Mutually reinforced network embedding: An integrated approach to research paper recommendation
Expert Systems with Applications(2022)
摘要
With the rapid growth of research publications, it has become time-consuming and cumbersome for researchers to find research papers relevant to their research. Research paper recommendation, which provides a reference list for a given manuscript, providing convenience for researchers. In the past, researchers embedded papers into a low-dimensional vector space through textual information and network structure to obtain paper embeddings, and then made recommendations based on the paper embeddings. This paper argues that in addition to the network structure and textual information, the co-authorship of a paper should also be considered, as it reveals the author’s interaction preference in the papers involved and reflects the topic of the paper from the author’s perspective. In this paper, we consider network structure, textual information and co-authorship of a paper simultaneously, and develop a mutual reinforcement network embedding (MRNE) model to enhance network embedding via mutual reinforcement between co-authorship and textual information based on network structure. Based on this model, we propose a cold-start research paper recommendation approach, which is evaluated on the link prediction-based paper recommendation and cold-start-based paper recommendation, respectively. Experimental results on HepTh and AAN datasets demonstrate effectiveness of the proposed method. This paper provides a new idea for the study of the cold start problem in research paper recommendation.
更多查看译文
关键词
Research paper recommendation,Network embedding,Mutually reinforced model
AI 理解论文
溯源树
样例
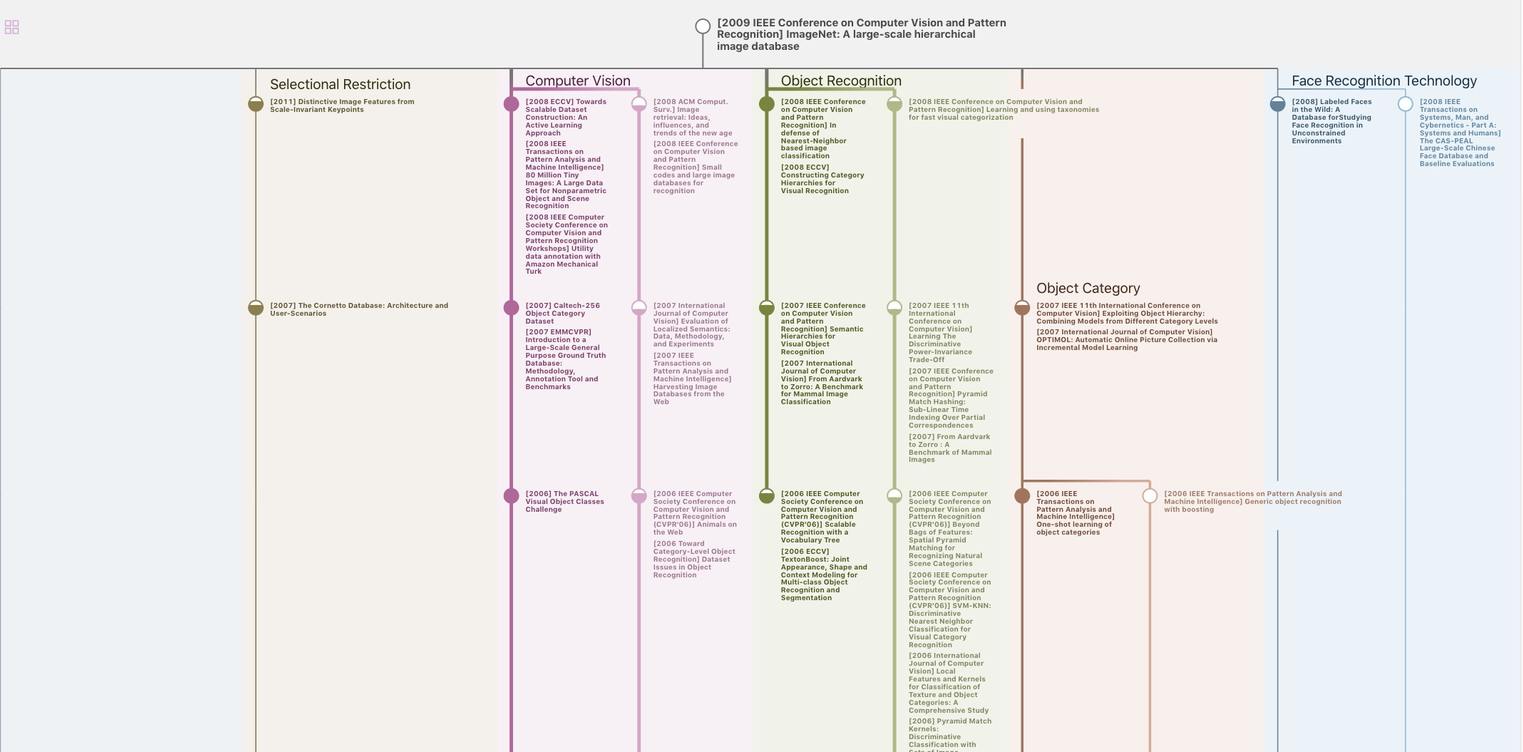
生成溯源树,研究论文发展脉络
Chat Paper
正在生成论文摘要