RFPruning: A retraining-free pruning method for accelerating convolutional neural networks
APPLIED SOFT COMPUTING(2021)
摘要
Network pruning has been developed as a remedy for accelerating the inference of deep convolutional neural networks (DCNNs). The mainstream methods retrain the pruned models, which maintain the performance of the pruned models but consume a great deal of time. While the other methods reduce the time consumption by omitting to retrain, they lose the performance. To resolve the above conflicts, we propose a two-stage Retraining-Free pruning method, named RFPruning, which embeds the rough screening of channels into training and fine-tunes the structures during pruning, to achieve both good performance and low time consumption. In the first stage, the network training is reformulated as an optimization problem with constraints and solved by a sparse learning approach for rough channel selection. In the second stage, the pruning process is regarded as a multiobjective optimization problem, where the genetic algorithm is applied to carefully select channels for a trade-off between the performance and model size. The proposed method is evaluated against several DCNNs on CIFAR-10 and ImageNet datasets. Extensive experiments demonstrate that such a retraining-free pruning method obtains 43.0% similar to 88.4% compression on model size and maintains the accuracy as the methods with retraining while achieving 3x speed up in pruning (C) 2021 Elsevier B.V. All rights reserved.
更多查看译文
关键词
DCNN,Network pruning,Retraining-free
AI 理解论文
溯源树
样例
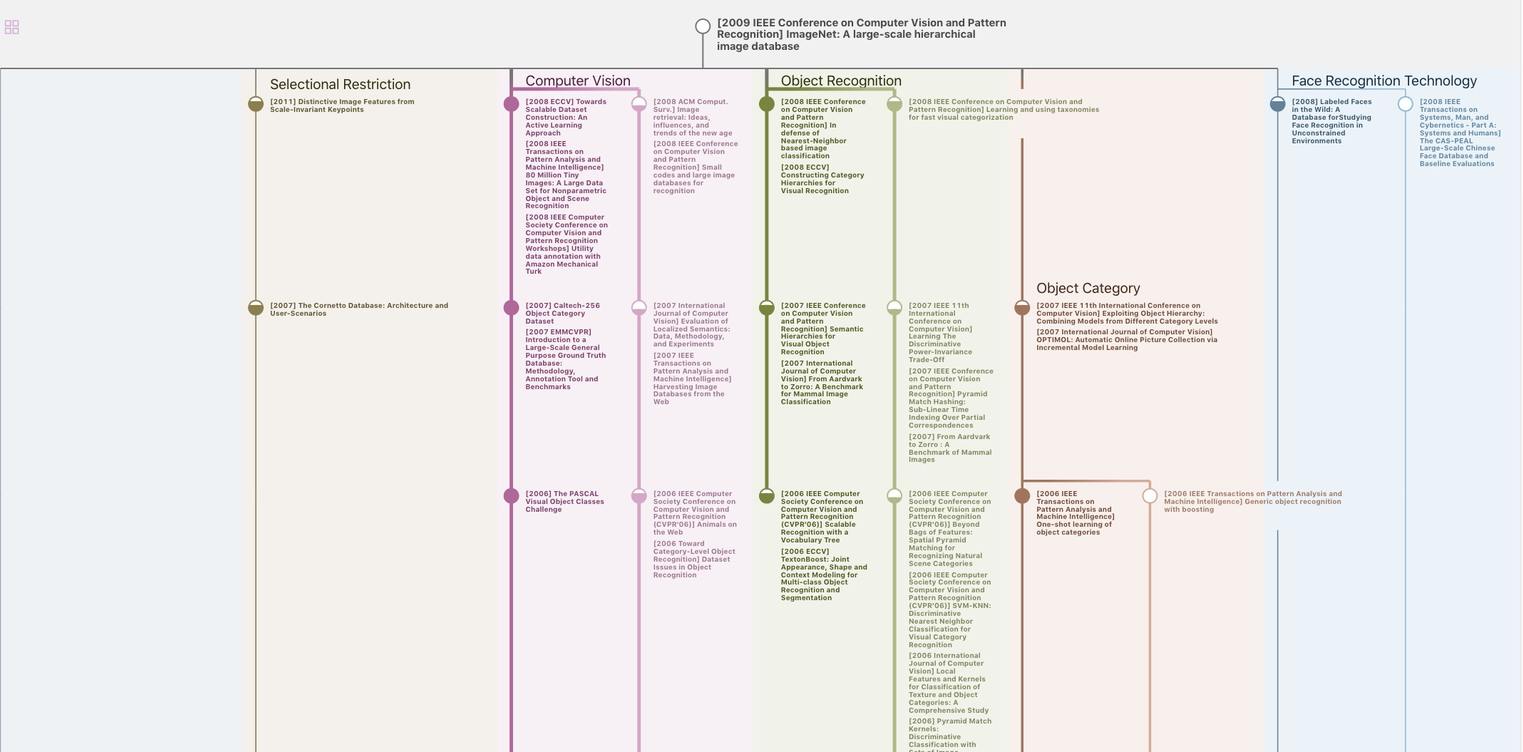
生成溯源树,研究论文发展脉络
Chat Paper
正在生成论文摘要