SAQAM: Spatial Audio Quality Assessment Metric
Conference of the International Speech Communication Association (INTERSPEECH)(2022)
摘要
Audio quality assessment is critical for assessing the perceptual realism of sounds. However, the time and expense of obtaining ''gold standard'' human judgments limit the availability of such data. For AR&VR, good perceived sound quality and localizability of sources are among the key elements to ensure complete immersion of the user. Our work introduces SAQAM which uses a multi-task learning framework to assess listening quality (LQ) and spatialization quality (SQ) between any given pair of binaural signals without using any subjective data. We model LQ by training on a simulated dataset of triplet human judgments, and SQ by utilizing activation-level distances from networks trained for direction of arrival (DOA) estimation. We show that SAQAM correlates well with human responses across four diverse datasets. Since it is a deep network, the metric is differentiable, making it suitable as a loss function for other tasks. For example, simply replacing an existing loss with our metric yields improvement in a speech-enhancement network.
更多查看译文
关键词
audio,quality
AI 理解论文
溯源树
样例
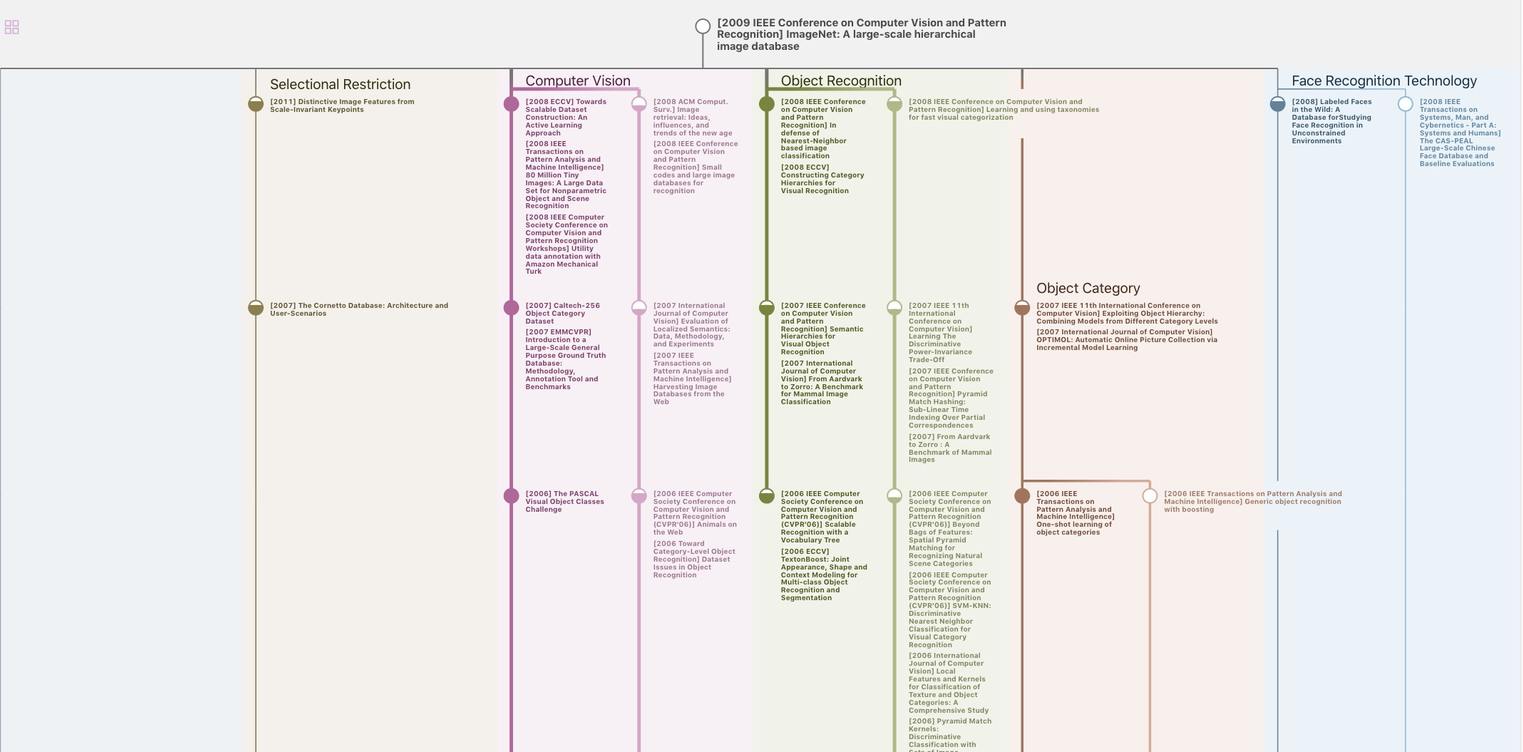
生成溯源树,研究论文发展脉络
Chat Paper
正在生成论文摘要