Unsupervised Feature Selection with Flexible Optimal Graph
IEEE TRANSACTIONS ON NEURAL NETWORKS AND LEARNING SYSTEMS(2024)
摘要
In the unsupervised feature selection method based on spectral analysis, constructing a similarity matrix is a very important part. In existing methods, the linear low-dimensional projection used in the process of constructing the similarity matrix is too hard, it is very challenging to construct a reliable similarity matrix. To this end, we propose a method to construct a flexible optimal graph. Based on this, we propose an unsupervised feature selection method named unsupervised feature selection with flexible optimal graph and l(2,1)-norm regularization (FOG-R). Unlike other methods that use linear projection to approximate the low-dimensional manifold of the original data when constructing a similarity matrix, FOG-R can learn a flexible optimal graph, and by combining flexible optimal graph learning and feature selection into a unified framework to get an adaptive similarity matrix. In addition, an iterative algorithm with a strict convergence proof is proposed to solve FOG-R. l(2,1)-norm regularization will introduce an additional regularization parameter, which will cause parameter-tuning trouble. Therefore, we propose another unsupervised feature selection method, that is, unsupervised feature selection with a flexible optimal graph and l(2,0)-norm constraint (FOG-C), which can avoid tuning additional parameters and obtain a more sparse projection matrix. Most critically, we propose an effective iterative algorithm that can solve FOG-C globally' with strict convergence proof. Comparative experiments conducted on 12 public datasets show that FOG-R and FOG-C perform better than the other nine state-of-the-art unsupervised feature selection algorithms.
更多查看译文
关键词
Flexible optimal graph,l(2,0)-norm constraint optimization,l(2,1)-norm regularization,unsupervised feature selection
AI 理解论文
溯源树
样例
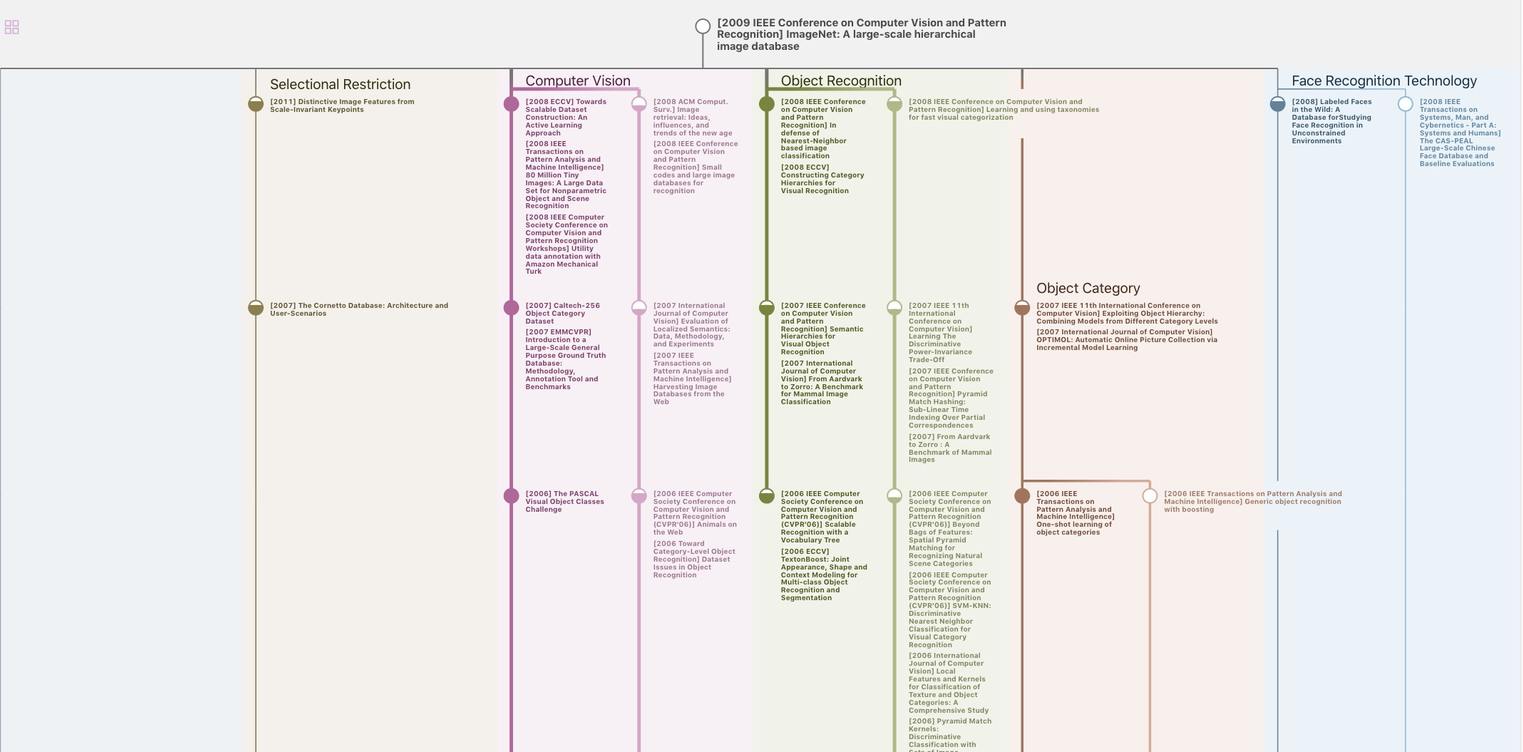
生成溯源树,研究论文发展脉络
Chat Paper
正在生成论文摘要