An Efficient CNN for Radiogenomic Classification of Low-Grade Gliomas on MRI in a Small Dataset
WIRELESS COMMUNICATIONS & MOBILE COMPUTING(2022)
摘要
Gliomas, often known as low-grade gliomas, are malignant brain tumors. Codeletion of chromosomal arms 1p/19q has been connected with a good response to treatment in low-grade gliomas (LGG) in several studies. For treatment planning, the ability to anticipate 1p19q status is crucial. This research's purpose is to develop a noninvasive approach based on MR images using our efficient CNNs. While public networks like VGGNet, GoogleNet, and other well-known public networks can use transfer learning to identify brain cancer on MRI, the model contains a large number of components that are unrelated to brain tumors. We build a model from the bottom-up, rather than relying on transfer learning. Our network structure flexibly uses a deep convolution stack mixed with dropout and dense operation, which reduces overfitting and enhances performance. We increase the number of samples by augmenting the dataset. The Gaussian noise is introduced during the model training. To address the issue of data imbalance, we use stratified k-fold cross-validation during training to find the best model. Our proposed model is compared with models fine-tuned through transfer learning, such as MobileNetV2, InceptionResNetV2, and VGG16. Our model achieves better results than these models on the same small dataset. In the test set, when deciding whether or not an image should be 1p/19q codeleted, the proposed architecture achieved an F1-score of 96.50%, precision of 96.50%, recall of 96.49%, and accuracy of 96.50%. By comparing with the transfer model, we found that transfer learning does not outperform CNN on a small dataset.
更多查看译文
关键词
radiogenomic classification,gliomas,mri,efficient cnn,low-grade
AI 理解论文
溯源树
样例
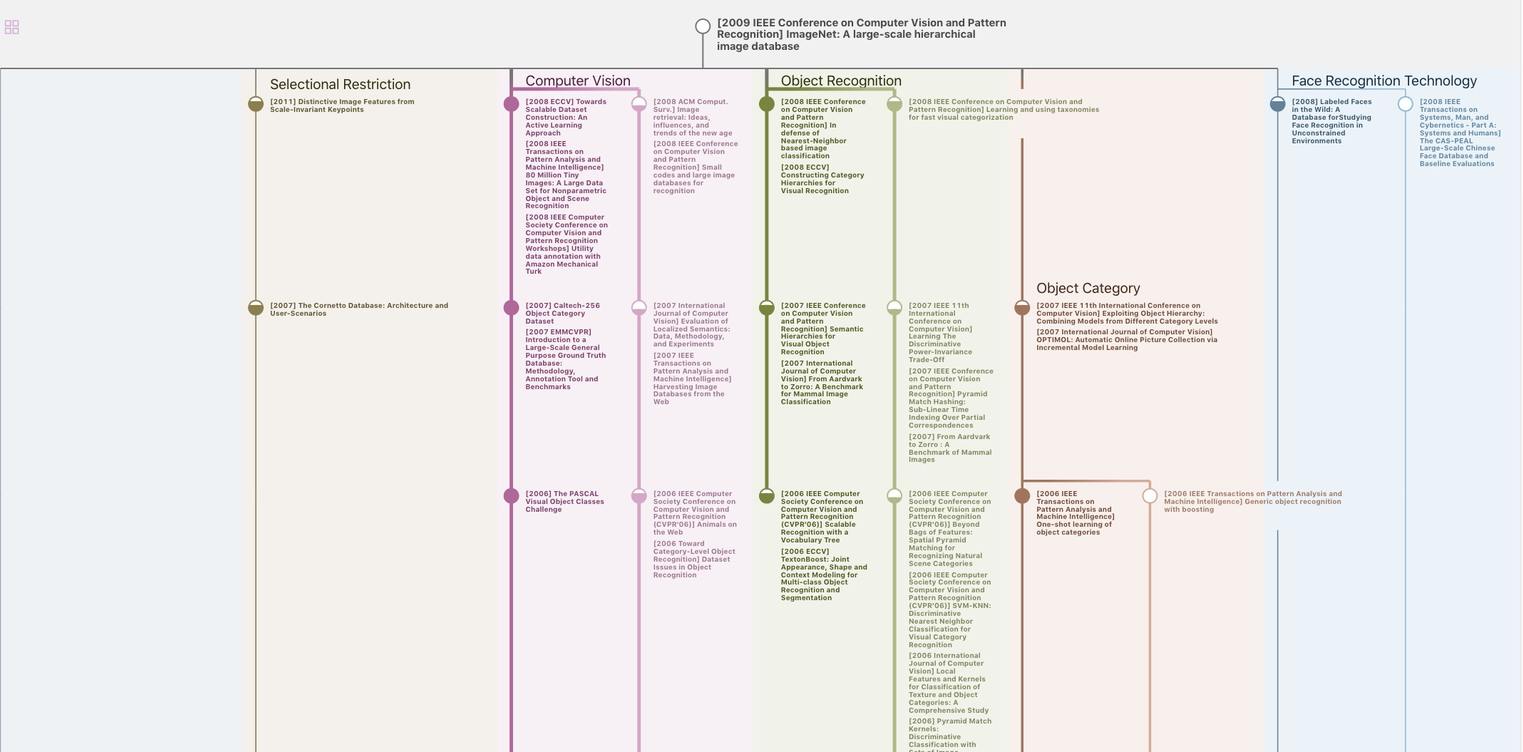
生成溯源树,研究论文发展脉络
Chat Paper
正在生成论文摘要