Sequential Recommendation with User Evolving Preference Decomposition
ANNUAL INTERNATIONAL ACM SIGIR CONFERENCE ON RESEARCH AND DEVELOPMENT IN INFORMATION RETRIEVAL IN THE ASIA PACIFIC REGION, SIGIR-AP 2023(2023)
摘要
Modeling user sequential behaviors has recently attracted increasing attention in the recommendation domain. Existing methods mostly assume coherent preference in the same sequence. However, user personalities are volatile and easily changed, and there can be multiple mixed preferences underlying user behaviors. To solve this problem, in this paper, we propose a novel sequential recommender model via decomposing and modeling user independent preferences. To achieve this goal, we highlight three practical challenges considering the inconsistent, evolving and uneven nature of the user behaviors. For overcoming these challenges in a unified framework, we introduce a reinforcement learning module to simulate the evolution of user preference. More specifically, the action aims to allocate each item into a sub-sequence or create a new one according to how the previous items are decomposed as well as the time interval between successive behaviors. The reward is associated with the final loss of the learning objective, aiming to generate sub-sequences which can better fit the training data. We conduct extensive experiments based on eight real-world datasets across different domains. Comparing with the state-of-the-art methods, empirical studies manifest that our model can on average improve the performance by about 9.68%, 12.4%, 8.56% and 7.13% on the metrics of Precision, Recall, NDCG and MRR, respectively.
更多查看译文
关键词
Sequential Recommendation,Reinforcement Learning,User Behavior Analysis
AI 理解论文
溯源树
样例
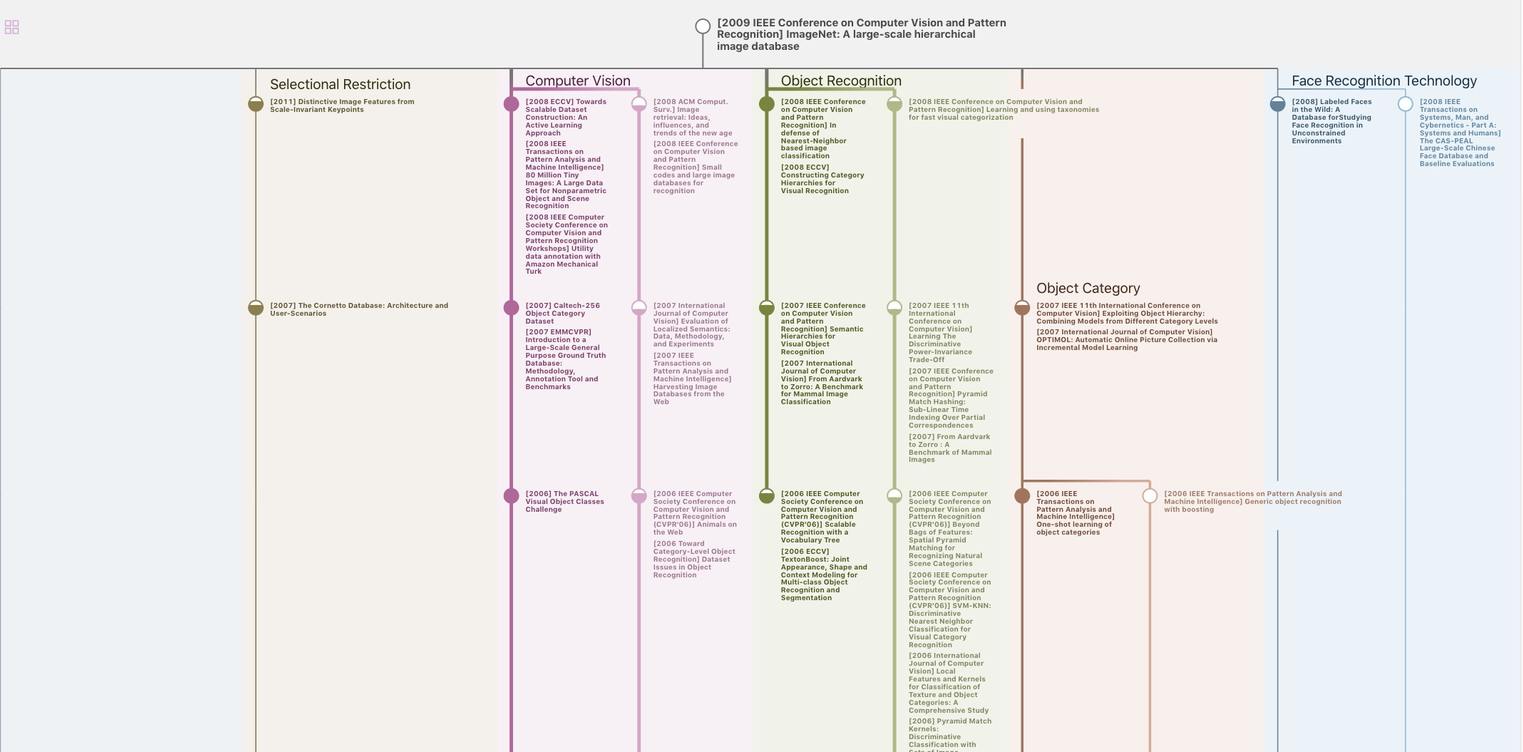
生成溯源树,研究论文发展脉络
Chat Paper
正在生成论文摘要