Aligning Dual Disentangled User Representations from Ratings and Textual Content
KDD '22: Proceedings of the 28th ACM SIGKDD Conference on Knowledge Discovery and Data Mining(2022)
摘要
Classical recommendation methods typically render user representation as a single vector in latent space. Oftentimes, a user's interactions with items are influenced by several hidden factors. To better uncover these hidden factors, we seek disentangled representations. Existing disentanglement methods for recommendations are mainly concerned with user-item interactions alone. To further improve not only the effectiveness of recommendations but also the interpretability of the representations, we propose to learn a second set of disentangled user representations from textual content and to align the two sets of representations with one another. The purpose of this coupling is two-fold. For one benefit, we leverage textual content to resolve sparsity of user-item interactions, leading to higher recommendation accuracy. For another benefit, by regularizing factors learned from user-item interactions with factors learned from textual content, we map uninterpretable dimensions from user representation into words. An attention-based alignment is introduced to align and enrich hidden factors representations. A series of experiments conducted on four real-world datasets show the efficacy of our methods in improving recommendation quality.
更多查看译文
关键词
dual disentangled user representations,ratings,content
AI 理解论文
溯源树
样例
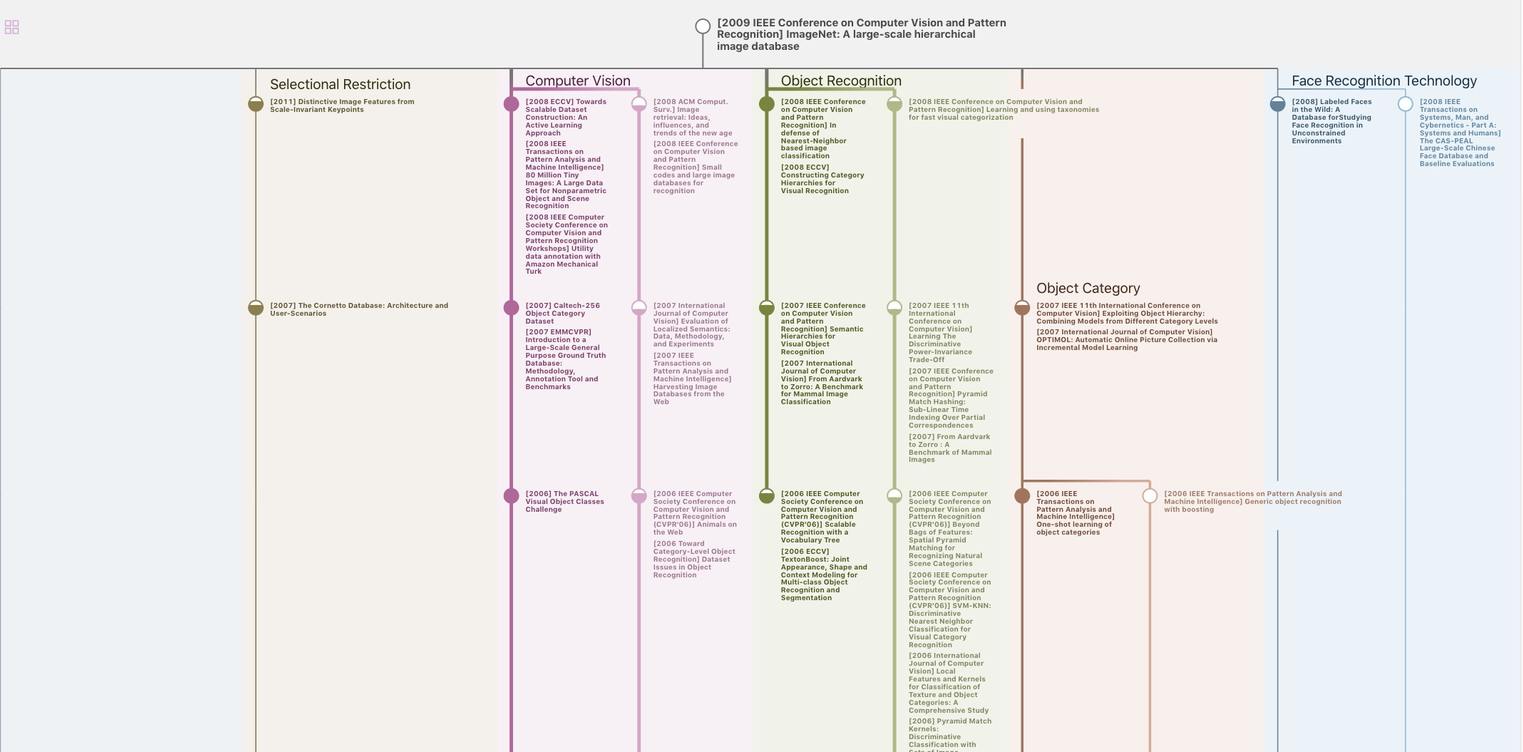
生成溯源树,研究论文发展脉络
Chat Paper
正在生成论文摘要