Generative Adversarial Network for Car Following Trajectory Generation and Anomaly Detection
Journal of intelligent transportation systems(2024)
摘要
Car-following trajectory generation and anomaly detection are critical functions in the sensing module of an automated vehicle. However, developing models that capture realistic trajectory data distribution and detect anomalous driving behaviors could be challenging. This paper proposes ‘TrajGAN’, an unsupervised learning approach based on the Generative Adversarial Network (GAN) to exploit vehicle car following trajectory data for generation and anomaly detection. The proposed TrajGAN consists of two modules, an encoder-decoder Long Short-Term Memory (LSTM)-based generator and an LSTM-multilayer perceptron (MLP) based discriminator, whose former component is used to generate vehicular car following trajectories and the latter one is for trajectory anomaly detection. By letting these two modules game with each other in training, we can simultaneously achieve robust trajectory generators and anomaly detectors. Trained with the Next Generation Simulation (NGSIM) dataset, TrajGAN can generate realistic trajectories with a similar distribution of training data and identify a manifold of anomalous trajectories based on an anomaly scoring scheme. Simulation results indicate that the proposed approach is efficient in reproducing artificial trajectories and identifying anomalous driving behaviors.
更多查看译文
关键词
anomaly detection,car,following,GAN,LSTM,trajectory generation
AI 理解论文
溯源树
样例
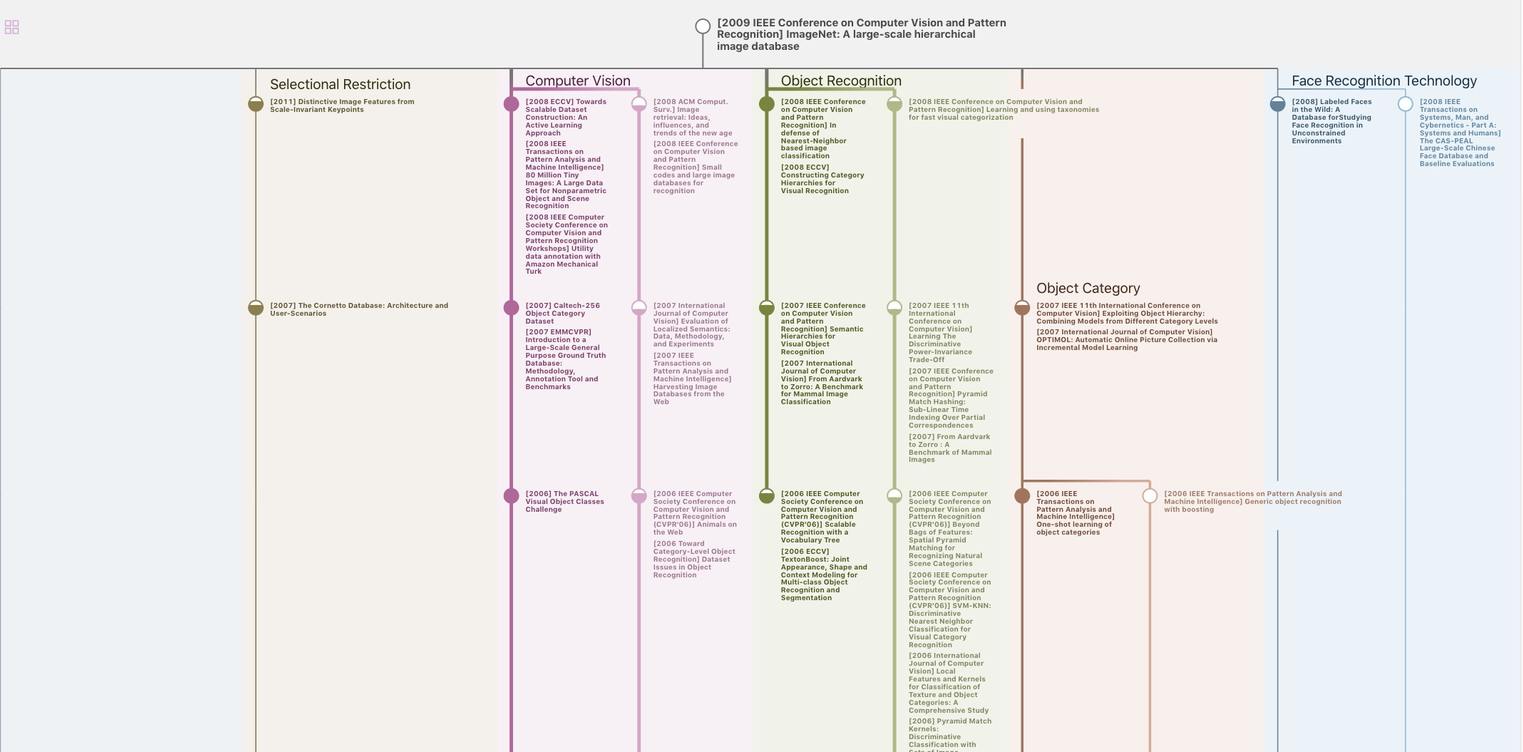
生成溯源树,研究论文发展脉络
Chat Paper
正在生成论文摘要