Sparsifying Transformer Models with Trainable Representation Pooling.
Annual Meeting of the Association for Computational Linguistics(2022)
摘要
We propose a novel method to sparsify attention in the Transformer model by learning to select the most-informative token representations during the training process, thus focusing on the task-specific parts of an input. A reduction of quadratic time and memory complexity to sublinear was achieved due to a robust trainable top-
更多查看译文
AI 理解论文
溯源树
样例
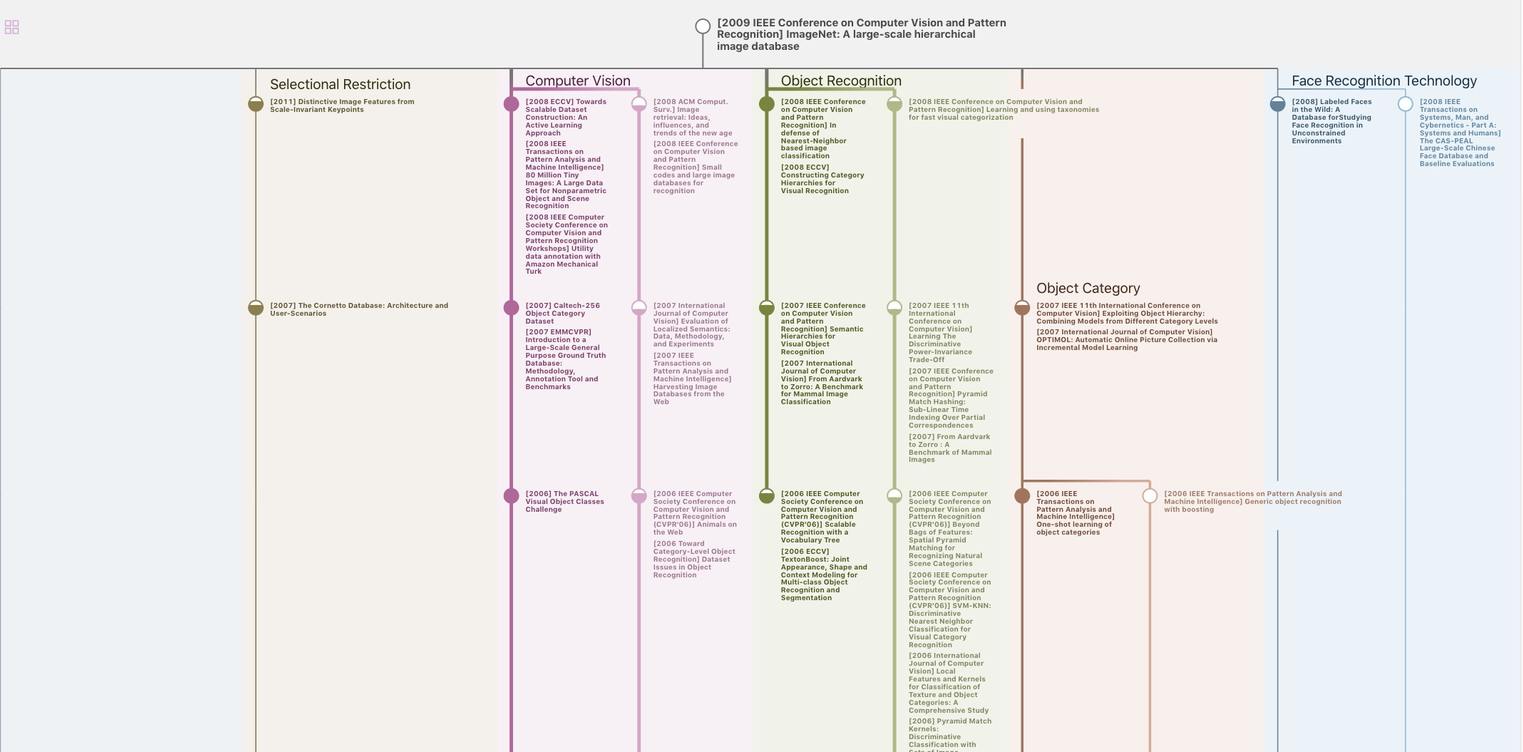
生成溯源树,研究论文发展脉络
Chat Paper
正在生成论文摘要