KING: Generating Safety-Critical Driving Scenarios for Robust Imitation via Kinematics Gradients.
European Conference on Computer Vision(2022)
摘要
Simulators offer the possibility of safe, low-cost development of self-driving systems. However, current driving simulators exhibit na\"ive behavior models for background traffic. Hand-tuned scenarios are typically added during simulation to induce safety-critical situations. An alternative approach is to adversarially perturb the background traffic trajectories. In this paper, we study this approach to safety-critical driving scenario generation using the CARLA simulator. We use a kinematic bicycle model as a proxy to the simulator's true dynamics and observe that gradients through this proxy model are sufficient for optimizing the background traffic trajectories. Based on this finding, we propose KING, which generates safety-critical driving scenarios with a 20% higher success rate than black-box optimization. By solving the scenarios generated by KING using a privileged rule-based expert algorithm, we obtain training data for an imitation learning policy. After fine-tuning on this new data, we show that the policy becomes better at avoiding collisions. Importantly, our generated data leads to reduced collisions on both held-out scenarios generated via KING as well as traditional hand-crafted scenarios, demonstrating improved robustness.
更多查看译文
关键词
robust imitation,kinematics gradients,safety-critical
AI 理解论文
溯源树
样例
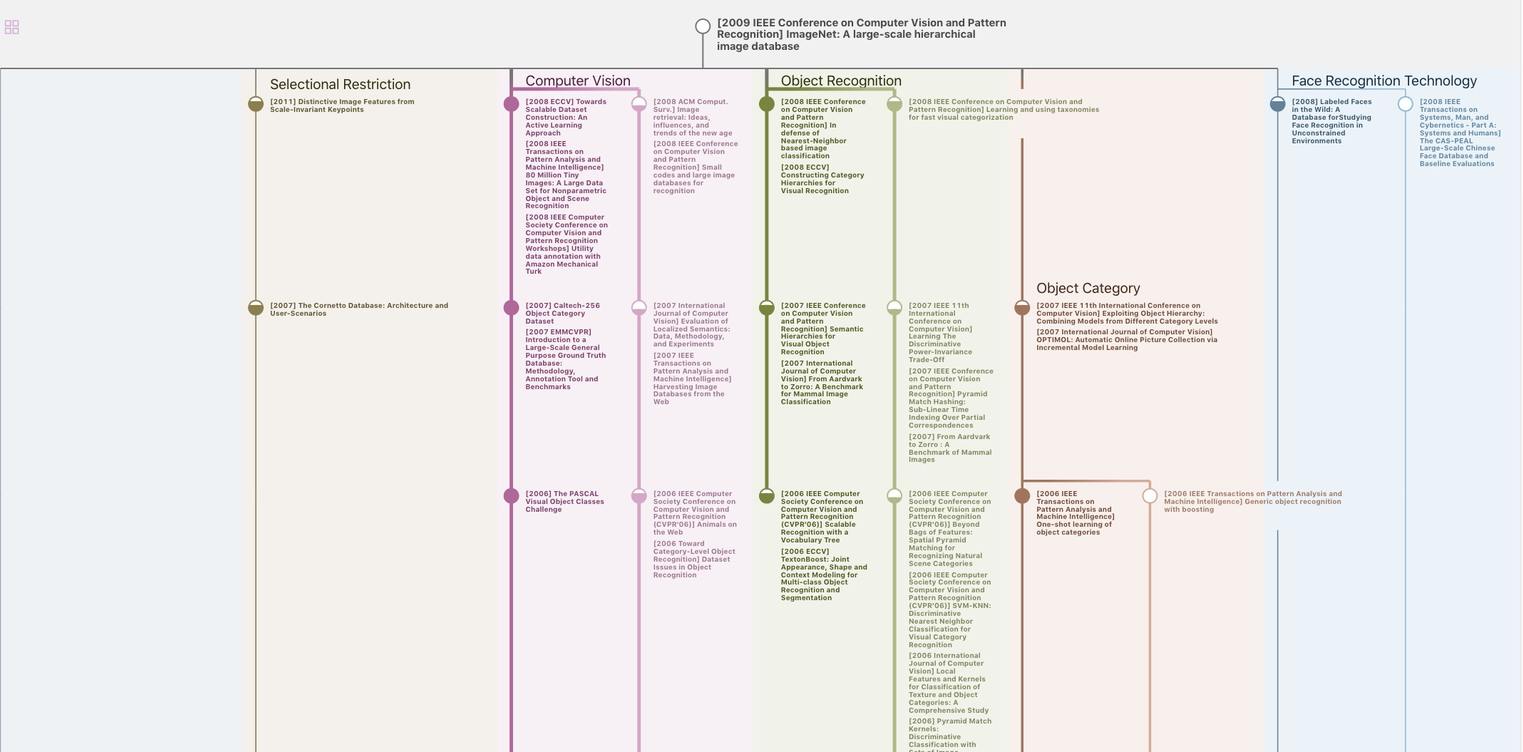
生成溯源树,研究论文发展脉络
Chat Paper
正在生成论文摘要