Adapting CLIP For Phrase Localization Without Further Training
arxiv(2022)
摘要
Supervised or weakly supervised methods for phrase localization (textual grounding) either rely on human annotations or some other supervised models, e.g., object detectors. Obtaining these annotations is labor-intensive and may be difficult to scale in practice. We propose to leverage recent advances in contrastive language-vision models, CLIP, pre-trained on image and caption pairs collected from the internet. In its original form, CLIP only outputs an image-level embedding without any spatial resolution. We adapt CLIP to generate high-resolution spatial feature maps. Importantly, we can extract feature maps from both ViT and ResNet CLIP model while maintaining the semantic properties of an image embedding. This provides a natural framework for phrase localization. Our method for phrase localization requires no human annotations or additional training. Extensive experiments show that our method outperforms existing no-training methods in zero-shot phrase localization, and in some cases, it even outperforms supervised methods. Code is available at https://github.com/pals-ttic/adapting-CLIP .
更多查看译文
关键词
phrase localization,clip
AI 理解论文
溯源树
样例
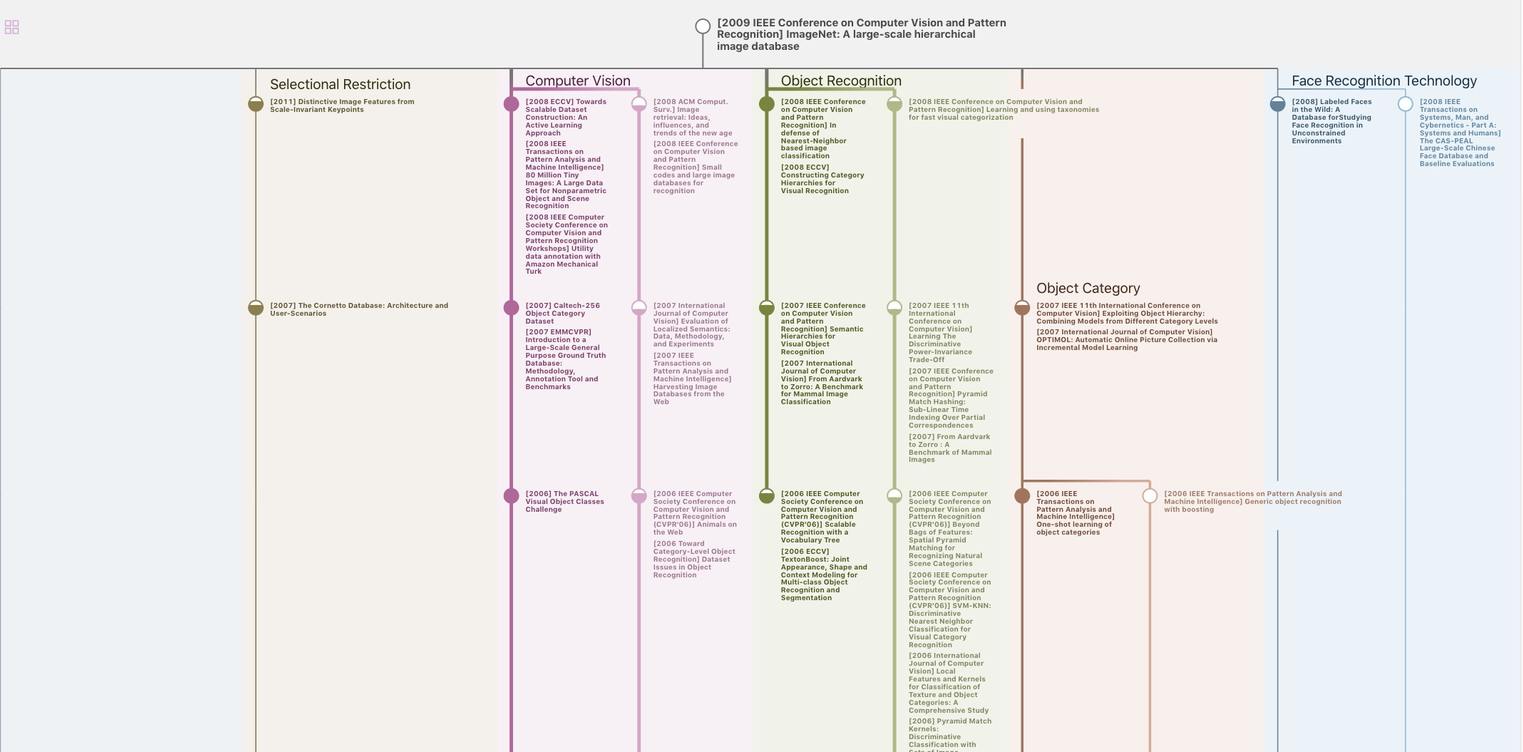
生成溯源树,研究论文发展脉络
Chat Paper
正在生成论文摘要