Calibration of Derivative Pricing Models: a Multi-Agent Reinforcement Learning Perspective
PROCEEDINGS OF THE 4TH ACM INTERNATIONAL CONFERENCE ON AI IN FINANCE, ICAIF 2023(2023)
摘要
One of the most fundamental questions in quantitative finance is the existence of continuous-time diffusion models that fit market prices of a given set of options. Traditionally, one employs a mix of intuition, theoretical and empirical analysis to find models that achieve exact or approximate fits. Our contribution is to show how a suitable game theoretical formulation of this problem can help solve this question by leveraging existing developments in modern deep multi-agent reinforcement learning to search in the space of stochastic processes. Our experiments show that we are able to learn local volatility, as well as path-dependence required in the volatility process to minimize the price of a Bermudan option. Our algorithm can be seen as a particle method a la Guyon et HenryLabordere where particles, instead of being designed to ensure sigma(loc) (t, S-t)(2) = E[sigma(2)(t) |S-t], are learning RL-driven agents cooperating towards more general calibration targets.
更多查看译文
关键词
reinforcement learning,calibration,derivative pricing model
AI 理解论文
溯源树
样例
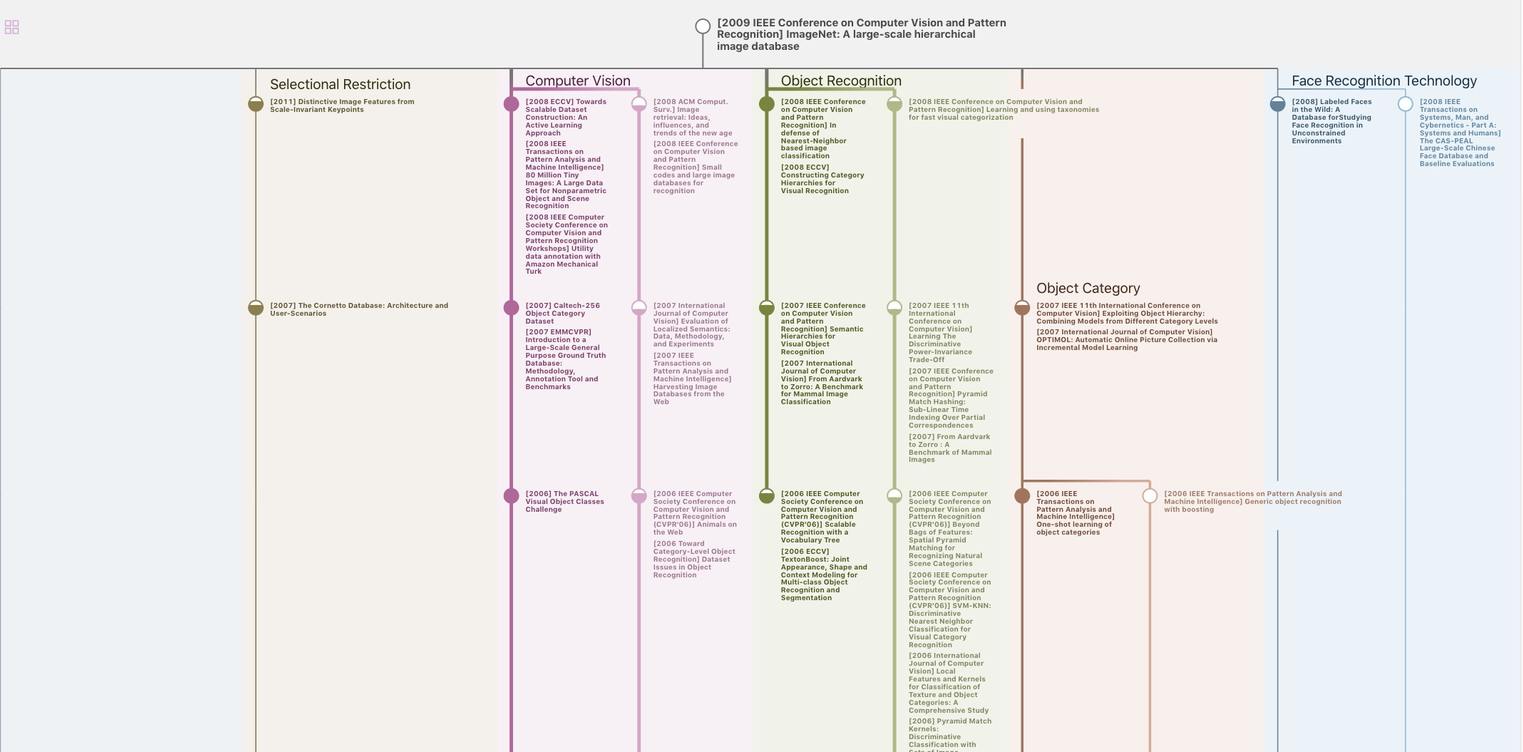
生成溯源树,研究论文发展脉络
Chat Paper
正在生成论文摘要