. We propose a heuristic-rules based method to exclude non-chewing data and segment each chew accurately. The relative difference series of the left and right sensors are then calculated to characterize the difference of muscle bulge and skull vibration between the chewing side and the non-chewing side. A two-class classifier is trained using long short-term memory (LSTM), an artificial recurrent neural network, to model the data samples and classify chewing sides. A real-world evaluation dataset of eight food types is collected from eight human subjects. The average detection accuracy reaches 84.8%. The highest detection accuracy for a single subject is up to 97.4%.
更多查看译文
关键词
Chewing side preference,Wearable motion sensors,Muscle bulge,Skull vibration,LSTM
AI 理解论文
溯源树
样例
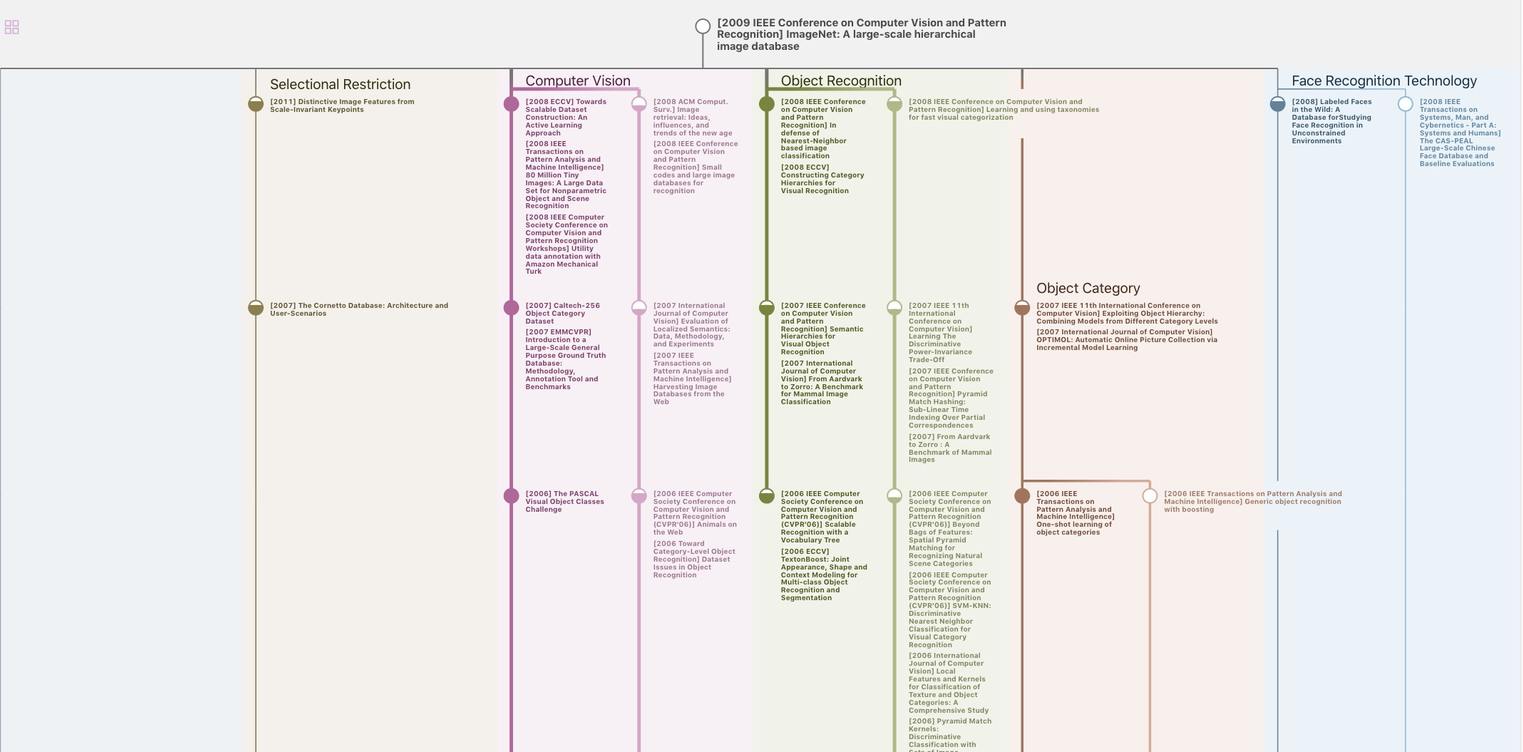
生成溯源树,研究论文发展脉络
Chat Paper
正在生成论文摘要