Planning for Potential: Efficient Safe Reinforcement Learning
Machine learning(2022)
摘要
Deep reinforcement learning (DRL) has shown remarkable success in artificial domains and in some real-world applications. However, substantial challenges remain such as learning efficiently under safety constraints. Adherence to safety constraints is a hard requirement in many high-impact application domains such as healthcare and finance. These constraints are preferably represented symbolically to ensure clear semantics at a suitable level of abstraction. Existing approaches to safe DRL assume that being unsafe leads to low rewards. We show that this is a special case of symbolically constrained RL and analyze a generic setting in which total reward and being safe may or may not be correlated. We analyze the impact of symbolic constraints and identify a connection between expected future reward and distance towards a goal in an automaton representation of the constraints. We use this connection in an algorithm for learning complex behaviors safely and efficiently. This algorithm relies on symbolic reasoning over safety constraints to improve the efficiency of a subsymbolic learner with a symbolically obtained measure of progress. We measure sample efficiency on a grid world and a conversational product recommender with real-world constraints. The so-called Planning for Potential algorithm converges quickly and significantly outperforms all baselines. Specifically, we find that symbolic reasoning is necessary for safety during and after learning and can be effectively used to guide a neural learner towards promising areas of the solution space. We conclude that RL can be applied both safely and efficiently when combined with symbolic reasoning.
更多查看译文
关键词
Reinforcement learning,Safety constraints,Symbolic planning,Reward shaping
AI 理解论文
溯源树
样例
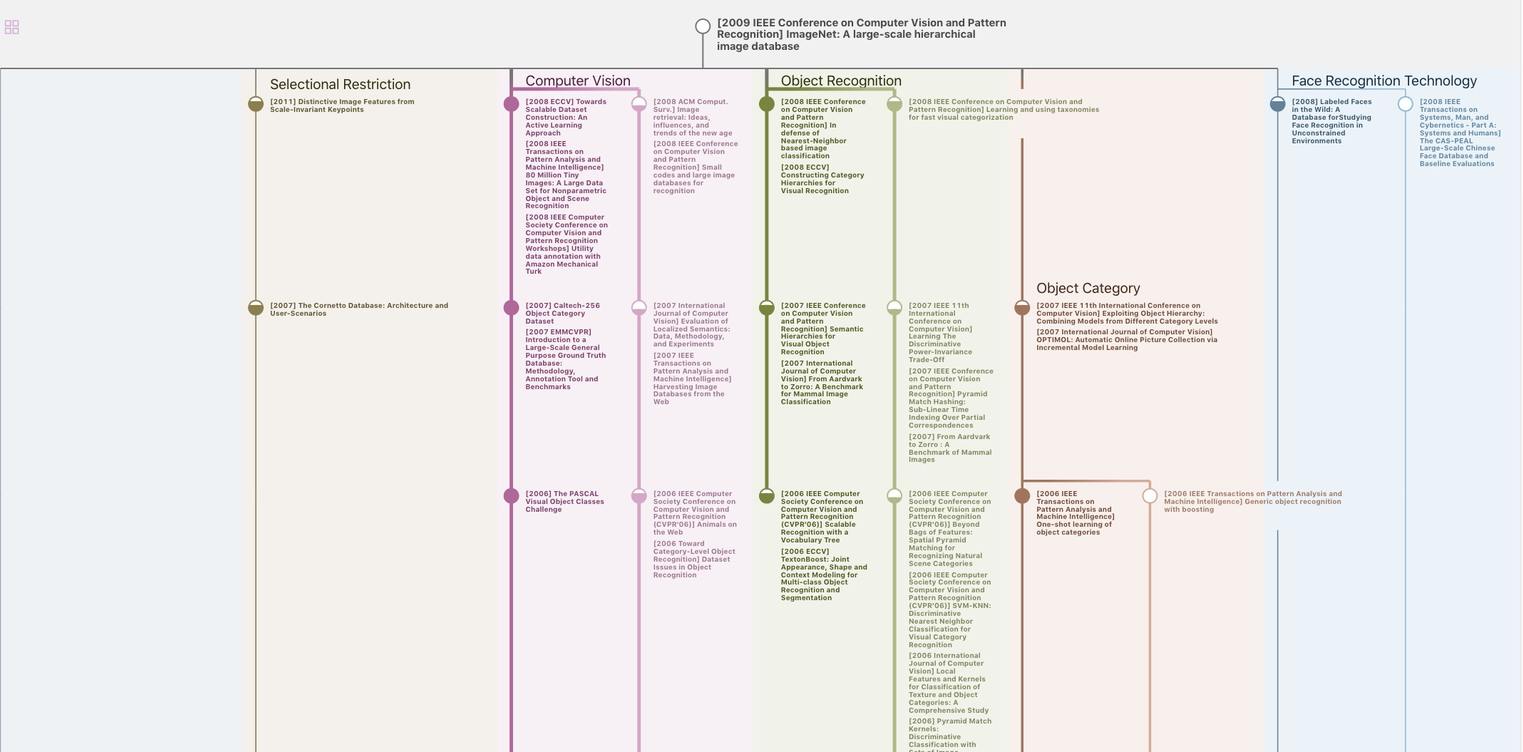
生成溯源树,研究论文发展脉络
Chat Paper
正在生成论文摘要