Learning Modality-Invariant Features by Cross-Modality Adversarial Network for Visual Question Answering
WEB AND BIG DATA, APWEB-WAIM 2021, PT I(2021)
摘要
Visual Question Answering (VQA) is a typical multimodal task with significant development prospect on web application. In order to answer the question based on the corresponding image, a VQA model needs to utilize the information from different modality efficiently. Although the multimodal fusion methods such as attention mechanism make significant contribution for VQA, these methods try to co-learn the multimodal features directly, ignoring the large gap between different modality and thus poor aligning the semantic. In this paper, we propose a Cross-Modality Adversarial Network (CMAN) to address this limitation. Our method combines cross-modality adversarial learning with modality-invariant attention learning aiming to learn the modality-invariant features for better semantic alignment and higher answer prediction accuracy. The accuracy of model achieves 70.81% on the test-dev split on the VQA-v2 dataset. Our results also show that the model narrows the gap between different modalities effectively and improves the alignment performance of the multimodal information.
更多查看译文
关键词
Visual question answering, Domain adaptation, Modality-invariant co-learning
AI 理解论文
溯源树
样例
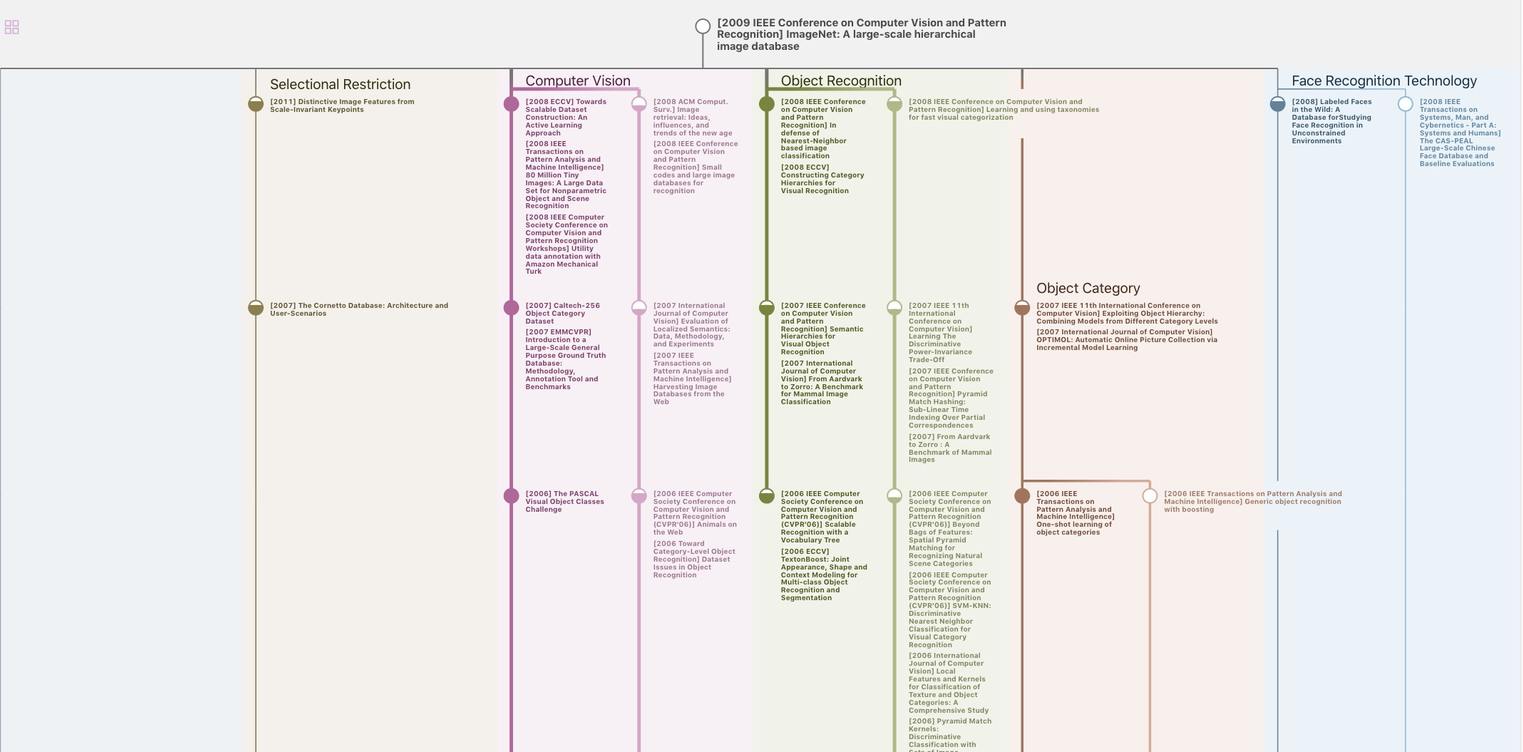
生成溯源树,研究论文发展脉络
Chat Paper
正在生成论文摘要