Dynamic gradient reactivation for backward compatible person re-identification
PATTERN RECOGNITION(2024)
摘要
We study the backward compatible problem for person re-identification (Re-ID), which aims to constrain the features of an updated new model to be comparable with the existing features from the old model in galleries. Most of the existing works adopt distillation-based methods, which focus on pushing new features to imitate the distribution of the old ones. However, the distillation-based methods are intrinsically sub-optimal since it forces the new feature space to imitate the inferior old feature space. To address this issue, we propose the Ranking-based Backward Compatible Learning (RBCL), which directly optimizes the ranking metric between new features and old features. Different from previous methods, RBCL only pushes the new features to find best-ranking positions in the old feature space instead of strictly alignment, and is in line with the ultimate goal of backward retrieval. However, the sharp sigmoid function used to make the ranking metric differentiable also incurs the gradient vanish issue, therefore stems the ranking refinement during the later period of training. To address this issue, we propose the Dynamic Gradient Reactivation (DGR), which can reactivate the suppressed gradients by adding dynamically computed constants during the forward step. To further help target the best -ranking positions, we include the Neighbor Context Agents (NCAs) to approximate the entire old feature space during training. Unlike previous works that mainly test on the in-domain settings, we make the early attempt to introduce the cross-domain settings (including both supervised and unsupervised) for the backward compatible person Re-ID task, which are more challenging yet meaningful. The experimental results on all five settings show that the proposed RBCL outperforms previous state-of-the-art methods by large margins.
更多查看译文
关键词
Person re-identification,Backward compatible training,Deep learning
AI 理解论文
溯源树
样例
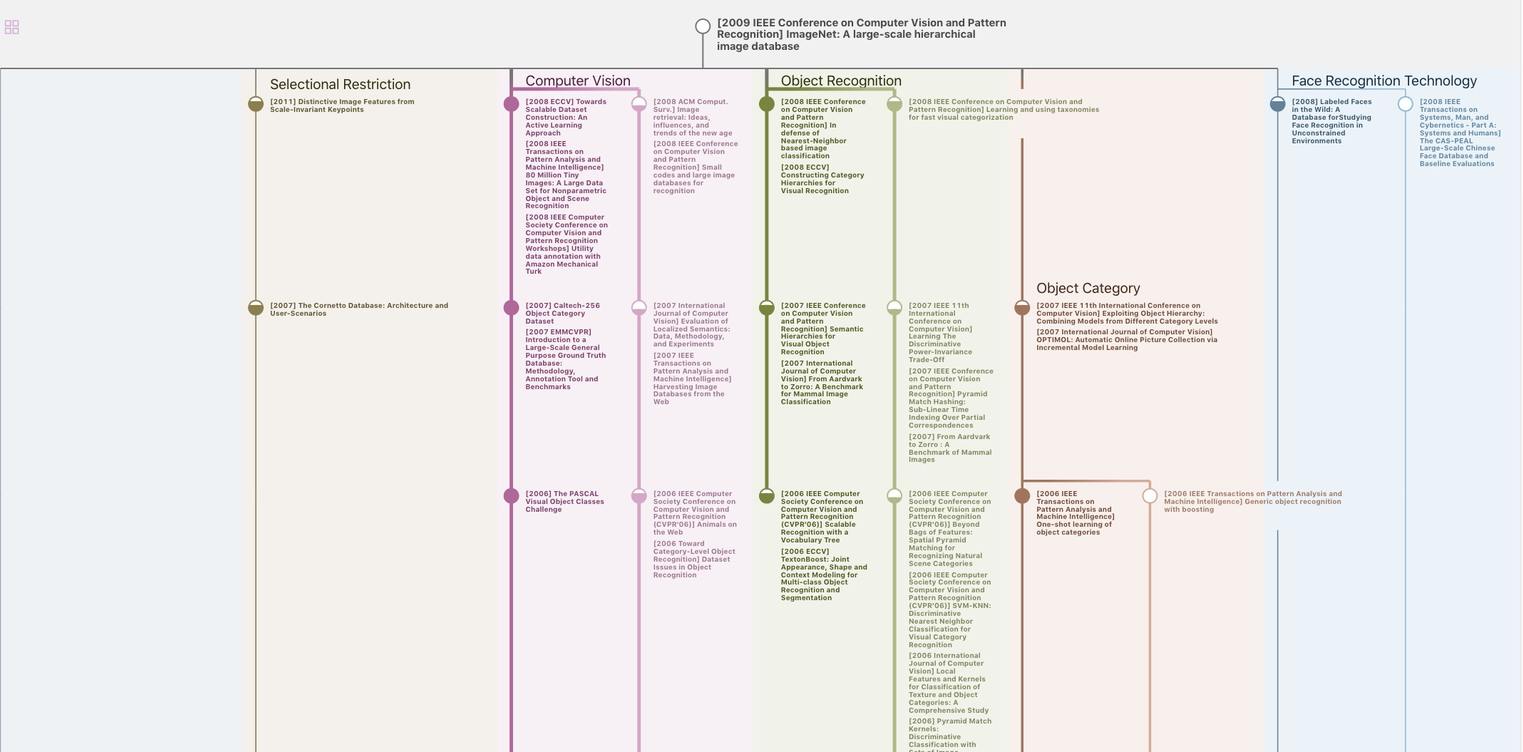
生成溯源树,研究论文发展脉络
Chat Paper
正在生成论文摘要