METRO: Efficient Denoising Pretraining of Large Scale Autoencoding Language Models with Model Generated Signals
arxiv(2022)
摘要
We present an efficient method of pretraining large-scale autoencoding language models using training signals generated by an auxiliary model. Originated in ELECTRA, this training strategy has demonstrated sample-efficiency to pretrain models at the scale of hundreds of millions of parameters. In this work, we conduct a comprehensive empirical study, and propose a recipe, namely "Model generated dEnoising TRaining Objective" (METRO), which incorporates some of the best modeling techniques developed recently to speed up, stabilize, and enhance pretrained language models without compromising model effectiveness. The resultant models, METRO-LM, consisting of up to 5.4 billion parameters, achieve new state-of-the-art on the GLUE, SuperGLUE, and SQuAD benchmarks. More importantly, METRO-LM are efficient in that they often outperform previous large models with significantly smaller model sizes and lower pretraining cost.
更多查看译文
关键词
efficient denoising pretraining,large scale autoencoding
AI 理解论文
溯源树
样例
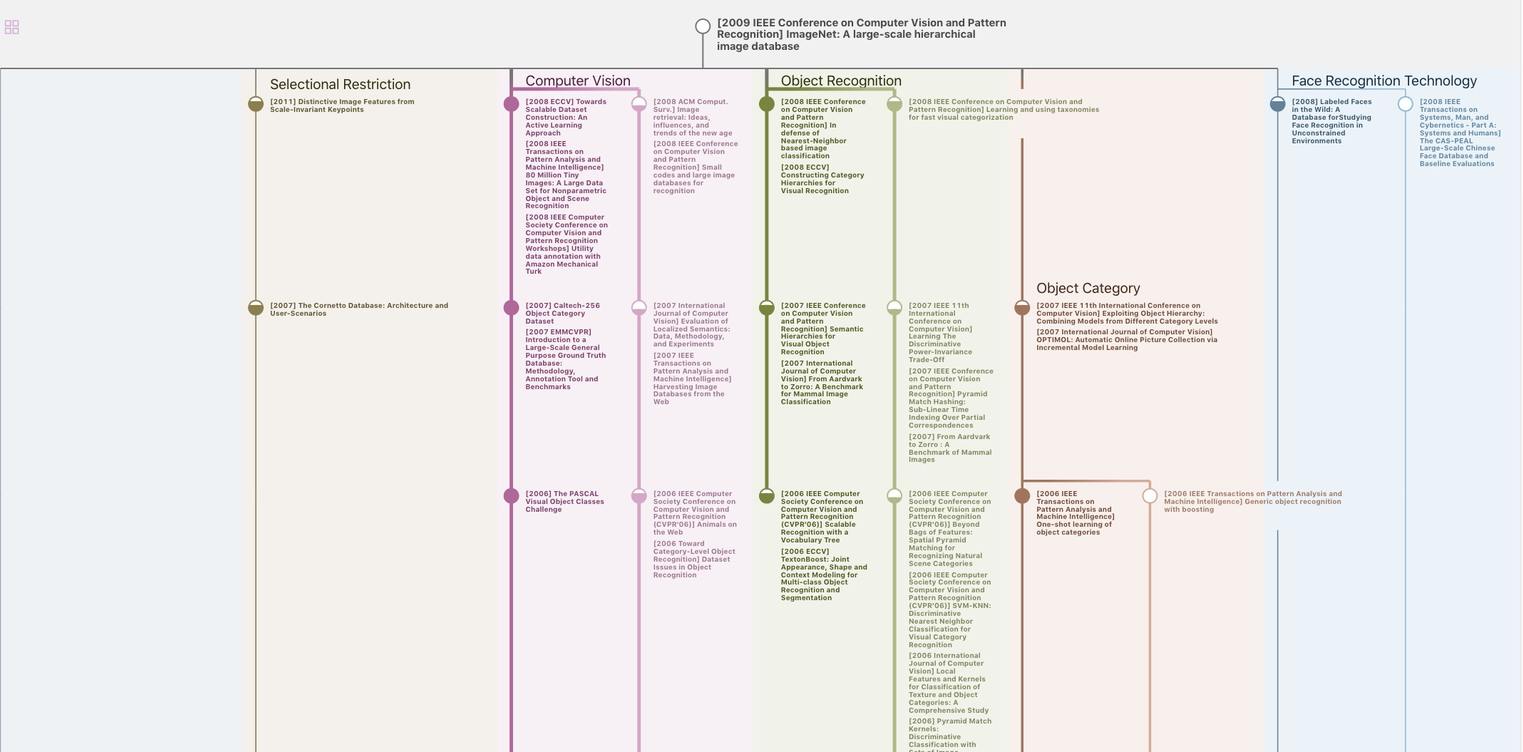
生成溯源树,研究论文发展脉络
Chat Paper
正在生成论文摘要