Weakly Supervised Object Detection With Class Prototypical Network
IEEE Transactions on Multimedia(2023)
摘要
In this paper, we aim to devise a new framework to compel the network to be equipped with the capability of detecting objects using image-level class labels as supervision. The challenge of such a weakly supervised setting mainly lies in how to make the network accurately understand both semantics and objectness of a given proposal without bounding box annotations. To this end, we contribute a concise framework, named Class Prototypical Network (CPNet). Concretely, our CPNet defines a set of learnable class prototypes to help classify object proposals. To endow the prototypes be not only discriminative for classes but also sensitive for proposals' objectness, we conduct both class-aware cross-attention and location-aware cross-attention between the feature embeddings of the learnable prototypes and the proposals. The learned attention scores are then used to form the proposal-level category information into the image-level one, making the entire framework be trained without any bounding box annotations. Besides, by applying these two kinds of attention mechanisms, the knowledge from both proposals' location and its class information can be successfully transferred into the corresponding prototypes. With the help of prototypes, our CPNet detects true positive object proposals. In addition, the CPNet further introduces a multi-head detection head to perform complementary training, preventing the model from falling into local discriminative parts and improving the model's performance on challenging non-rigid categories. We examine our CPNet on popular benchmarks, i.e., PASCAL VOC 2007, 2012 and MS COCO 2014. Extensive experiments show our CPNet is a simple and effective framework.
更多查看译文
关键词
Cross-attention,prototypical network,weakly supervised object detection
AI 理解论文
溯源树
样例
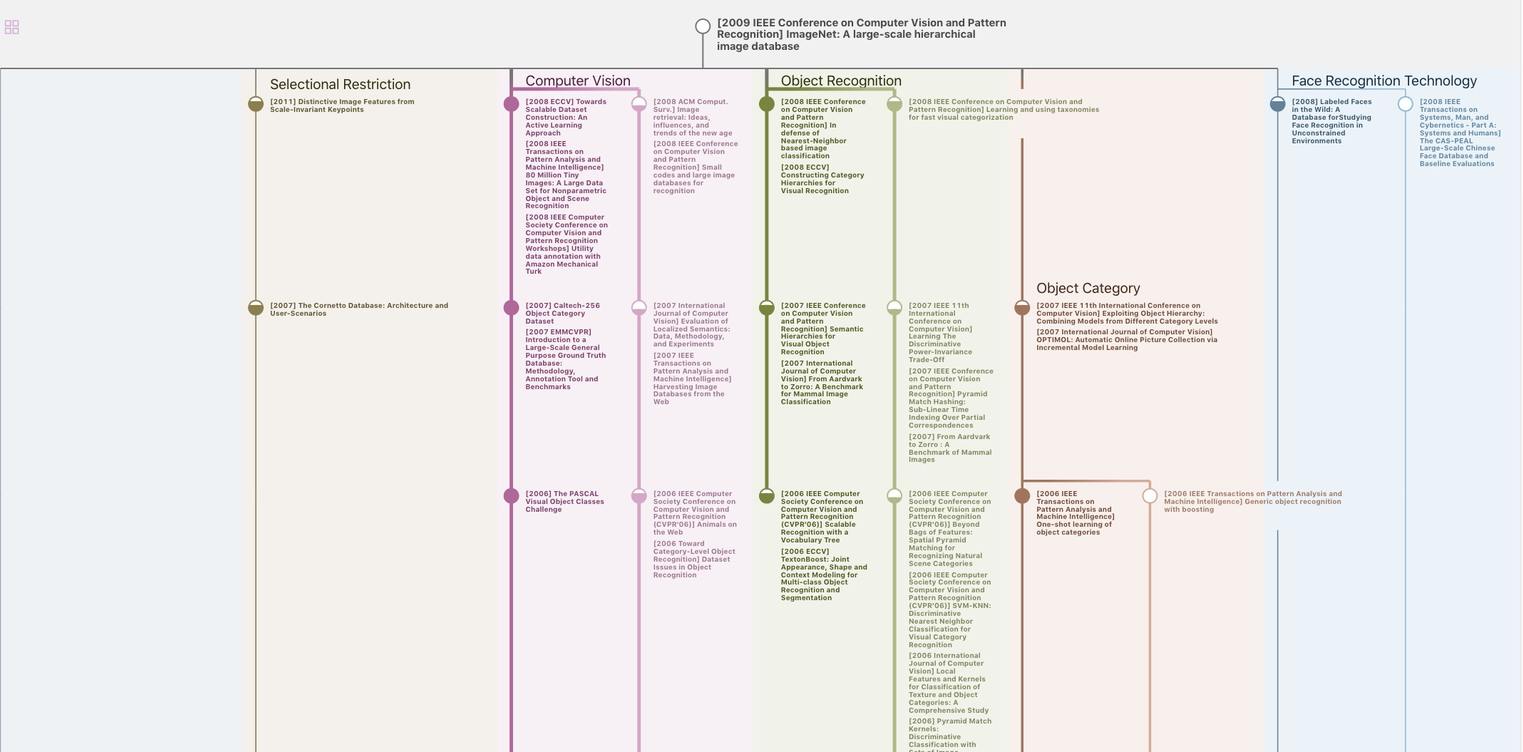
生成溯源树,研究论文发展脉络
Chat Paper
正在生成论文摘要