Pollen Grain Classification Based on Ensemble Transfer Learning on the Cretan Pollen Dataset
PLANTS-BASEL(2022)
摘要
Pollen identification is an important task for the botanical certification of honey. It is performed via thorough microscopic examination of the pollen present in honey; a process called melissopalynology. However, manual examination of the images is hard, time-consuming and subject to inter- and intra-observer variability. In this study, we investigated the applicability of deep learning models for the classification of pollen-grain images into 20 pollen types, based on the Cretan Pollen Dataset. In particular, we applied transfer and ensemble learning methods to achieve an accuracy of 97.5%, a sensitivity of 96.9%, a precision of 97%, an F1 score of 96.89% and an AUC of 0.9995. However, in a preliminary case study, when we applied the best-performing model on honey-based pollen-grain images, we found that it performed poorly; only 0.02 better than random guessing (i.e., an AUC of 0.52). This indicates that the model should be further fine-tuned on honey-based pollen-grain images to increase its effectiveness on such data.
更多查看译文
关键词
pollen grain, classification, honey certification, melissopalynology, deep learning, transfer learning, ensemble
AI 理解论文
溯源树
样例
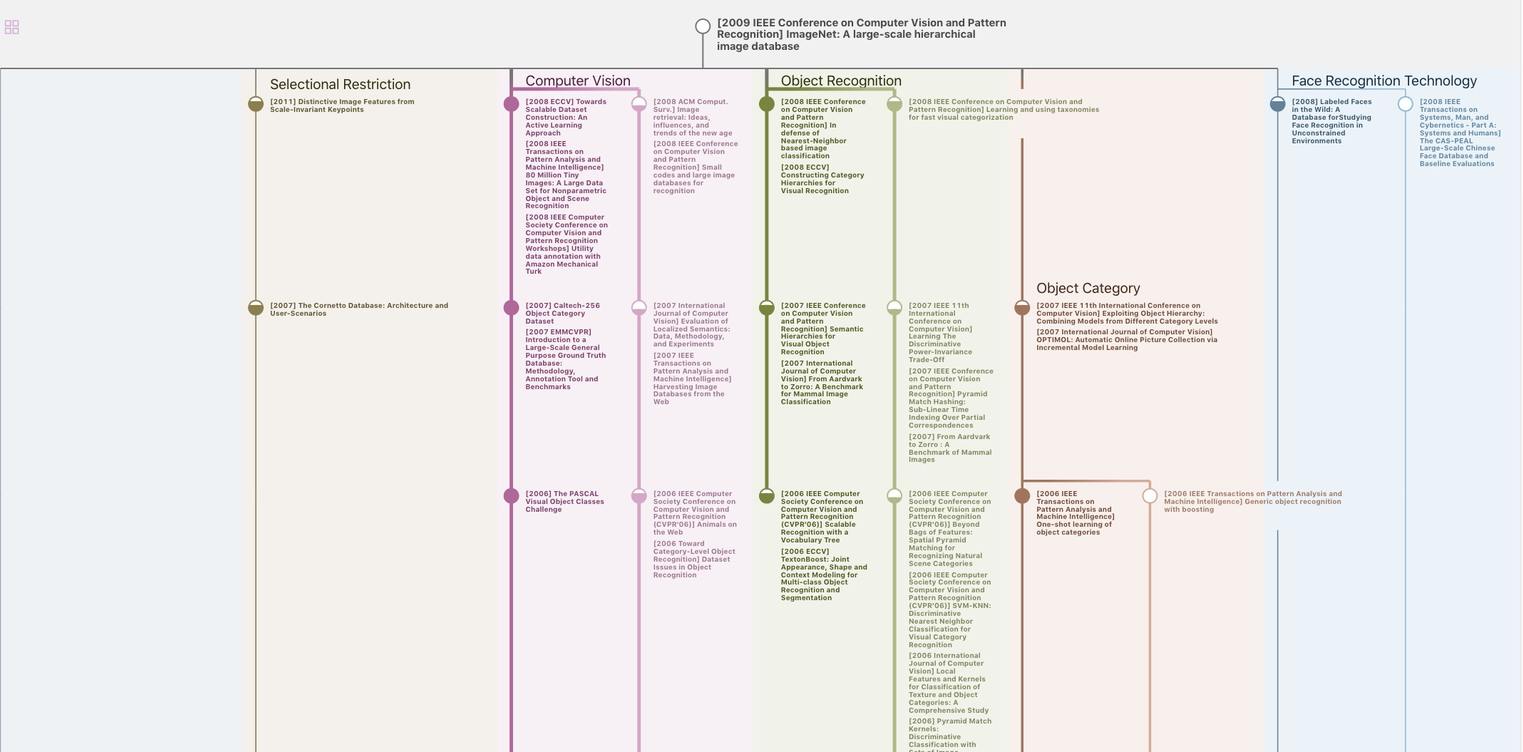
生成溯源树,研究论文发展脉络
Chat Paper
正在生成论文摘要