PB-GCN: Progressive binary graph convolutional networks for skeleton-based action recognition
Neurocomputing(2022)
摘要
Skeleton-based action recognition is an essential yet challenging visual task, whose accuracy has been remarkably improved due to the successful application of graph convolutional networks (GCNs). However, high computation cost and memory usage hinder their deployment on resource-constrained environment. To deal with the issue, in this paper, we introduce two novel progressive binary graph convolutional network for skeleton-based action recognition PB-GCN and PB-GCN*, which can obtain significant speed-up and memory saving. In PB-GCN, the filters are binarized, and in PB-GCN*, both filters and activations are binary. Specifically, we propose a progressive optimization, i.e., employing ternary models as the initialization of binary GCNs (BGCN) to improve the representational capability of binary models. Moreover, the center loss is exploited to improve the training procedure for better performance. Experimental results on two public benchmarks (i.e., Skeleton-Kinetics and NTU RGB + D) demonstrate that the accuracy of the proposed PB-GCN and PB-GCN* are comparable to their full-precision counterparts and outperforms the state-of-the-art methods, such as BWN, XNOR-Net, and Bi-Real Net.
更多查看译文
关键词
Binary neural network,Center loss,Progressive optimization,Skeleton-based action recognition,Spatial-temporal graph convolutional networks
AI 理解论文
溯源树
样例
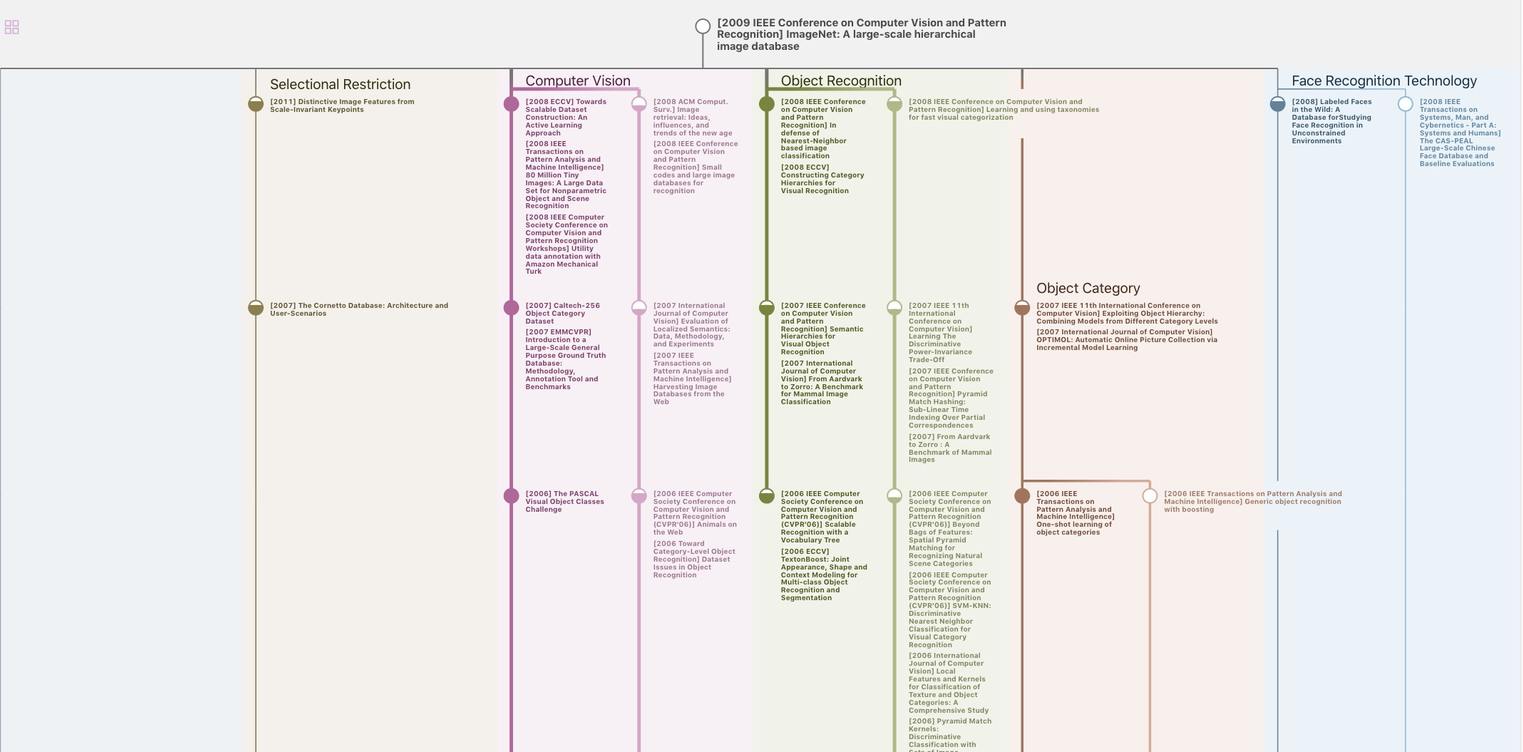
生成溯源树,研究论文发展脉络
Chat Paper
正在生成论文摘要