Learning Insurance Benefit Rules from Policy Texts with Small Labeled Data.
World Congress on Medical and Health (Medical) Informatics (MedInfo)(2022)
摘要
To protect vital health program funds from being paid out on services that are wasteful and inconsistent with medical practices, government healthcare insurance programs need to validate the integrity of claims submitted by providers for reimbursement. However, due the complexity of healthcare billing policies and the lack of coded rules, maintaining "integrity" is a labor-intensive task, often narrow-scope and expensive. We propose an approach that combines deep learning and an ontology to support the extraction of actionable knowledge on benefit rules from regulatory healthcare policy text. We demonstrate its feasibility even in the presence of small ground truth labeled data provided by policy investigators. Leveraging deep learning and rich ontological information enables the system to learn from human corrections and capture better benefit rules from policy text, beyond just using a deterministic approach based on pre-defined textual and semantic pattterns.
更多查看译文
关键词
deep learning,health policy,ontology
AI 理解论文
溯源树
样例
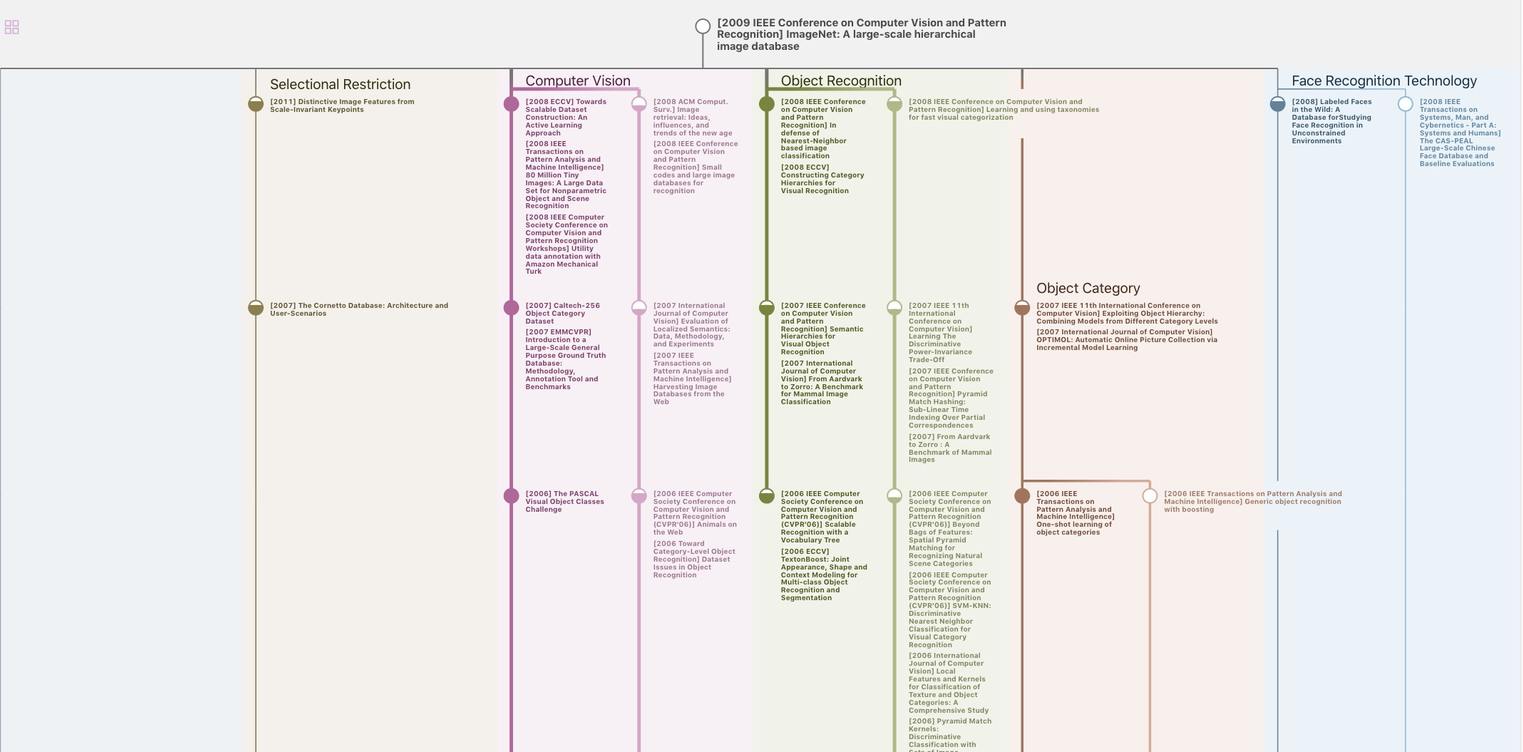
生成溯源树,研究论文发展脉络
Chat Paper
正在生成论文摘要