Surrogate modeling for neutron diffusion problems based on conservative physics-informed neural networks with boundary conditions enforcement
ANNALS OF NUCLEAR ENERGY(2022)
摘要
Application of physics-informed neural network(PINN) on neutron diffusion equation, which is of great engineering significance for reactor physics field, has not received much attention yet. Meanwhile, the non-smoothness of solution for neutron diffusion equation brings difficulties for PINN's application. Therefore, we introduce the conservative PINN(cPINN) which develops PINN for each sub-domain and considers additional conservation law along the sub-domains' interfaces, to solve heterogeneous neutron diffusion problems. Specifically, we develop PINNs on each sub-domain which has same material property and set equality constraints for neutron flux and neutron current on adjacent sub-domains. Furthermore, we propose a neural network constructing method to ensure that PINN/cPINN predictions strictly conform to three types of boundary conditions(BCs) involved in neutron diffusion problems. The results of numerical examples demonstrate that cPINN can solve heterogeneous neutron diffusion problems with non-smooth solutions and the proposed BC-imposed method can help to improve the cPINN performance on complex heterogeneous problems. (C) 2022 Published by Elsevier Ltd.
更多查看译文
关键词
Neutron diffusion equation,Conservative physics-informed neural network,Deep Learning,Boundary conditions enforcement,Heterogeneous problems
AI 理解论文
溯源树
样例
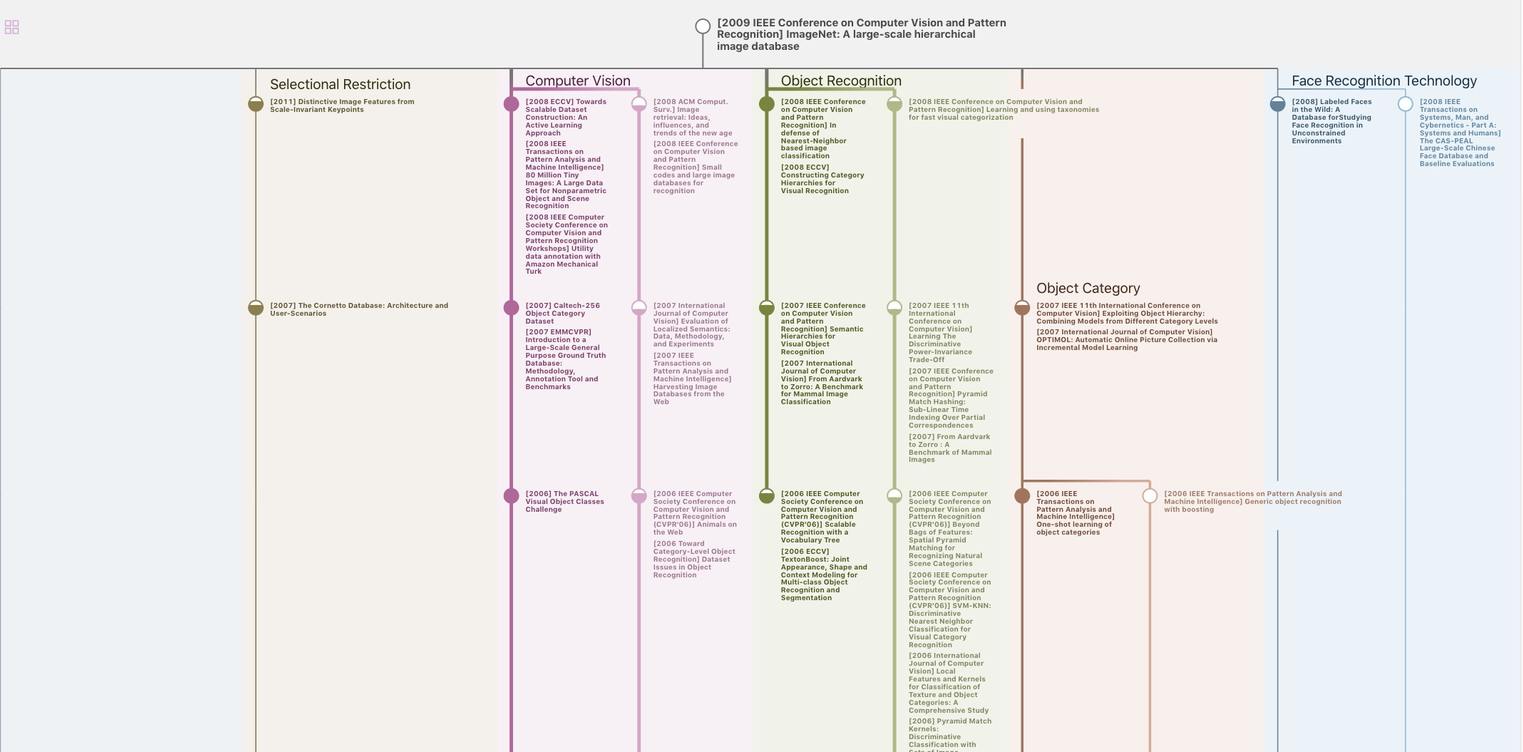
生成溯源树,研究论文发展脉络
Chat Paper
正在生成论文摘要