Fractional Chebyshev deep neural network (FCDNN) for solving differential models
CHAOS SOLITONS & FRACTALS(2021)
摘要
Differential and integral equations have been used vastly in modeling engineering and science problems. Solving these equations has been always an active and important area of research. In this paper, we propose the Fractional Chebyshev Deep Neural Network (FCDNN) for solving fractional differential models. Chebyshev orthogonal polynomials are basic functions in spectral methods. These functions are used as activation functions in FCDNN. The marching in time technique and the Gaussian method are applied in the fractional operations to simplify the calculations. We show how FCDNN can be used to solve fractional Fredholm integral equations (FFIEs). We also propose a solution to the extension of fractional time order partial differential equations (FPDEs). In this approach, fractional PDEs are first discretized by the finite difference and the marching in time methods and then are solved using FCDNN. Fractional Fred holm integral equations are also first approximated by the numerically Gaussian quadrature method and then are solved using FCDNN. A comparison between the results from FCDNN and some other methods is presented to validate the effectiveness and advance of the proposed method. (c) 2021 Elsevier Ltd. All rights reserved.
更多查看译文
关键词
Deep neural network, Chebyshev polynomials, Collocation method, Fractional partial differential equations, (FPDEs), Fractional Fredholm integral equations, (FFIEs)
AI 理解论文
溯源树
样例
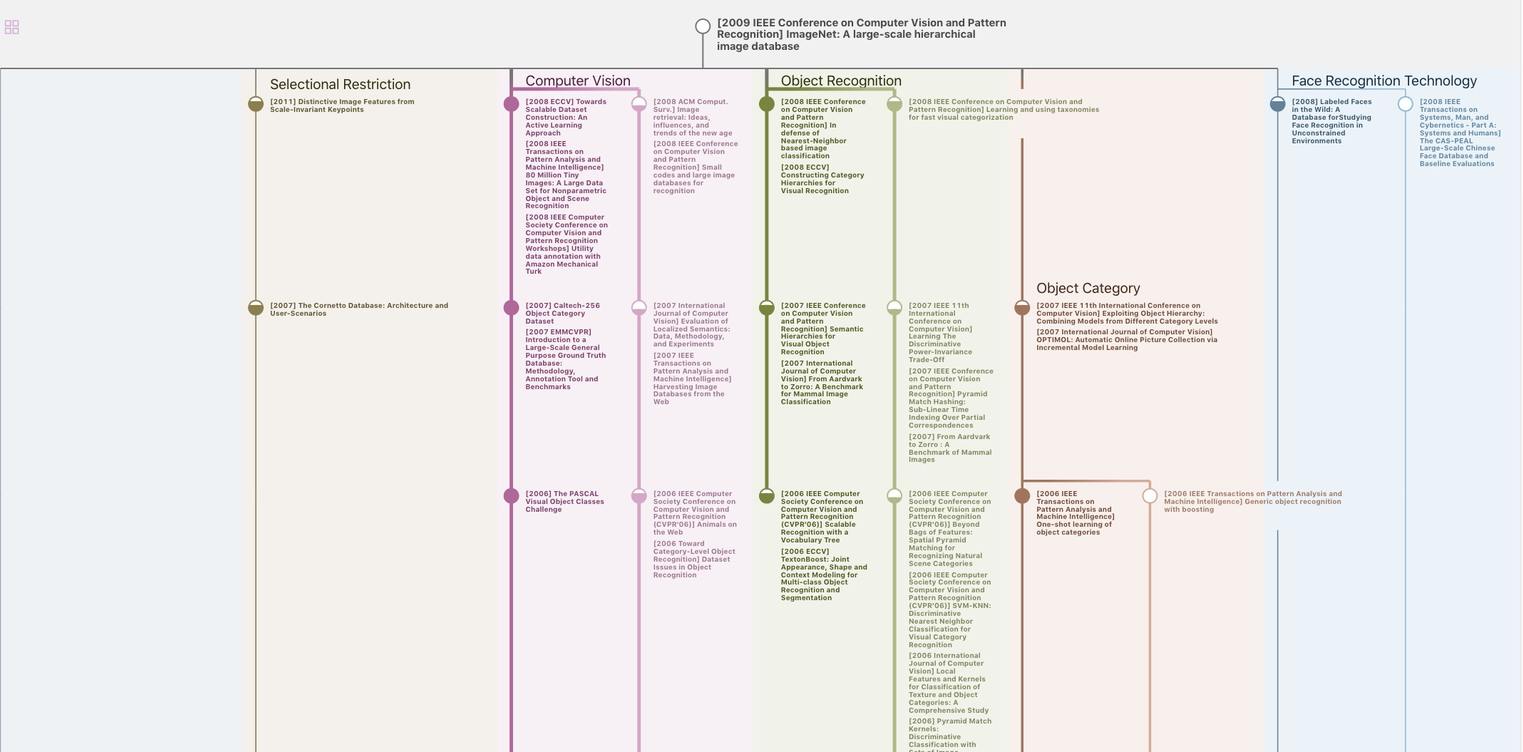
生成溯源树,研究论文发展脉络
Chat Paper
正在生成论文摘要