Parallel Synthesis for Autoregressive Speech Generation
arxiv(2023)
摘要
Autoregressive neural vocoders have achieved outstanding performance in speech synthesis tasks such as text-to-speech and voice conversion. An autoregressive vocoder predicts a sample at some time step conditioned on those at previous time steps. Though it synthesizes natural human speech, the iterative generation inevitably makes the synthesis time proportional to the utterance length, leading to low efficiency. Many works were dedicated to generating the whole speech sequence in parallel and proposed GAN-based, flow-based, and score-based vocoders. This paper proposed a new thought for the autoregressive generation. Instead of iteratively predicting samples in a time sequence, the proposed model performs frequency-wise autoregressive generation (FAR) and bit-wise autoregressive generation (BAR) to synthesize speech. In FAR, a speech utterance is split into frequency subbands, and a subband is generated conditioned on the previously generated one. Similarly, in BAR, an 8-bit quantized signal is generated iteratively from the first bit. By redesigning the autoregressive method to compute in domains other than the time domain, the number of iterations in the proposed model is no longer proportional to the utterance length but to the number of subbands/bits, significantly increasing inference efficiency. Besides, a post-filter is employed to sample signals from output posteriors; its training objective is designed based on the characteristics of the proposed methods. Experimental results show that the proposed model can synthesize speech faster than real-time without GPU acceleration. Compared with baseline vocoders, the proposed model achieves better MUSHRA results and shows good generalization ability for unseen speakers and 44 kHz speech.
更多查看译文
关键词
Vocoder,neural network,neural speech synthesis,autoregressive model
AI 理解论文
溯源树
样例
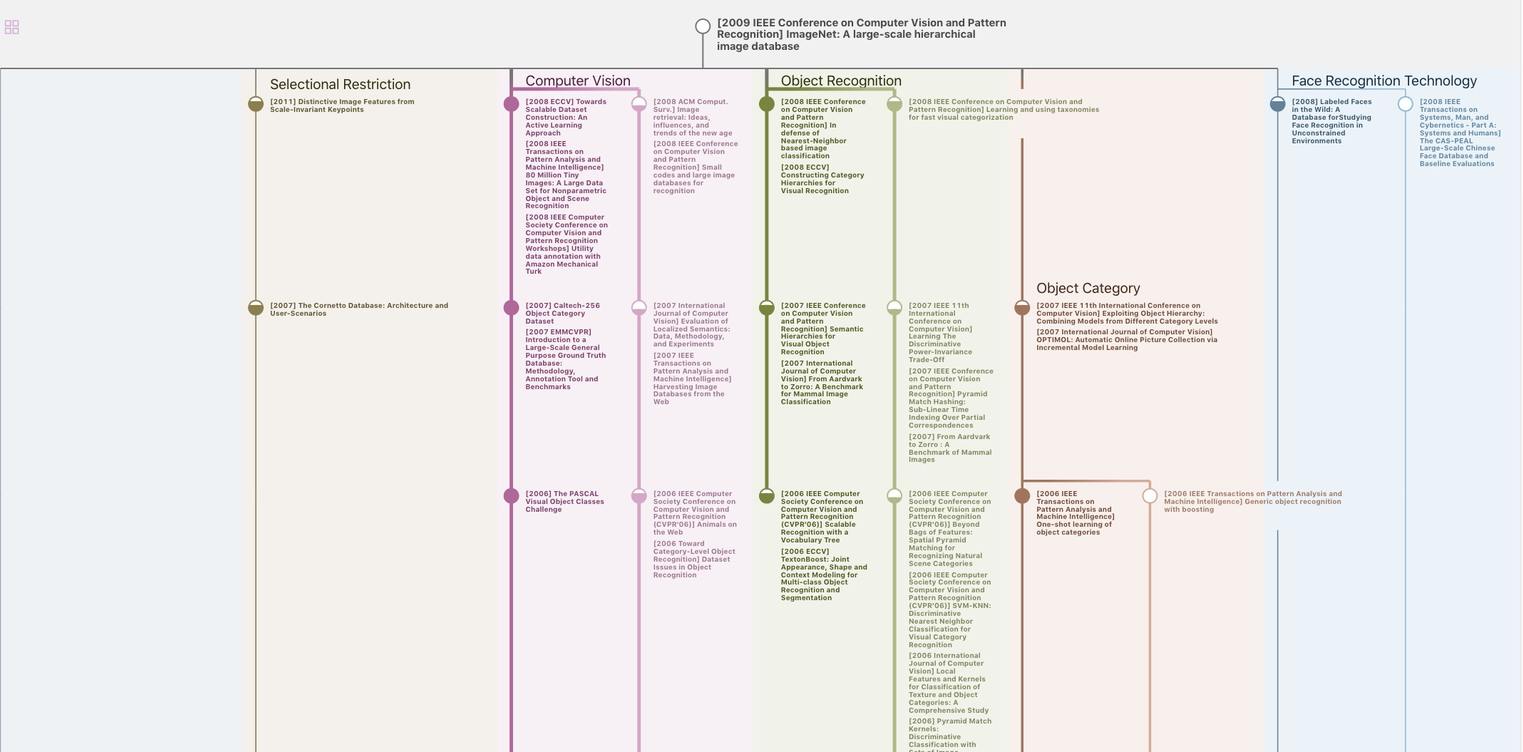
生成溯源树,研究论文发展脉络
Chat Paper
正在生成论文摘要