Vector-Quantized Input-Contextualized Soft Prompts for Natural Language Understanding
emnlp 2022(2022)
摘要
Prompt Tuning has been largely successful as a parameter-efficient method of conditioning large-scale pre-trained language models to perform downstream tasks. Thus far, soft prompt tuning learns a fixed set of task-specific continuous vectors, i.e., soft tokens that remain static across the task samples. A fixed prompt, however, may not generalize well to the diverse kinds of inputs the task comprises. In order to address this, we propose Vector-quantized Input-contextualized Prompts (VIP) as an extension to the soft prompt tuning framework. VIP particularly focuses on two aspects -- contextual prompts that learns input-specific contextualization of the soft prompt tokens through a small-scale sentence encoder and quantized prompts that maps the contextualized prompts to a set of learnable codebook vectors through a Vector quantization network. On various language understanding tasks like SuperGLUE, QA, Relation classification, NER and NLI, VIP outperforms the soft prompt tuning (PT) baseline by an average margin of 1.19%. Further, our generalization studies show that VIP learns more robust prompt representations, surpassing PT by a margin of 0.6% - 5.3% on Out-of-domain QA and NLI tasks respectively, and by 0.75% on Multi-Task setup over 4 tasks spanning across 12 domains.
更多查看译文
关键词
soft prompts,natural language,understanding,vector-quantized,input-contextualized
AI 理解论文
溯源树
样例
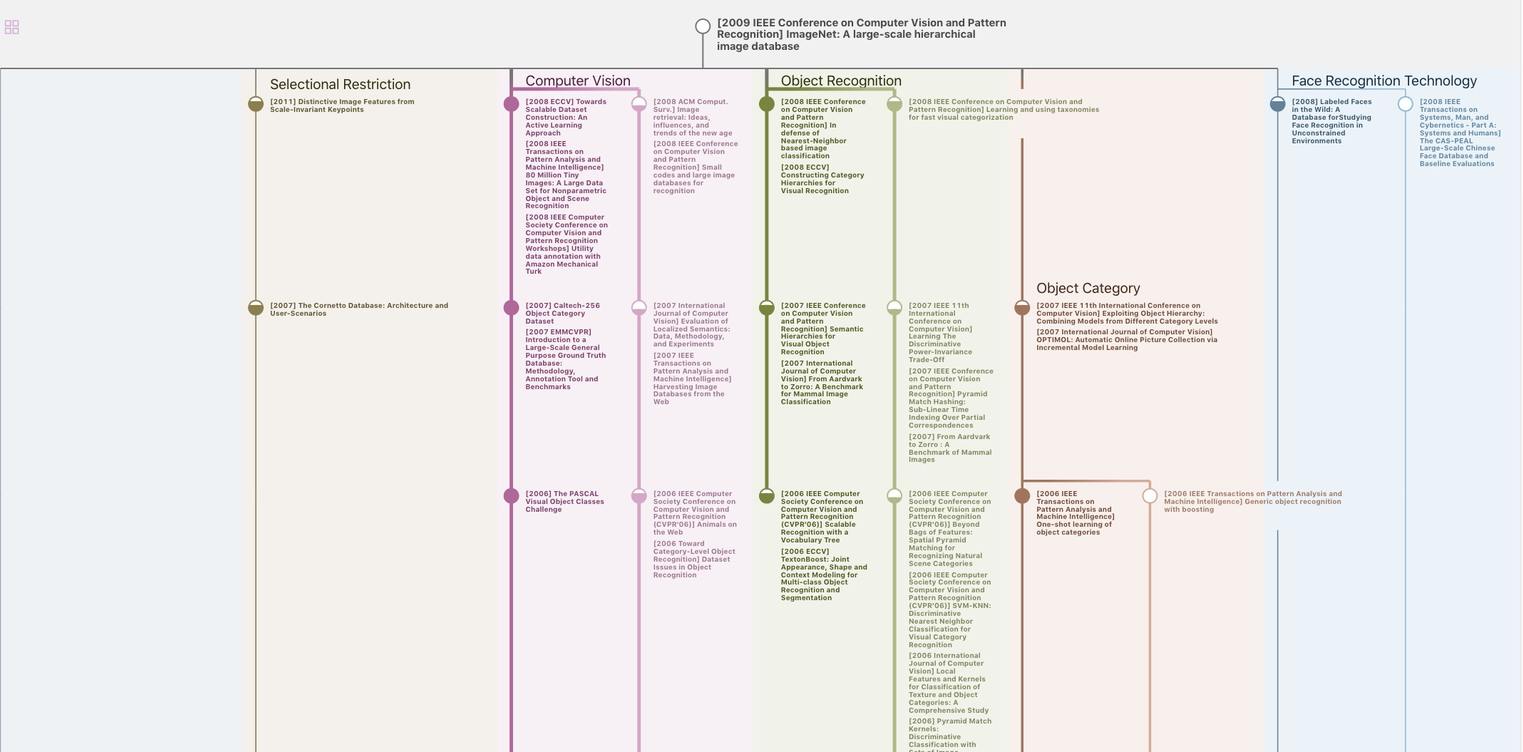
生成溯源树,研究论文发展脉络
Chat Paper
正在生成论文摘要