Neural Population Dynamics of Computing with Synaptic Modulations
eLife(2022)
摘要
In addition to long-time scale rewiring, synapses in the brain are subject to significant modulation that occurs at much shorter time scales and allows them to process short-term information. Despite this, models of the brain like recurrent neural networks (RNNs) often have their weights frozen after training, relying on an internal state stored in neuron activity to process temporal information. Although networks with dynamical synapses have been explored previously, often said dynamics are added to networks that also have recurrent connections and thus the short-time scale computational capabilities of synapse modulation alone remain unclear. In this work, we analyze the dynamics of a network that relies solely on synaptic modulations to process short-time scale information, the multi-plasticity network (MPN). We thoroughly examine the neural population dynamics of the MPN trained on integration-based tasks and compare it to known RNN dynamics, findings the two to have fundamentally different behavior and attractor structure. We find said differences in dynamics allow the MPN to outperform its RNN counterparts on several neuroscience-relevant tasks. Of note, the MPN has a significantly simpler attractor structure that allows it to be more flexible in training and sequential-learning settings. Lastly, how the dynamics change for MPNs trained on contextual and continuous integration tasks is also investigated.
### Competing Interest Statement
The authors have declared no competing interest.
更多查看译文
AI 理解论文
溯源树
样例
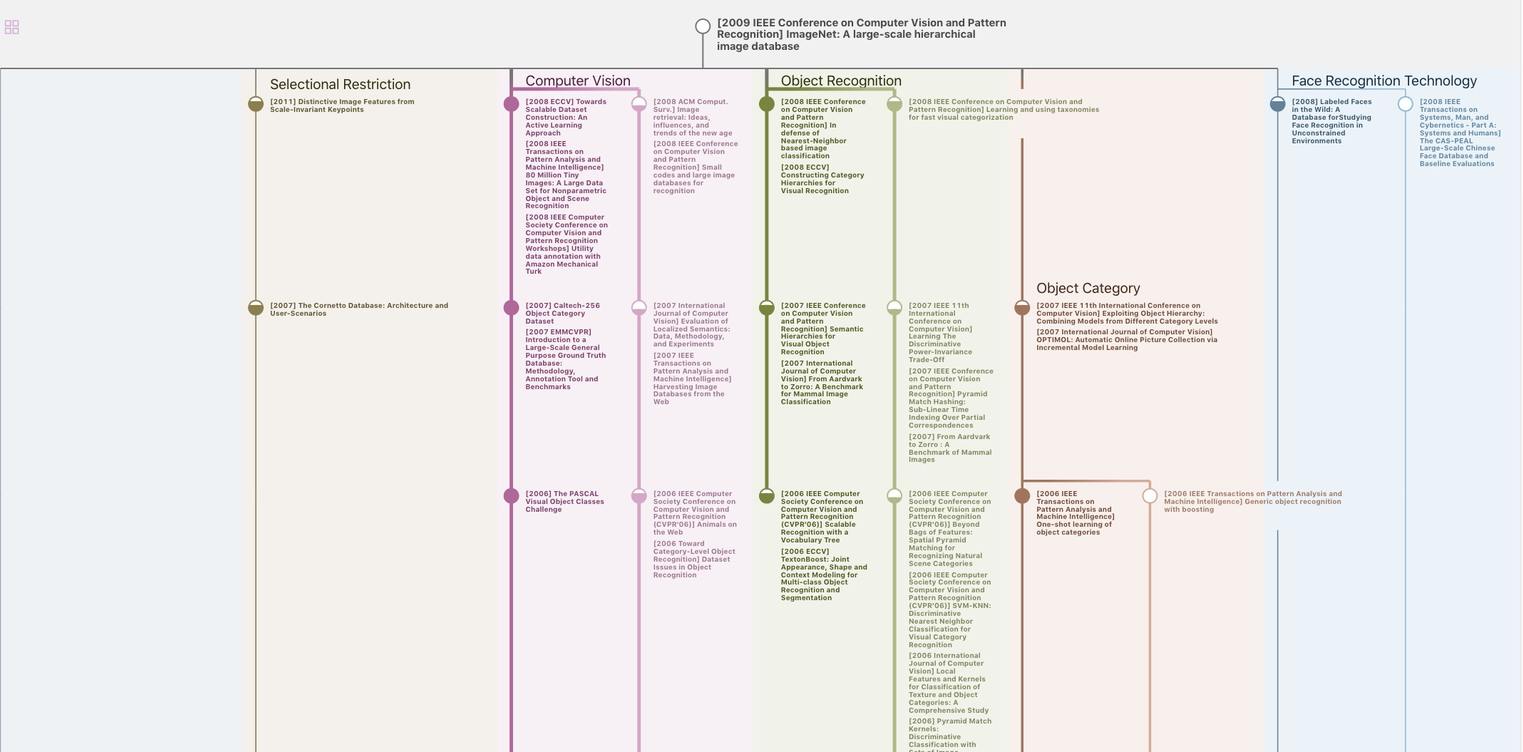
生成溯源树,研究论文发展脉络
Chat Paper
正在生成论文摘要