Training Large-Vocabulary Neural Language Models by Private Federated Learning for Resource-Constrained Devices
arxiv(2022)
摘要
Federated Learning (FL) is a technique to train models using data distributed across devices. Differential Privacy (DP) provides a formal privacy guarantee for sensitive data. Our goal is to train a large neural network language model (NNLM) on compute-constrained devices while preserving privacy using FL and DP. However, the DP-noise introduced to the model increases as the model size grows, which often prevents convergence. We propose Partial Embedding Updates (PEU), a novel technique to decrease noise by decreasing payload size. Furthermore, we adopt Low Rank Adaptation (LoRA) and Noise Contrastive Estimation (NCE) to reduce the memory demands of large models on compute-constrained devices. This combination of techniques makes it possible to train large-vocabulary language models while preserving accuracy and privacy.
更多查看译文
关键词
private federated
AI 理解论文
溯源树
样例
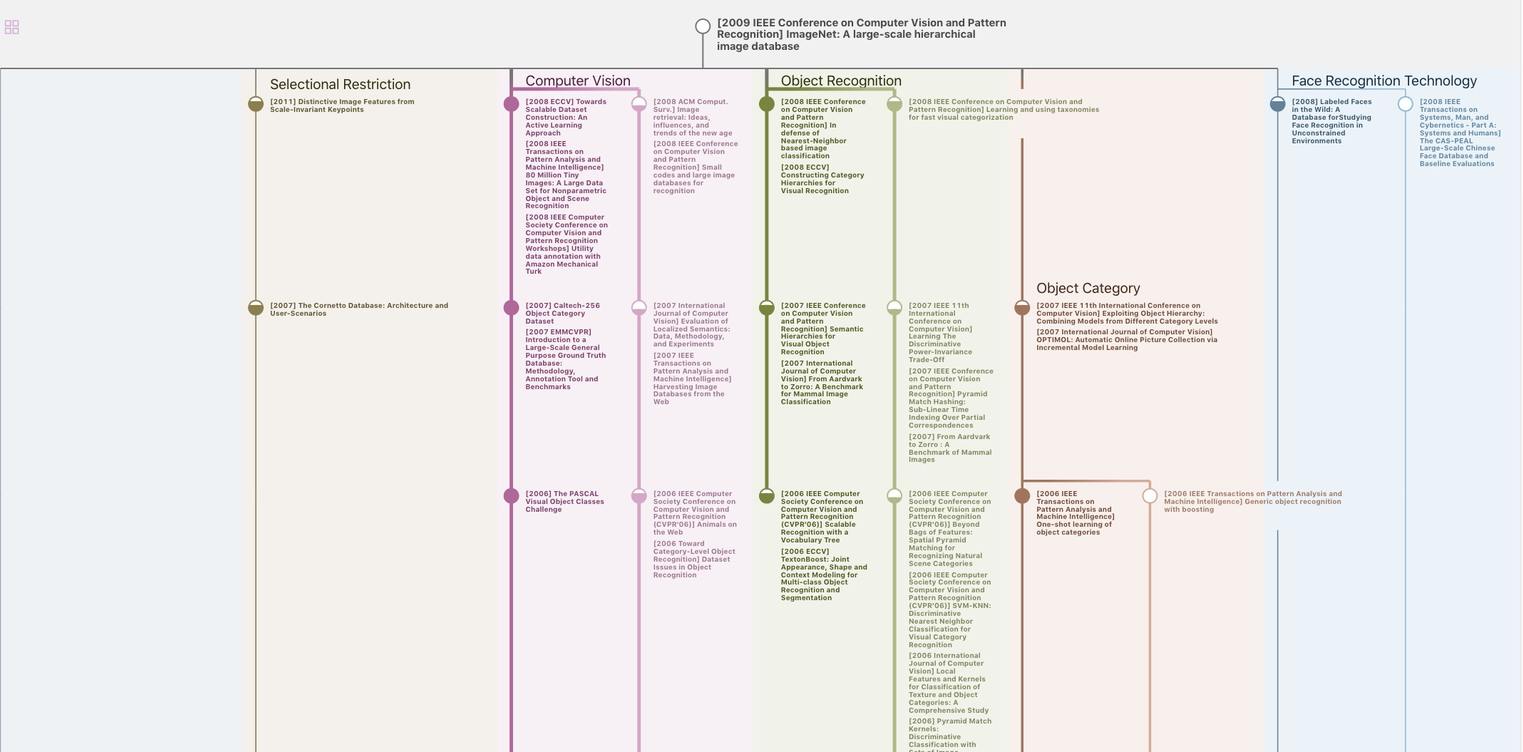
生成溯源树,研究论文发展脉络
Chat Paper
正在生成论文摘要