MINER: Multi-Interest Matching Network for News Recommendation
FINDINGS OF THE ASSOCIATION FOR COMPUTATIONAL LINGUISTICS (ACL 2022)(2022)
摘要
Personalized news recommendation is an essential technique to help users find interested news. Accurately matching user's interests and candidate news is the key to news recommendation. Most existing methods learn a single user embedding from user's historical behaviors to represent the reading interest. However, user interest is usually diverse and may not be adequately modeled by a single user embedding. In this paper, we propose a poly attention scheme to learn multiple interest vectors for each user, which encodes the different aspects of user interest. We further propose a disagreement regularization to make the learned interests vectors more diverse. Moreover, we design a category-aware attention weighting strategy that incorporates the news category information as explicit interest signals into the attention mechanism. Extensive experiments on the MIND news recommendation benchmark demonstrate that our approach significantly outperforms existing state-of-the-art methods.
更多查看译文
关键词
news recommendation,miner,network,multi-interest
AI 理解论文
溯源树
样例
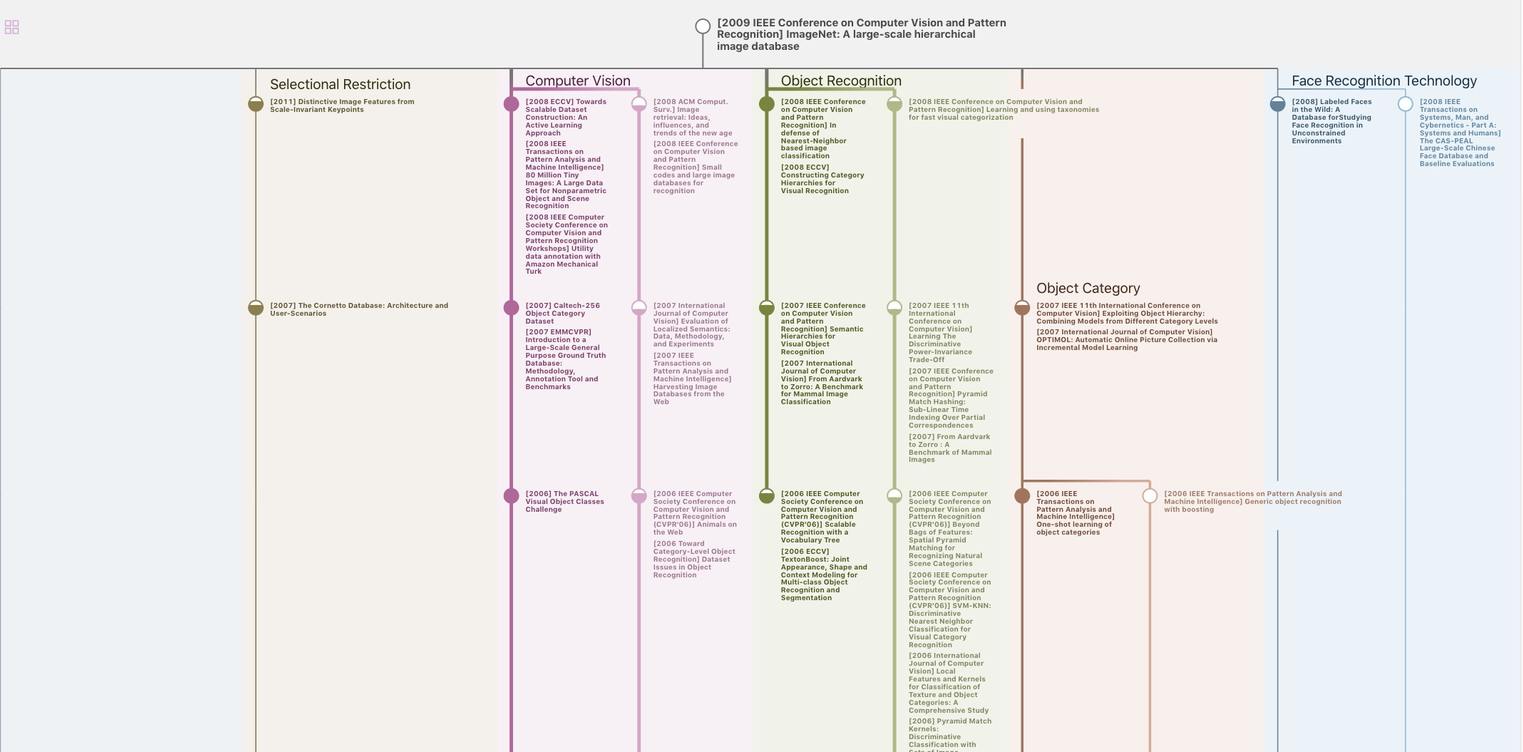
生成溯源树,研究论文发展脉络
Chat Paper
正在生成论文摘要